Adaptive Direct Rgb-D Registration And Mapping For Large Motions
COMPUTER VISION - ACCV 2016, PT IV(2016)
摘要
Dense direct RGB-D registration methods are widely used in tasks ranging from localization and tracking to 3D scene reconstruction. This work addresses a peculiar aspect which drastically limits the applicability of direct registration, namely the weakness of the convergence domain. First, we propose an activation function based on the conditioning of the RGB and ICP point-to-plane error terms. This function strengthens the geometric error influence in the first coarse iterations, while the intensity data term dominates in the finer increments. The information gathered from the geometric and photometric cost functions is not only considered for improving the system observability, but for exploiting the different convergence properties and convexity of each data term. Next, we develop a set of strategies as a flexible regularization and a pixel saliency selection to further improve the quality and robustness of this approach.The methodology is formulated for a generic warping model and results are given using perspective and spherical sensor models. Finally, our method is validated in different RGB-D spherical datasets, including both indoor and outdoor real sequences and using the KITTI VO/SLAM benchmark dataset. We show that the different proposed techniques (weighted activation function, regularization, saliency pixel selection), lead to faster convergence and larger convergence domains, which are the main limitations to the use of direct methods.
更多查看译文
关键词
Geometric Error, Warping Function, Planar Patch, Pixel Selection, Spurious Noise
AI 理解论文
溯源树
样例
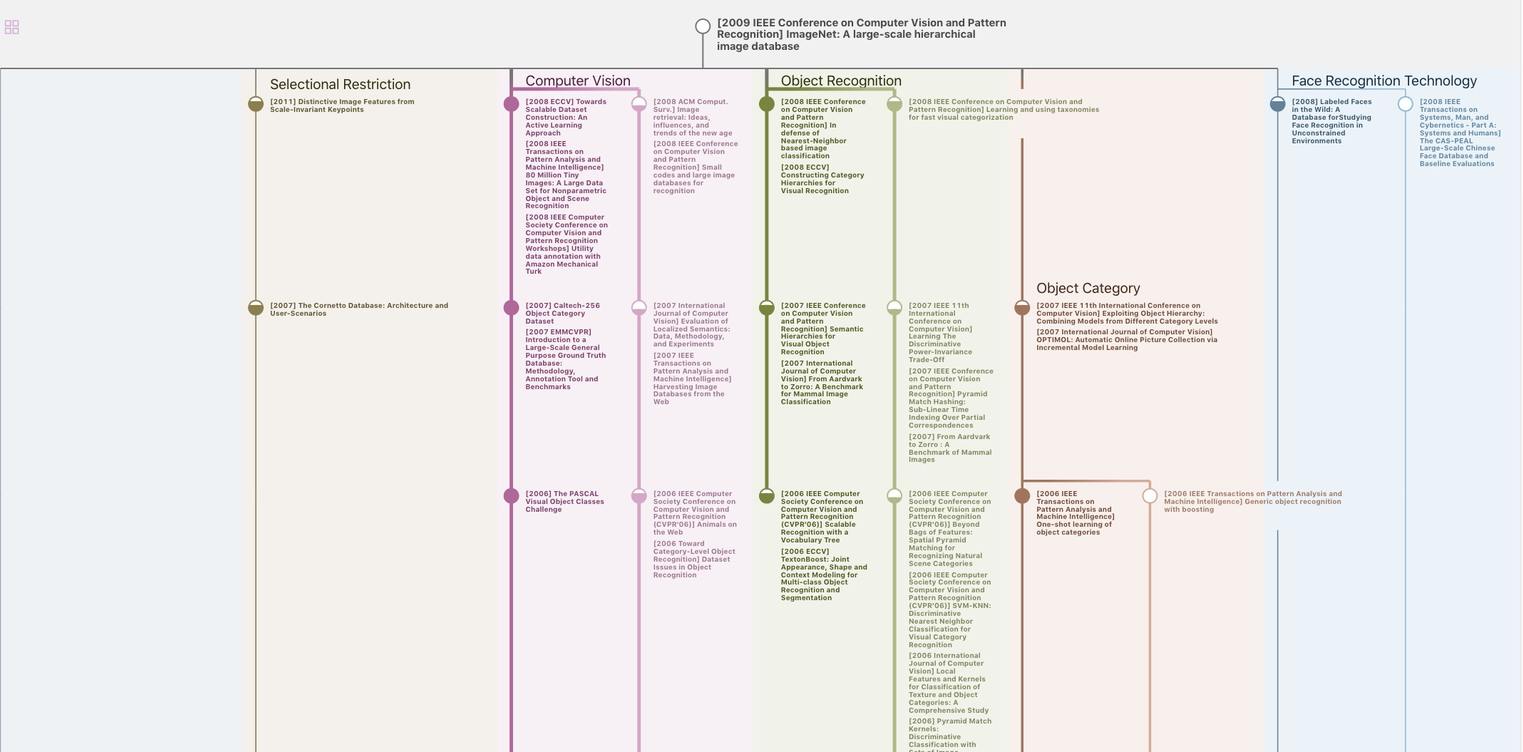
生成溯源树,研究论文发展脉络
Chat Paper
正在生成论文摘要