Robust Evaluation Matrix: Towards a More Principled Offline Exploration of Instructional Policies.
L@S(2017)
摘要
The gold standard for identifying more effective pedagogical approaches is to perform an experiment. Unfortunately, frequently a hypothesized alternate way of teaching does not yield an improved effect. Given the expense and logistics of each experiment, and the enormous space of potential ways to improve teaching, it would be highly preferable if it were possible to estimate in advance of running a study whether an alternative teaching strategy would improve learning. This is true even in learning at scale situations, since even if it is logistically easier to recruit a large number of subjects, it remains a high stakes environment because the experiment is impacting many real students. For certain classes of alternate teaching approaches, such as new ways to sequence existing material, it is possible to build student models that can be used as simulators to estimate the performance of learners under new proposed teaching methods. However, existing methods for doing so can overestimate the performance of new teaching methods. We instead propose the Robust Evaluation Matrix (REM) method which explicitly considers model mismatch between the student model used to derive the teaching strategy and that used as a simulator to evaluate the teaching strategy effectiveness. We then present two case studies from a fractions intelligent tutoring system and from a concept learning task from prior work that show how REM could be used both to detect when a new instructional policy may not be effective on actual students and to detect when it may be effective in improving student learning.
更多查看译文
关键词
instructional policies, reinforcement learning, off-policy, policy estimation, policy selection
AI 理解论文
溯源树
样例
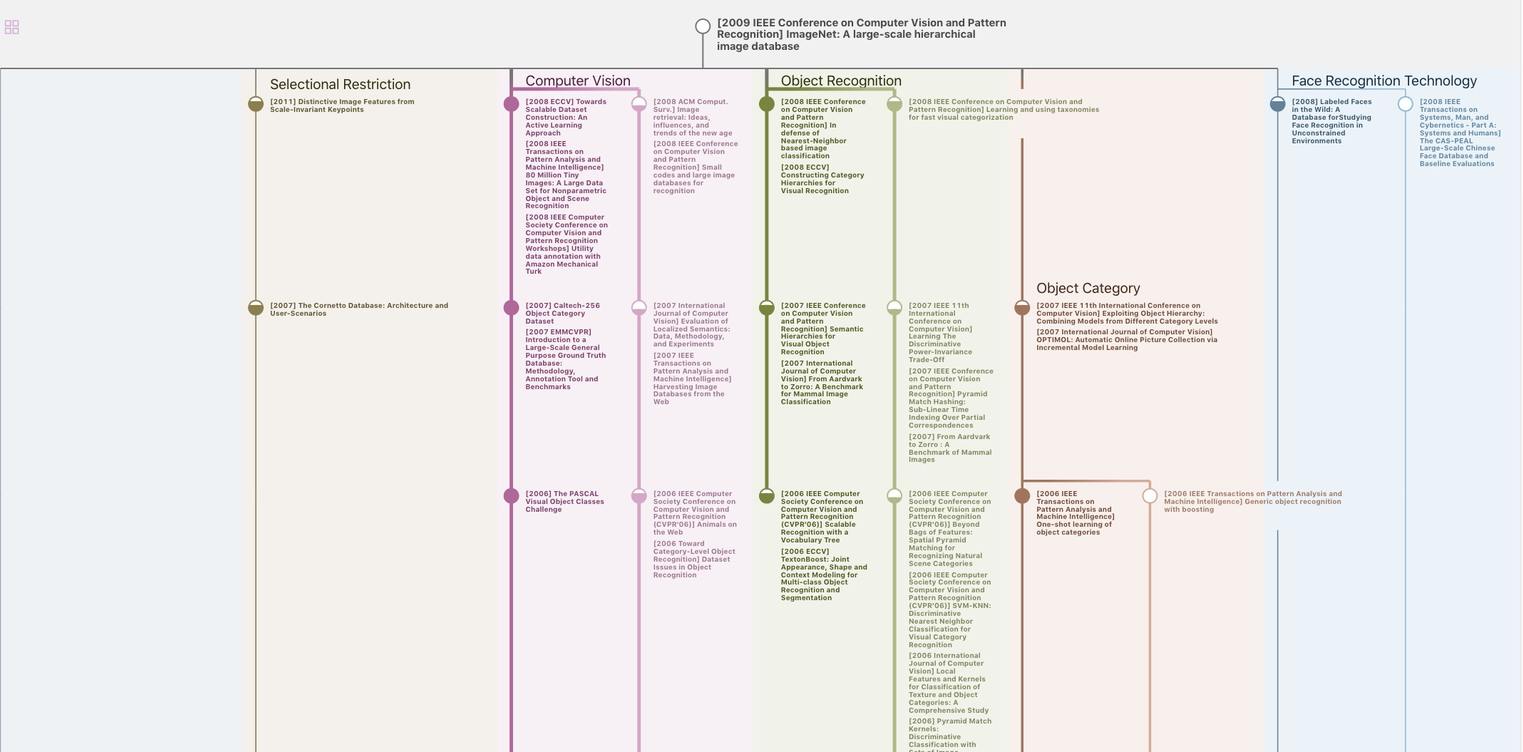
生成溯源树,研究论文发展脉络
Chat Paper
正在生成论文摘要