Estimating the unseen from multiple populations
ICML(2017)
摘要
Given samples from a distribution, how many new elements should we expect to find if we continue sampling this distribution? This is an important and actively studied problem, with many applications ranging from unseen species estimation to genomics. We generalize this extrapolation and related unseen estimation problems to the multiple population setting, where population j has an unknown distribution D_j from which we observe n_j samples. We derive an optimal estimator for the total number of elements we expect to find among new samples across the populations. Surprisingly, we prove that our estimator's accuracy is independent of the number of populations. We also develop an efficient optimization algorithm to solve the more general problem of estimating multi-population frequency distributions. We validate our methods and theory through extensive experiments. Finally, on a real dataset of human genomes across multiple ancestries, we demonstrate how our approach for unseen estimation can enable cohort designs that can discover interesting mutations with greater efficiency.
更多查看译文
关键词
multiple populations
AI 理解论文
溯源树
样例
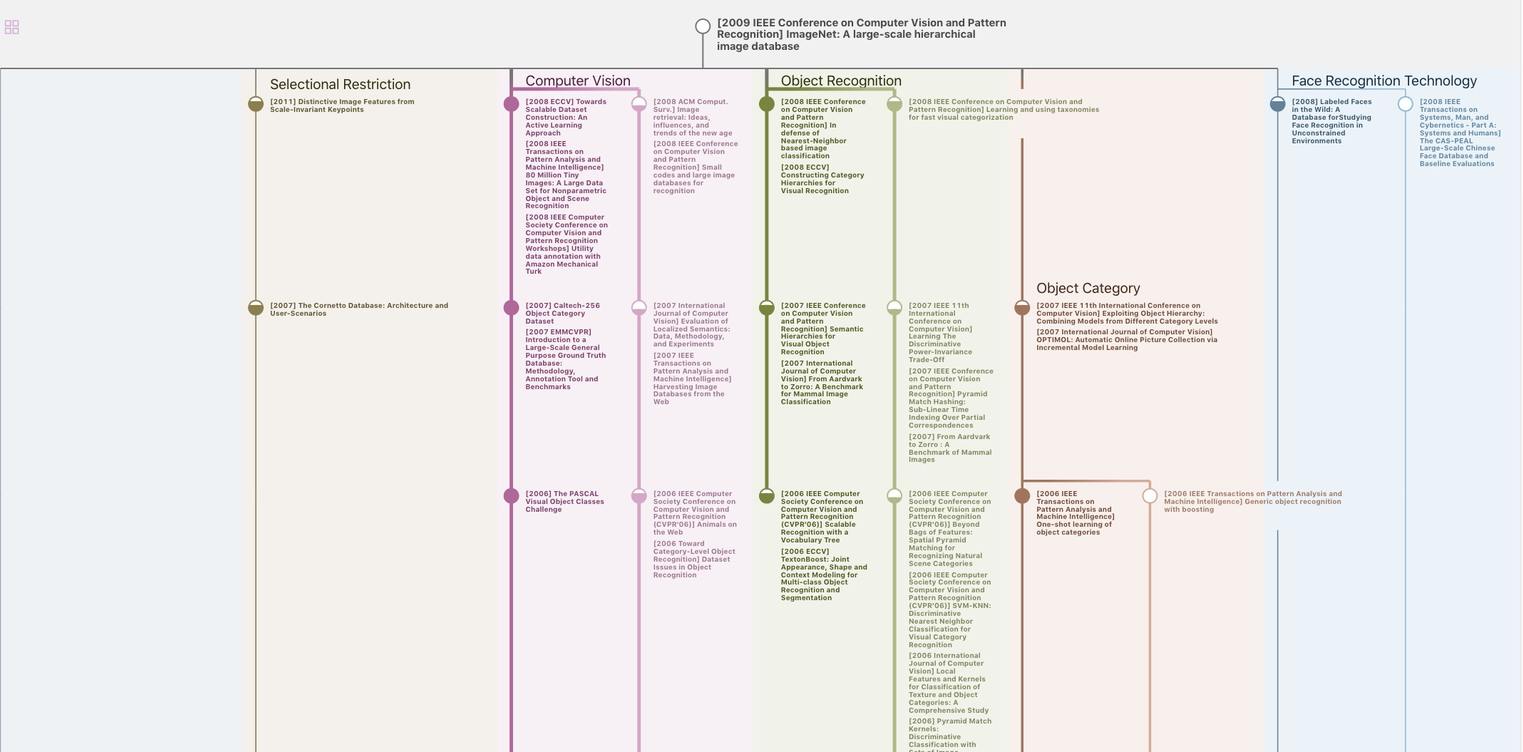
生成溯源树,研究论文发展脉络
Chat Paper
正在生成论文摘要