Robust Multi-Model Fitting Using Density And Preference Analysis
COMPUTER VISION - ACCV 2016, PT IV(2016)
摘要
Robust multi-model fitting problems are often solved using consensus based or preference based methods, each of which captures largely independent information from the data. However, most existing techniques still adhere to either of these approaches. In this paper, we bring these two paradigms together and present a novel robust method for discovering multiple structures from noisy, outlier corrupted data. Our method adopts a random sampling based hypothesis generation and works on the premise that inliers are densely packed around the structure, while the outliers are sparsely spread out. We leverage consensus maximization by defining the residual density, which is a simple and efficient measure of density in the 1-D residual space. We locate the inlier-outlier boundary by using preference based point correlations together with the disparity in residual density of inliers and outliers. Finally, we employ a simple strategy that uses preference based hypothesis correlation and residual density to identify one hypothesis representing each structure and their corresponding inliers. The strength of the proposed approach is evaluated empirically by comparing with state-ofthe- art techniques over synthetic data and the AdelaideRMF dataset.
更多查看译文
AI 理解论文
溯源树
样例
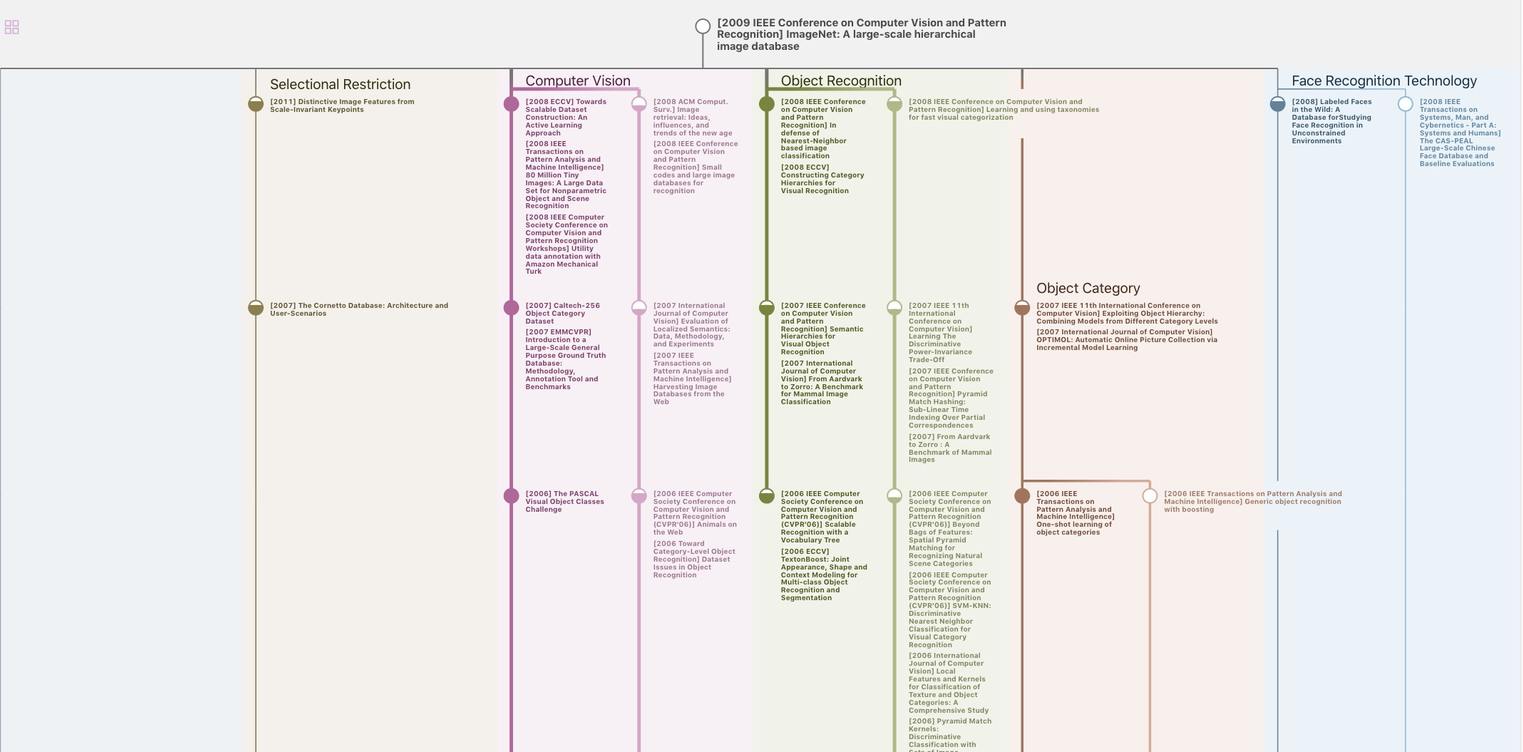
生成溯源树,研究论文发展脉络
Chat Paper
正在生成论文摘要