Efficient Model Averaging For Deep Neural Networks
COMPUTER VISION - ACCV 2016, PT II(2016)
摘要
Large neural networks trained on small datasets are increasingly prone to overfitting. Traditional machine learning methods can reduce overfitting by employing bagging or boosting to train several diverse models. For large neural networks, however, this is prohibitively expensive. To address this issue, we propose a method to leverage the benefits of ensembles without explicitely training several expensive neural network models. In contrast to Dropout, to encourage diversity of our sub-networks, we propose to maximize diversity of individual networks with a loss function: DivLoss. We demonstrate the effectiveness of DivLoss on the challenging CIFAR datasets.
更多查看译文
关键词
Hide Layer, Loss Function, Hide Unit, Ensemble Method, Individual Classifier
AI 理解论文
溯源树
样例
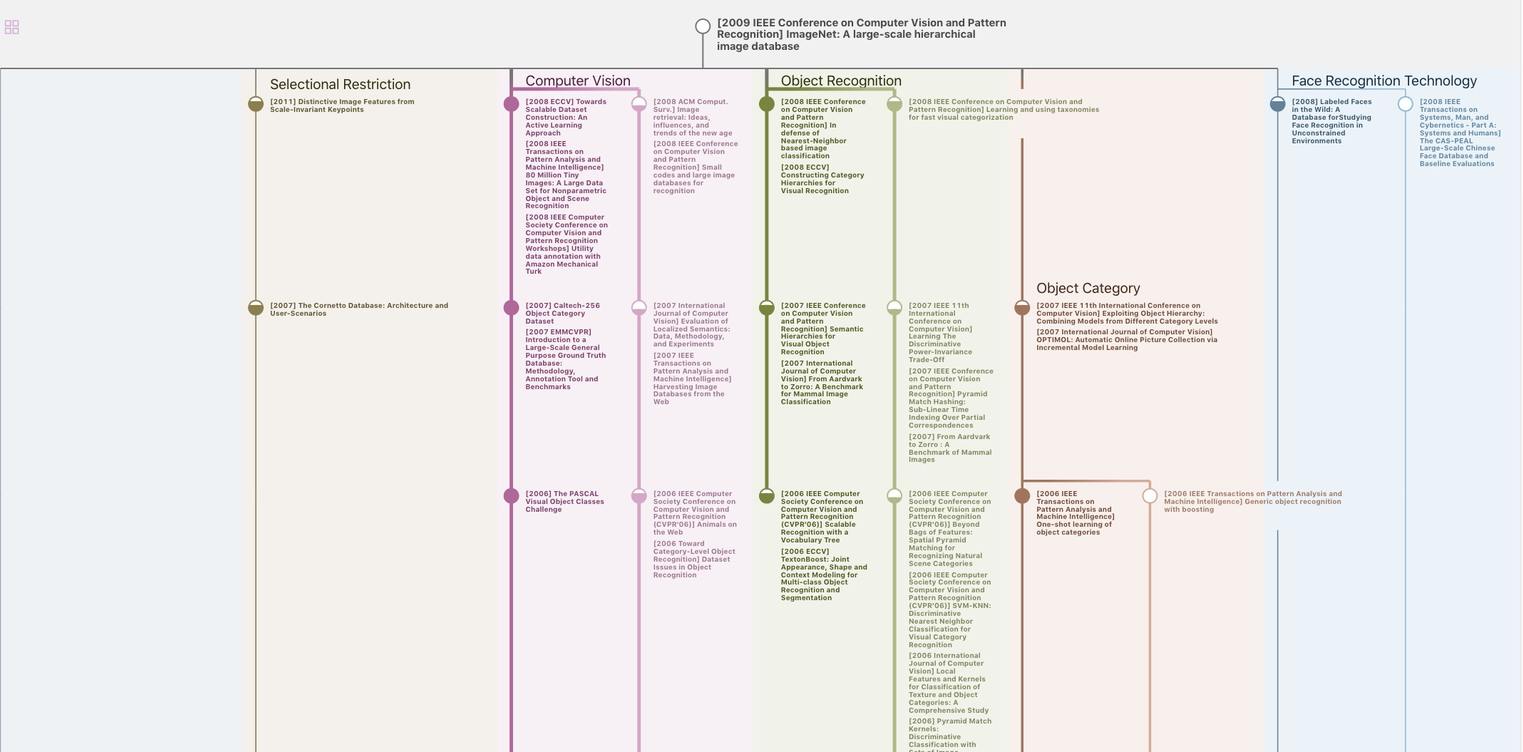
生成溯源树,研究论文发展脉络
Chat Paper
正在生成论文摘要