Temporal Archetypal Analysis for Action Segmentation
2017 12th IEEE International Conference on Automatic Face & Gesture Recognition (FG 2017)(2017)
摘要
Unsupervised learning of invariant representations that efficiently describe high-dimensional time series has several applications in dynamic visual data analysis. Clearly, the problem becomes more challenging when dealing with multiple time series arising from different modalities. A prominent example of this multimodal setting is the human motion which can be represented by multimodal time series of pixel intensities, depth maps, and motion capture data. Here, we study, for the first time, the problem of unsupervised learning of temporally and modality invariant informative representations, referred to as archetypes, from multiple time series originating from different modalities. To this end a novel method, coined as temporal archetypal analysis, is proposed. The performance of the proposed method is assessed by conducting experiments in unsupervised action segmentation. Experimental results on three different real world datasets using single modal and multimodal visual representations indicate the robustness and effectiveness of the proposed methods, outperforming compared state-of-the-art methods by a large, in most of the cases, margin.
更多查看译文
关键词
temporal archetypal analysis,action segmentation,unsupervised learning,invariant representations,high-dimensional time series,dynamic visual data analysis,human motion representation,multimodal time series,pixel intensities,depth maps,motion capture data,temporally invariant informative representations,modality invariant informative representations,multimodal visual representations,single modal visual representations
AI 理解论文
溯源树
样例
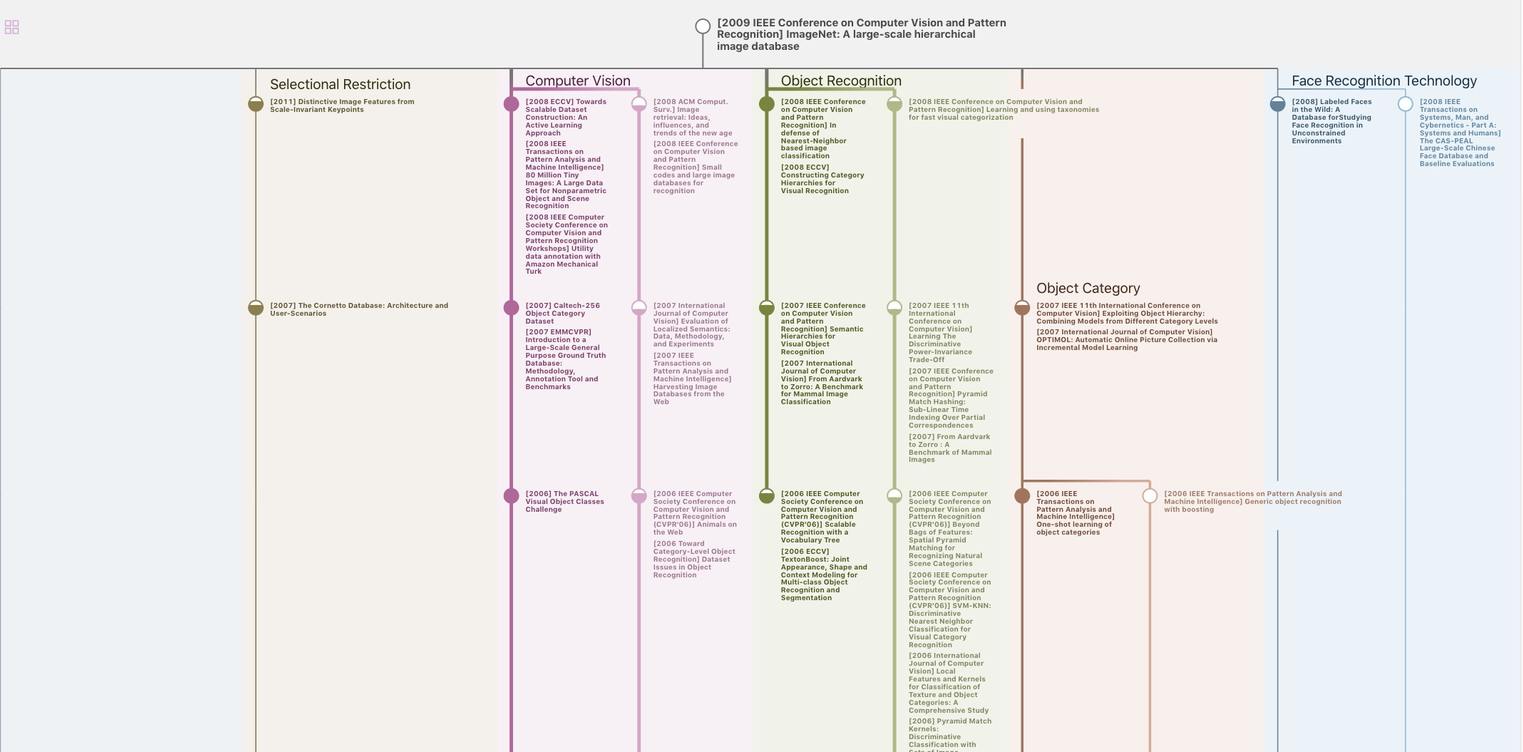
生成溯源树,研究论文发展脉络
Chat Paper
正在生成论文摘要