Recursive Feature Elimination With Ensemble Learning Using Som Variants
INTERNATIONAL JOURNAL OF COMPUTATIONAL INTELLIGENCE AND APPLICATIONS(2017)
摘要
To uncover an appropriate latent subspace for data representation, we propose in this paper a new extension of the random forests method which leads to the unsupervised feature selection called Feature Selection with Random Forests (RFS) based on SOM variants that evaluates the out-of-bag feature importance from a set of partitions. Every partition is created using a several bootstrap samples and a random features subset. We obtain empirical results on 19 benchmark datasets specifying that RFS, boosted with a recursive feature elimination (RFE) method, can lead to important enhancement in terms of clustering accuracy with a very restricted subset of features.Simulations are performed on nine different benchmarks, including face data, handwritten digit data, and document data. Promising experimental results and theoretical analysis prove the efficiency and effectiveness of the proposed method for feature selection in comparison with competitive representative algorithms.
更多查看译文
关键词
Unsupervised learning, self-organizing map variants, random forest, feature selection, recursive feature elimination
AI 理解论文
溯源树
样例
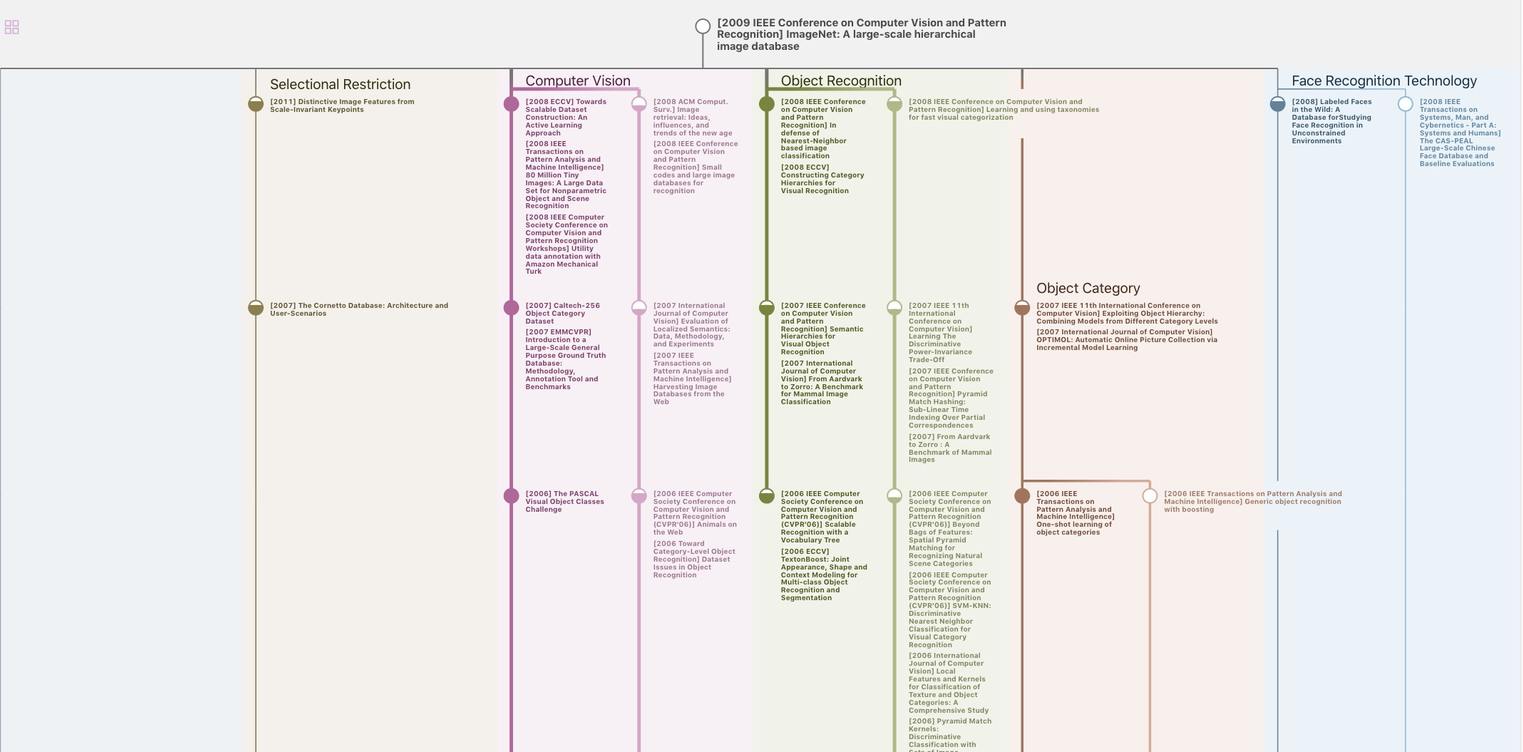
生成溯源树,研究论文发展脉络
Chat Paper
正在生成论文摘要