Robust group-Lasso for functional regression model.
COMMUNICATIONS IN STATISTICS-SIMULATION AND COMPUTATION(2017)
摘要
In this article, we consider the problem of selecting functional variables using the L1 regularization in a functional linear regression model with a scalar response and functional predictors, in the presence of outliers. Since the LASSO is a special case of the penalized least-square regression with L1 penalty function, it suffers from the heavy-tailed errors and/or outliers in data. Recently, Least Absolute Deviation (LAD) and the LASSO methods have been combined (the LAD-LASSO regression method) to carry out robust parameter estimation and variable selection simultaneously for a multiple linear regression model. However, variable selection of the functional predictors based on LASSO fails since multiple parameters exist for a functional predictor. Therefore, group LASSO is used for selecting functional predictors since group LASSO selects grouped variables rather than individual variables. In this study, we propose a robust functional predictor selection method, the LAD-group LASSO, for a functional linear regression model with a scalar response and functional predictors. We illustrate the performance of the LAD-group LASSO on both simulated and real data.
更多查看译文
关键词
Functional regression model,LAD-LASSO,LASSO,Outliers,Variable selection,Primary 60,Secondary 60
AI 理解论文
溯源树
样例
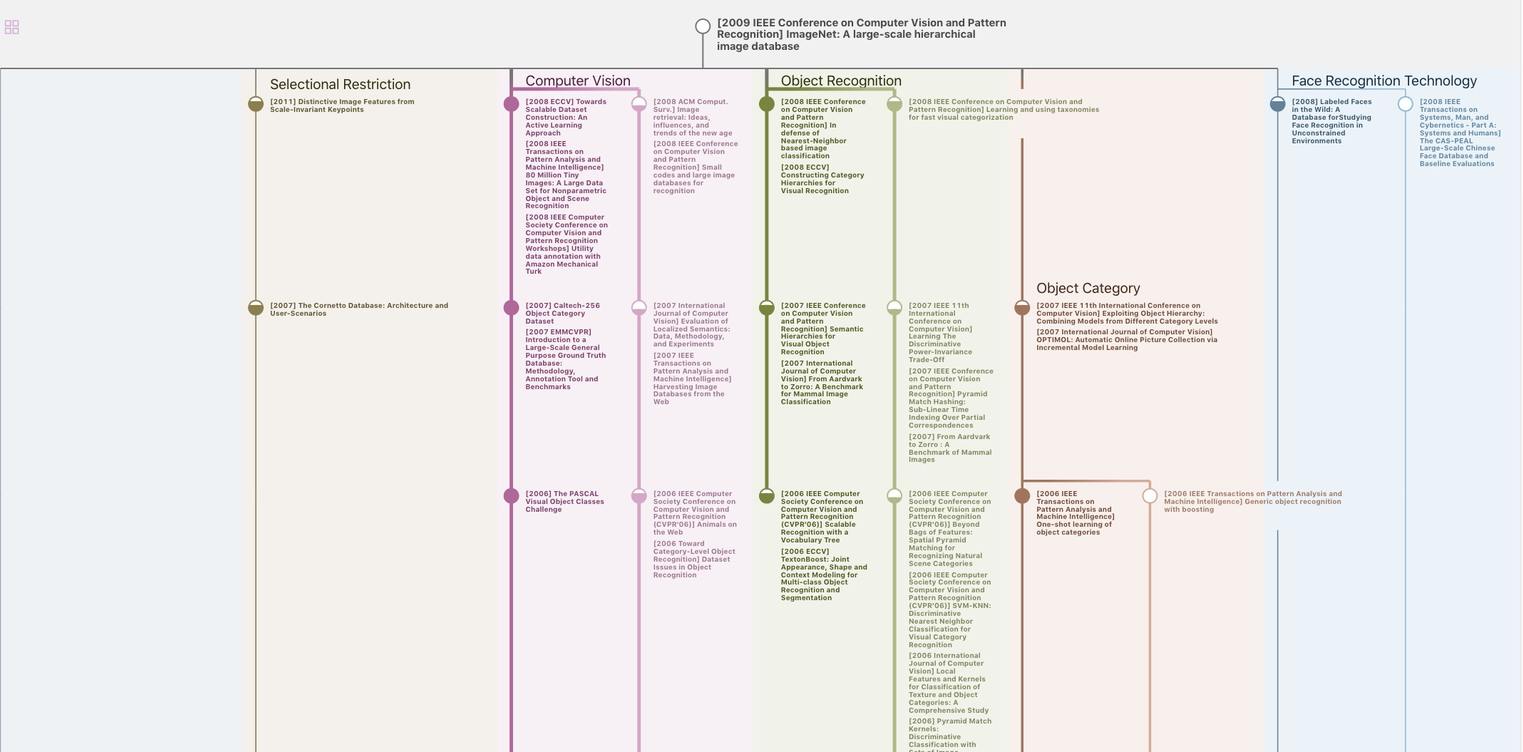
生成溯源树,研究论文发展脉络
Chat Paper
正在生成论文摘要