Walking on the Image Trail: Uncovering the Past and Predicting the Future
2017 IEEE Third International Conference on Multimedia Big Data (BigMM)(2017)
摘要
Predicting the temporal evolution of images is an interesting problem that has applications in surveillance, content recommendation and behavioral analysis. Given a single image or a stream of images with timestamps, the goal of this work is to predict possible images that could appear at different time instances in the future. We propose a data-driven Riemannian shape theoretic approach for this problem, which analyzes the space of temporal evolution patterns in training image streams, and performs statistics on this shape space to facilitate future image prediction. We consider both discriminative and generative statistical techniques on the shape space, to accommodate cases where the training and test data may or may not have an associated class/topic label, and report improved prediction results on two previously studied image prediction datasets. We also provide complimentary results on predicting images in the past, for time instances before the training data was acquired, and empirically analyze how the prediction accuracy varies over time.
更多查看译文
关键词
image trail,temporal evolution prediction,data-driven Riemannian shape theoretic approach,temporal evolution patterns,image streams,shape space,discriminative statistical techniques,generative statistical techniques,image prediction datasets,time instances,prediction accuracy
AI 理解论文
溯源树
样例
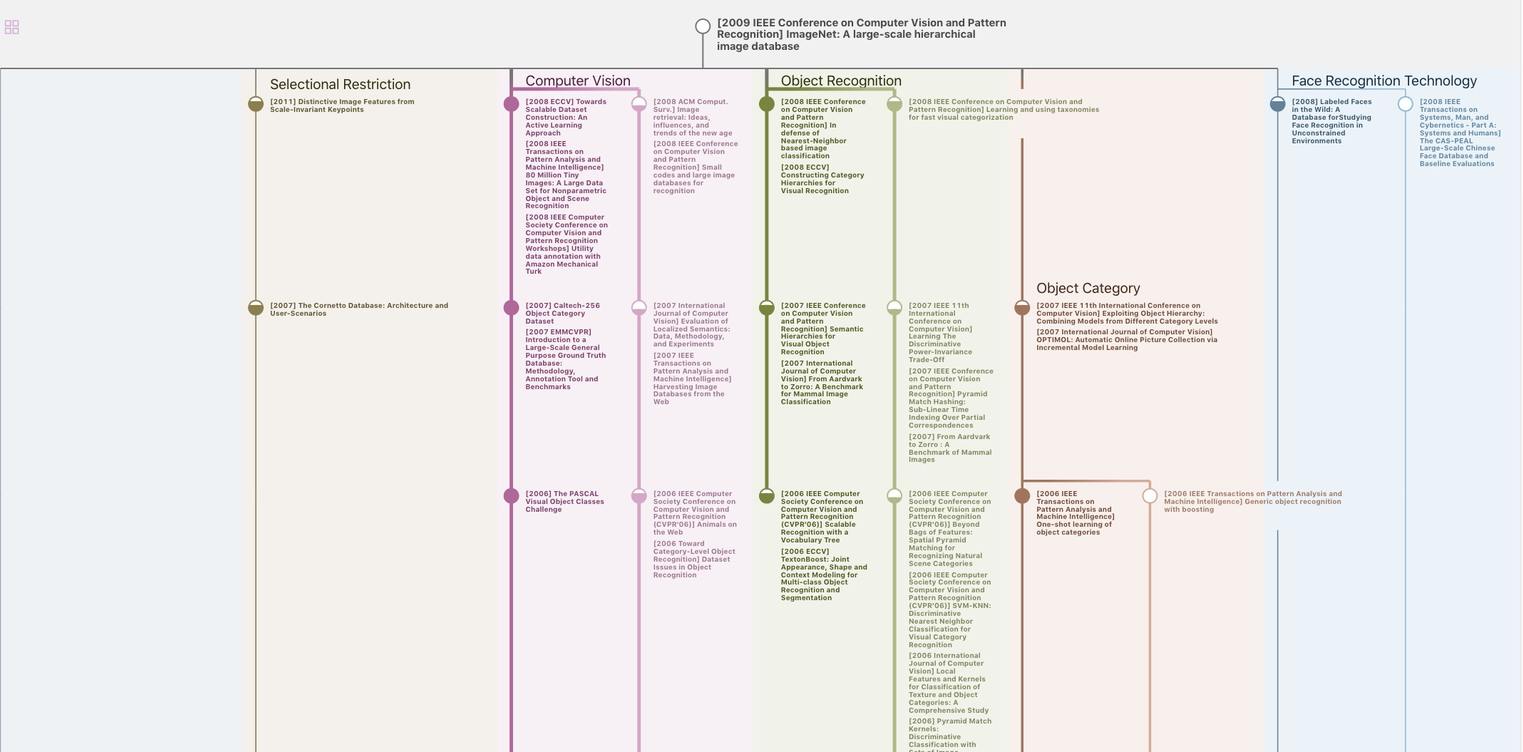
生成溯源树,研究论文发展脉络
Chat Paper
正在生成论文摘要