A locality constrained self-representation approach for unsupervised feature selection.
IJCSE(2017)
摘要
Recently, regularised self-representation RSR has been proposed as an efficient unsupervised feature selection algorithm. However, RSR only takes the self-representation ability of features into account, and neglects the locality structure preserving ability of features, which may degrade its performance. To overcome this limitation, a novel algorithm termed locality constrained regularised self-representation LCRSR is proposed in this paper. In our algorithm, a local scatter matrix is introduced to encode the locality geometric structure of high-dimensional data. Therefore, the locality information of the input database can be well preserved. Moreover, a simple yet efficient iterative update algorithm is developed to solve the proposed LCRSR. Extensive experiments are conducted on five publicly available databases such as JAFFE, ORL, AR, COIL20 and SRBCT to demonstrate the efficiency of the proposed algorithm. Experimental results show that LCRSR obtains better clustering performance than some other state-of-the-art approaches.
更多查看译文
关键词
unsupervised feature selection, self-representation, local structure, clustering
AI 理解论文
溯源树
样例
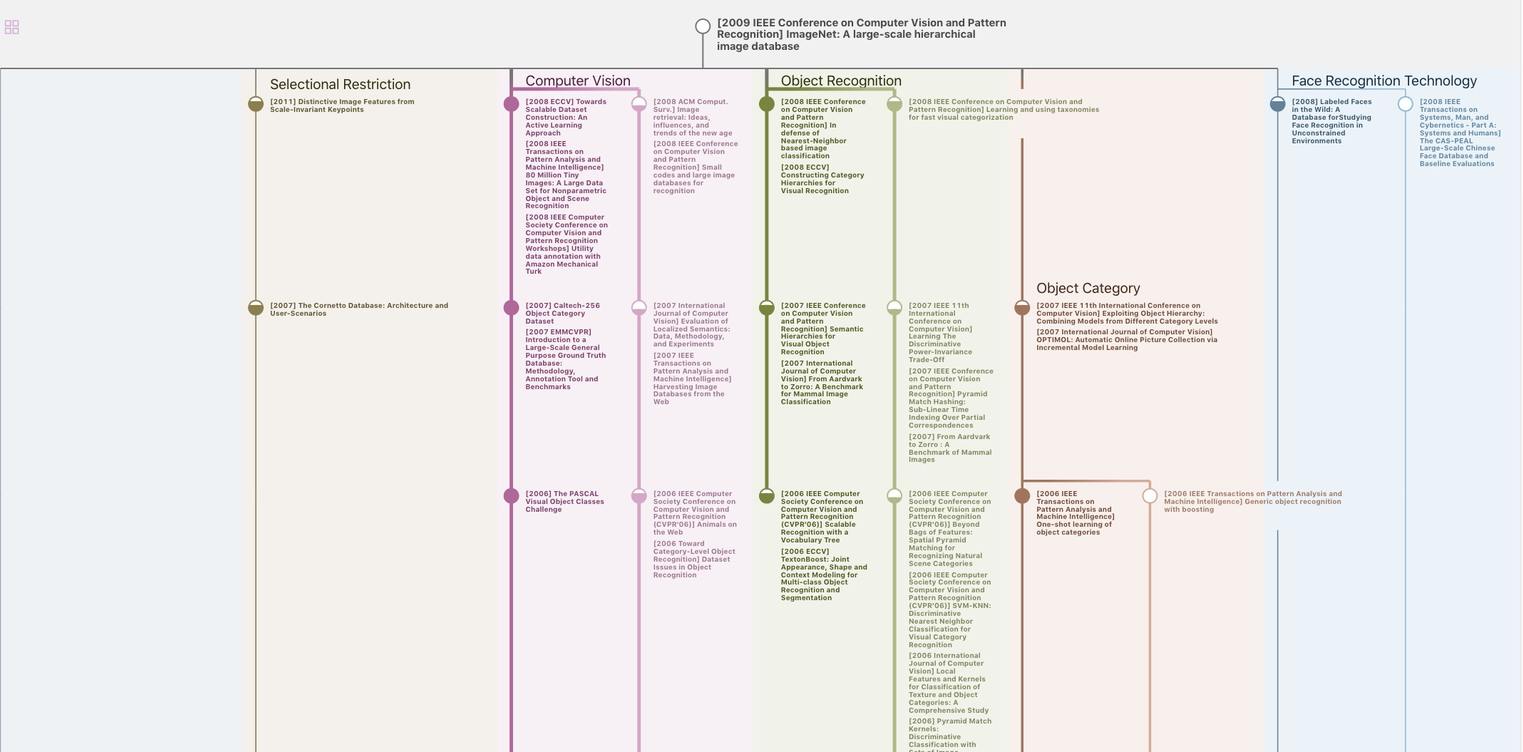
生成溯源树,研究论文发展脉络
Chat Paper
正在生成论文摘要