Low-Rank Tensor Recovery And Alignment Based On L(P) Minimization
ACCV Workshops(2017)
摘要
In this paper, we propose a framework of non-convex low-rank recovery and alignment for arbitrary tensor data. Specially, by using Schatten-p (0 < p < 1, the same below) norm and l(p) norm to relax the rank function and l(0) norm respectively, the model requires much weaker incoherence conditions to guarantee a successful recovery than the common used nuclear norm and l(1) norm. At the same time, we adopt a set of transformations which acts on the images of the tensor data to compensate the possible misalignments of images. By solving the optimal transformations, the strict alignments of the images are achieved in the low-rank recovery process. Furthermore, we propose an efficient algorithm based on the method of Alternating Direction Method of Multipliers (ADMM) for the non-convex optimization problem. The extensive experiments on the artificial data sets and real image data sets show the superiority of our method in image alignment and denoising.
更多查看译文
关键词
Face Image, Reconstruction Error, Rank Function, Pepper Noise, Nuclear Norm
AI 理解论文
溯源树
样例
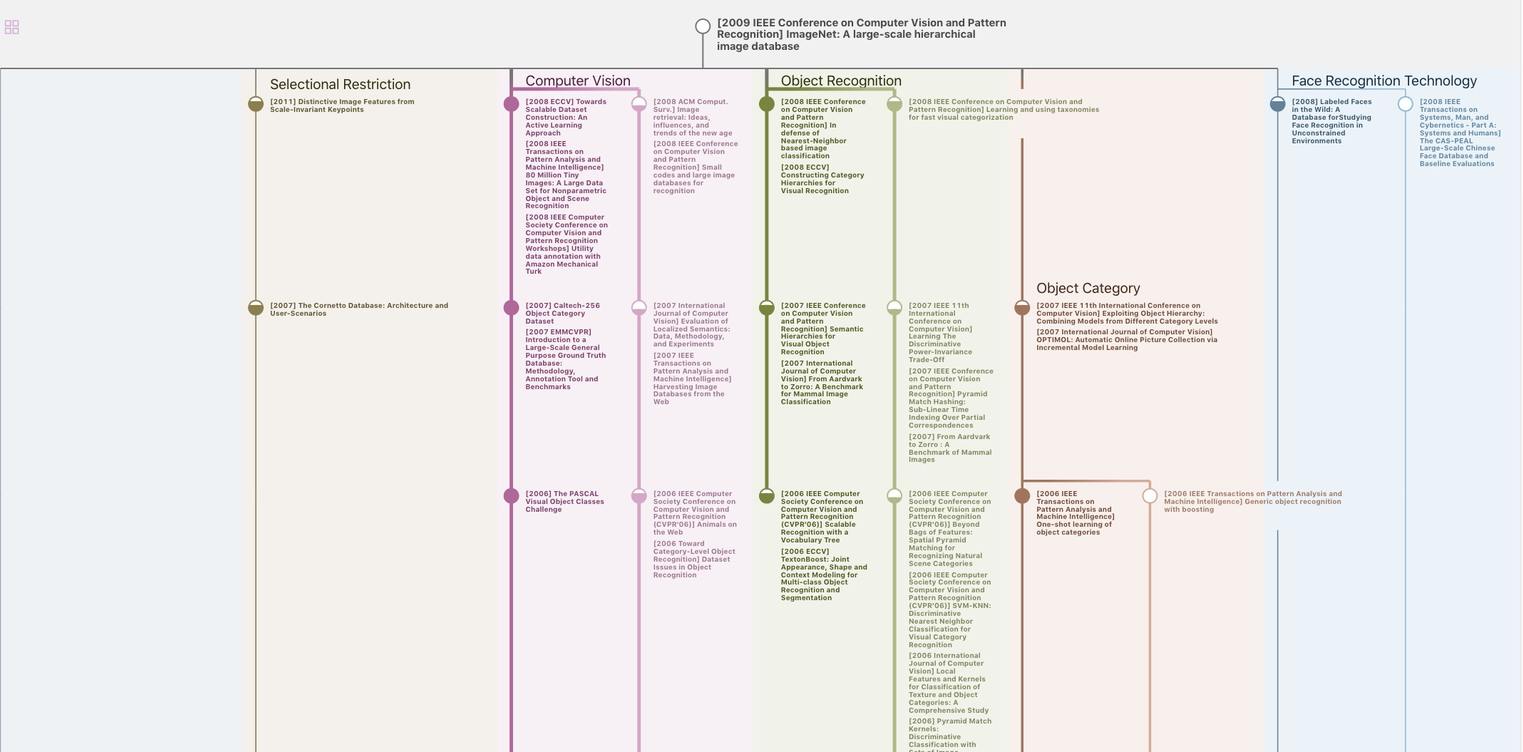
生成溯源树,研究论文发展脉络
Chat Paper
正在生成论文摘要