Learning Process Behavioral Baselines for Anomaly Detection
2017 IEEE 22nd Pacific Rim International Symposium on Dependable Computing (PRDC)(2017)
摘要
Intrusion resilience is a protection strategy aimed at building systems that can continue to provide service during attacks. One approach to intrusion resilience is to continuously monitor a system's state and change its configuration to maintain service even while attacks are occurring. Intrusion detection, through both anomaly detection (for unknown attacks) and signature detection (for known attacks) is thus a crucial part of that resilience strategy. In this paper, we introduce KOBRA, an online anomaly detection engine that learns behavioral baselines for applications. KOBRA is implemented as a set of cooperative kernel modules that collects time-stamped process events. The process events are converted to a discrete-time signal in the polar space. We learn local patterns that occur in the data and then learn the normal co-occurrence relationships between the patterns. The patterns and the co-occurrence relations model the normal behavioral baseline of an application. We compute an anomaly score for tested traces and compare it against a threshold for anomaly detection. We evaluate the baseline by experimenting with its ability to discriminate between different processes and detect malicious behavior.
更多查看译文
关键词
anomaly detection,behavioral baseline,intrusion detection system,intrusion resilience,kernel monitoring
AI 理解论文
溯源树
样例
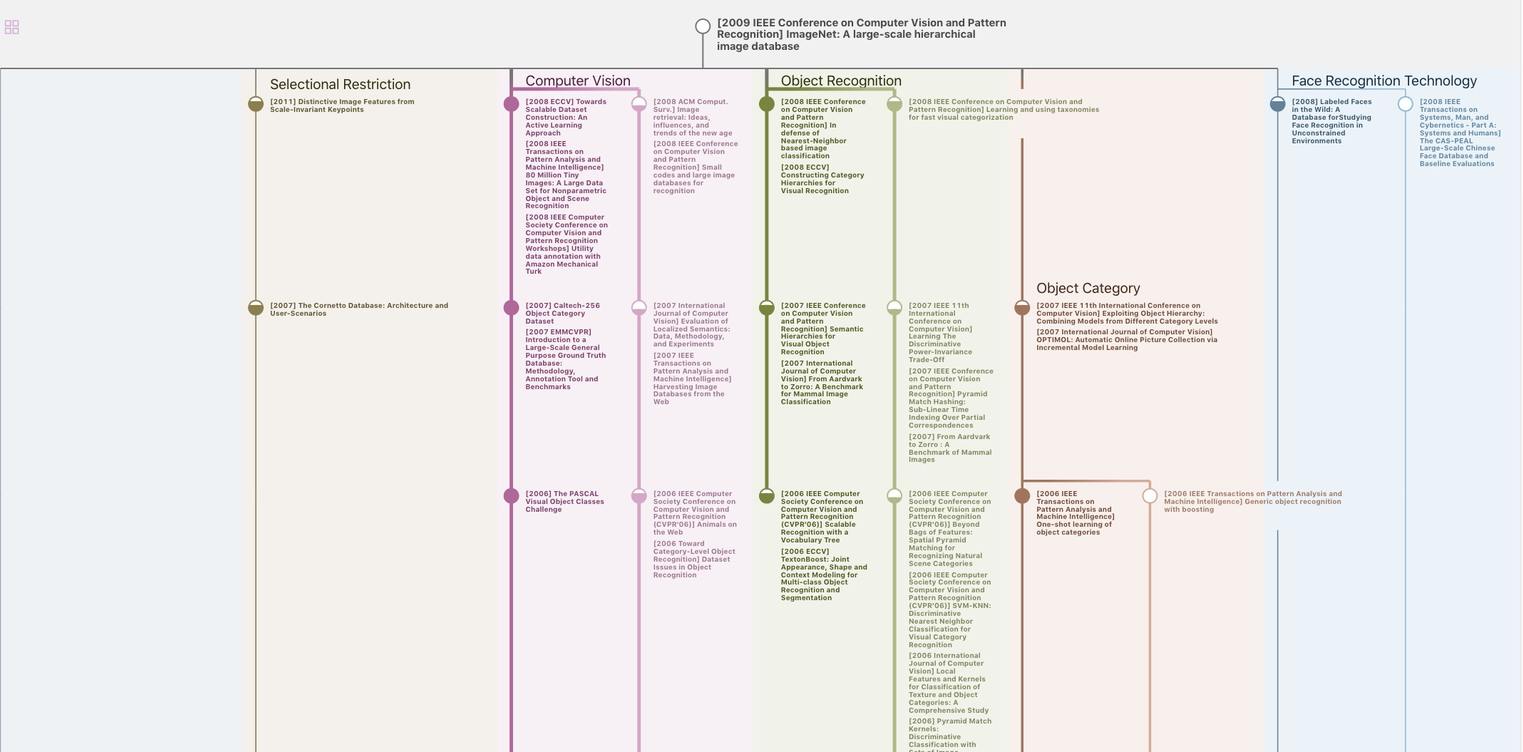
生成溯源树,研究论文发展脉络
Chat Paper
正在生成论文摘要