A Mixed Geographically and Temporally Weighted Regression: Exploring Spatial-Temporal Variations from Global and Local Perspectives.
ENTROPY(2017)
摘要
To capture both global stationarity and spatiotemporal non-stationarity, a novel mixed geographically and temporally weighted regression (MGTWR) model accounting for global and local effects in both space and time is presented. Since the constant and spatial-temporal varying coefficients could not be estimated in one step, a two-stage least squares estimation is introduced to calibrate the model. Both simulations and real-world datasets are used to test and verify the performance of the proposed MGTWR model. Additionally, an Akaike Information Criterion (AIC) is adopted as a key model fitting diagnostic. The experiments demonstrate that the MGTWR model yields more accurate results than do traditional spatially weighted regression models. For instance, the MGTWR model decreased AIC value by 2.7066, 36.368 and 112.812 with respect to those of the mixed geographically weighted regression (MGWR) model and by 45.5628, -38.774 and 35.656 with respect to those of the geographical and temporal weighted regression (GTWR) model for the three simulation datasets. Moreover, compared to the MGWR and GTWR models, the MGTWR model obtained the lowest AIC value and mean square error (MSE) and the highest coefficient of determination (R-2) and adjusted coefficient of determination (R-adj(2)). In addition, our experiments proved the existence of both global stationarity and spatiotemporal non-stationarity, as well as the practical ability of the proposed method.
更多查看译文
关键词
MGTWR,two-stage least squares estimation,global stationarity and spatiotemporal non-stationarity,spatial modeling
AI 理解论文
溯源树
样例
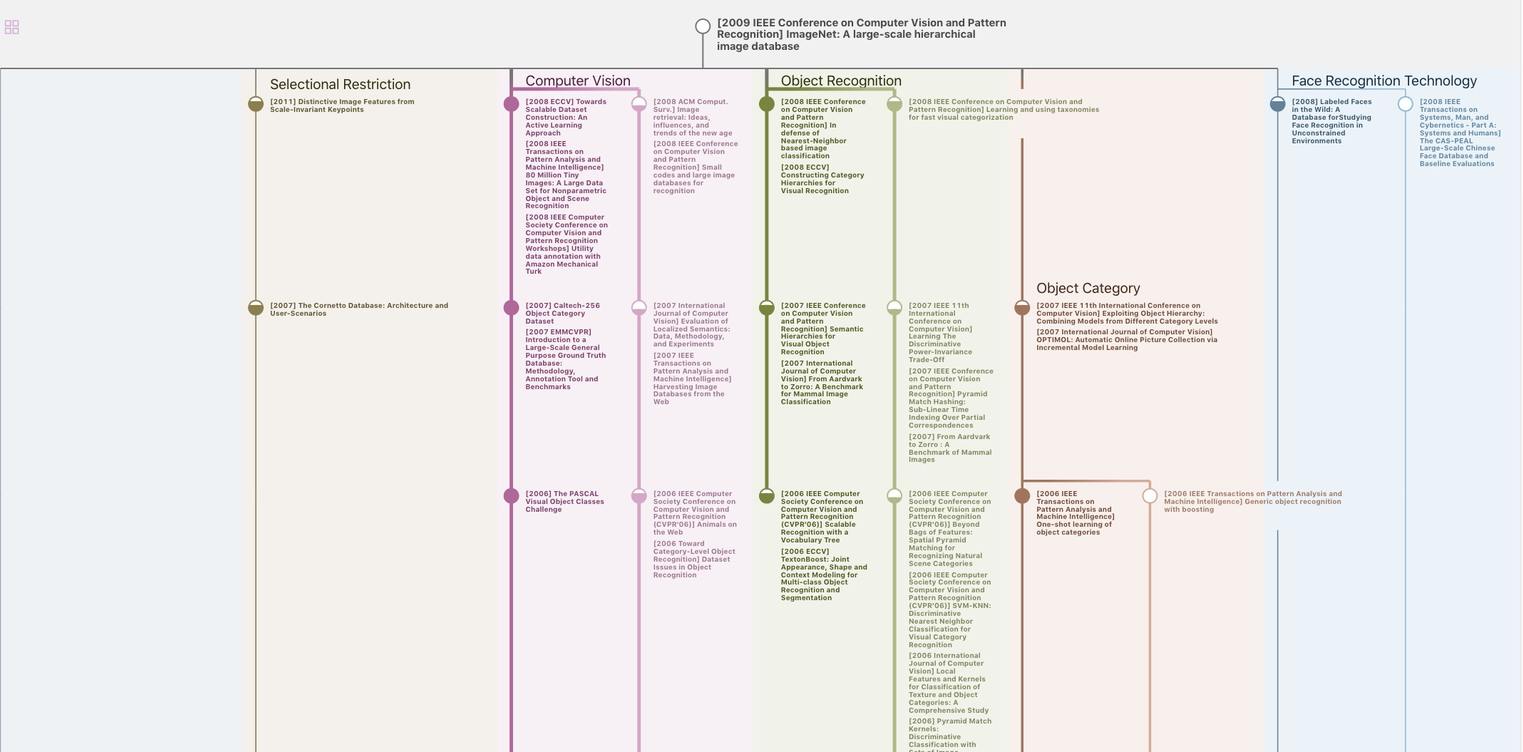
生成溯源树,研究论文发展脉络
Chat Paper
正在生成论文摘要