Short-Term Traffic Prediction Under Both Typical And Atypical Traffic Conditions Using A Pattern Transition Model
VEHITS: PROCEEDINGS OF THE 3RD INTERNATIONAL CONFERENCE ON VEHICLE TECHNOLOGY AND INTELLIGENT TRANSPORT SYSTEMS(2017)
摘要
One of the most challenging goals of the modern Intelligent Transportation Systems comprises the accurate and real-time short-term traffic prediction. The achievement of this goal becomes even more critical when the presence of atypical traffic conditions is concerned. In this paper, we propose a novel hybrid technique for short-term traffic prediction under both typical and atypical conditions. An Automatic Incident Detection (AID) algorithm, based on Support Vector Machines (SVM), is utilized to check for the presence of an atypical event (e.g. traffic accident). If such an event occurs, the k-Nearest Neighbors (k-NN) non-parametric regression model is used for traffic prediction. Otherwise, the Autoregressive Integrated Moving Average (ARIMA) parametric model is activated for the same purpose. In order to evaluate the performance of the proposed model, we use open real world traffic data from Caltrans Performance Measurement System (PeMS). We compare the proposed model with the unitary k-NN and ARIMA models, which represent the most commonly used non-parametric and parametric traffic prediction models. Preliminary results show that the proposed model achieves larger accuracy under both typical and atypical traffic conditions.
更多查看译文
关键词
Short-Term Traffic Prediction,Atypical Conditions,Automatic Incident Detection,Support Vector Machines,K-Nearest Neighbour,Autoregressive Integrated Moving Average
AI 理解论文
溯源树
样例
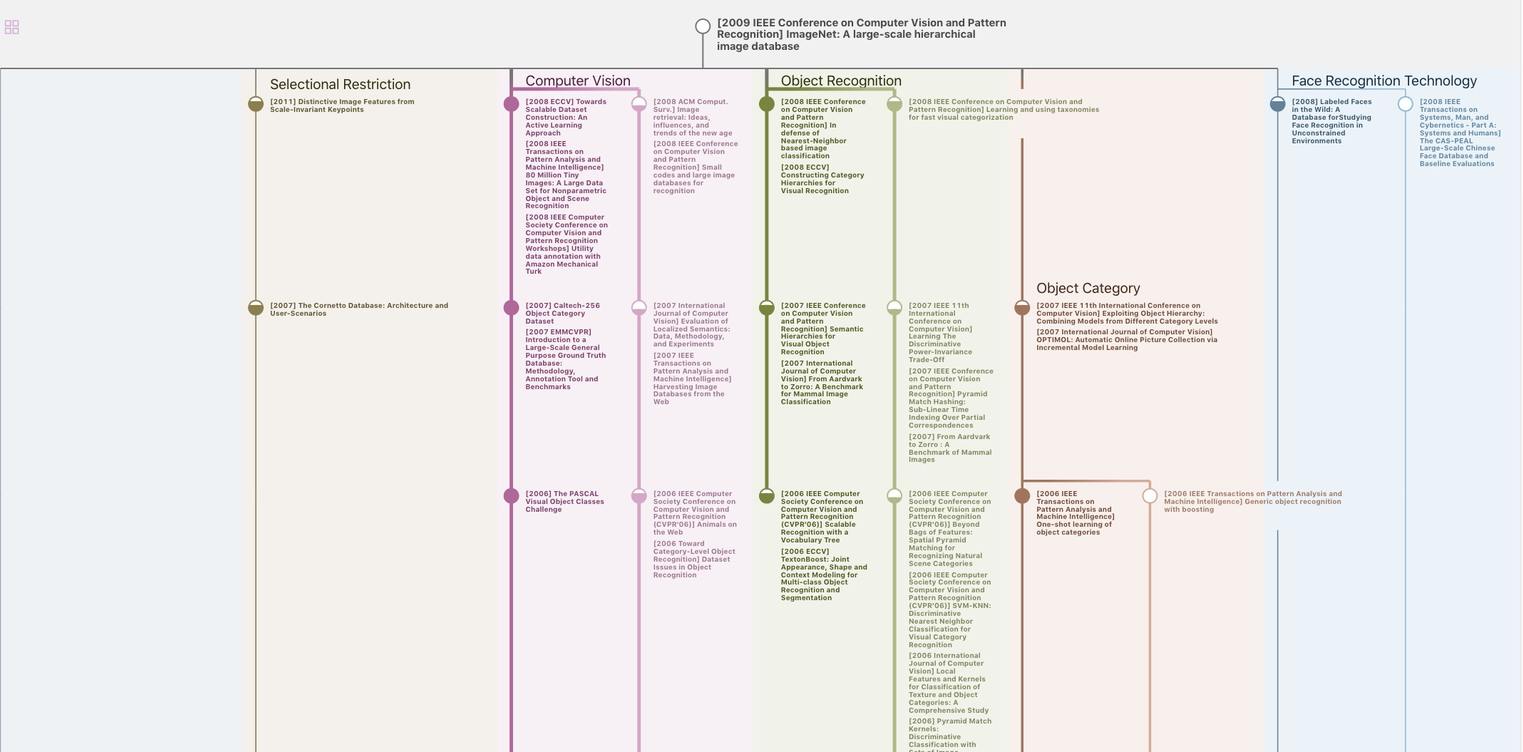
生成溯源树,研究论文发展脉络
Chat Paper
正在生成论文摘要