Quadratic Mutual Information Feature Selection.
ENTROPY(2017)
摘要
We propose a novel feature selection method based on quadratic mutual information which has its roots in Cauchy-Schwarz divergence and Renyi entropy. The method uses the direct estimation of quadratic mutual information from data samples using Gaussian kernel functions, and can detect second order non-linear relations. Its main advantages are: (i) unified analysis of discrete and continuous data, excluding any discretization; and (ii) its parameter-free design. The effectiveness of the proposed method is demonstrated through an extensive comparison with mutual information feature selection (MIFS), minimum redundancy maximum relevance (MRMR), and joint mutual information (JMI) on classification and regression problem domains. The experiments show that proposed method performs comparably to the other methods when applied to classification problems, except it is considerably faster. In the case of regression, it compares favourably to the others, but is slower.
更多查看译文
关键词
feature selection,information-theoretic measures,quadratic mutual information,Cauchy-Schwarz divergence
AI 理解论文
溯源树
样例
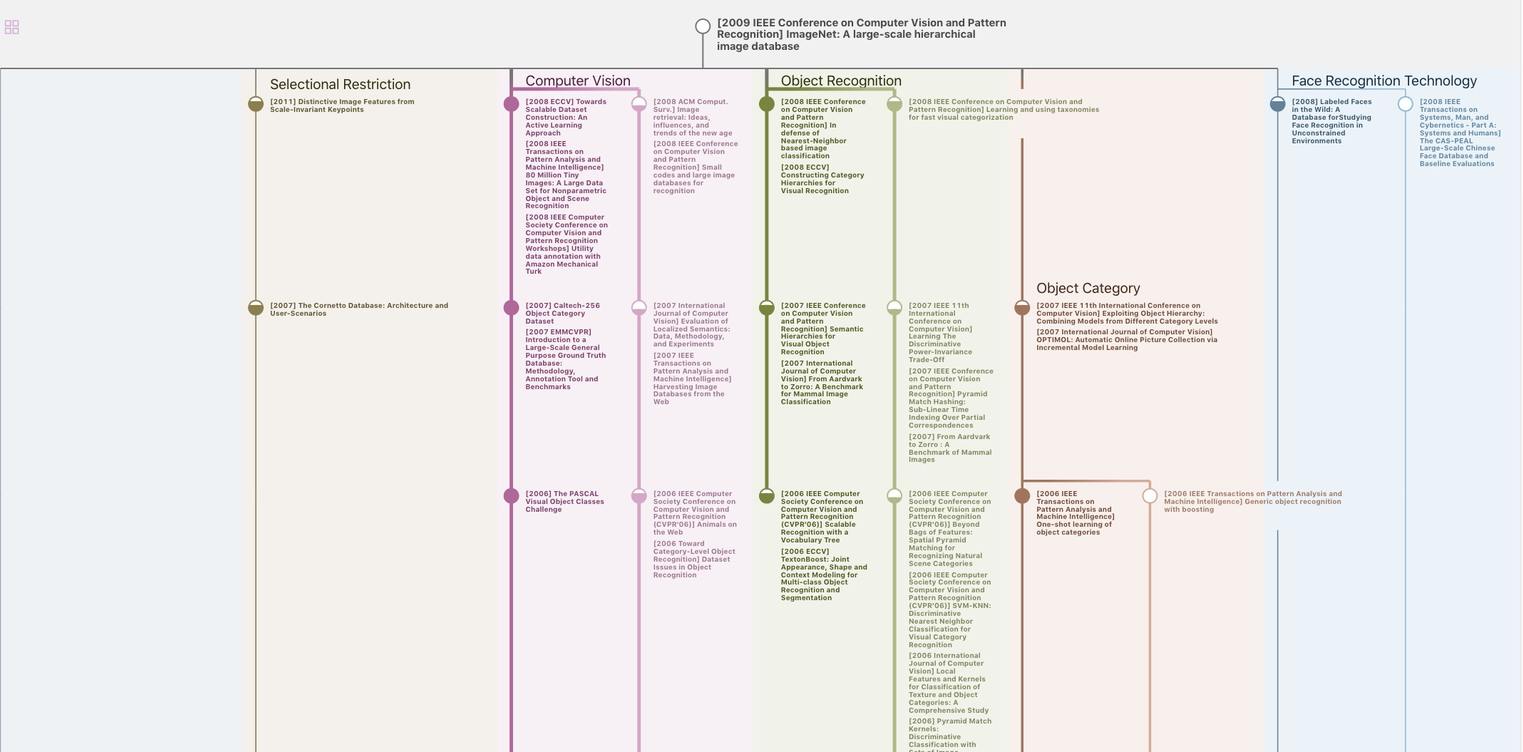
生成溯源树,研究论文发展脉络
Chat Paper
正在生成论文摘要