Robust Estimation of Self-Exciting Generalized Linear Models With Application to Neuronal Modeling.
IEEE Trans. Signal Processing(2017)
摘要
We consider the problem of estimating self-exciting generalized linear models from limited binary observations, where the history of the process serves as the covariate. We analyze the performance of two classes of estimators, namely, the $\\ell _1$-regularized maximum likelihood and greedy estimators, for a canonical self-exciting process and characterize the sampling trade-offs required for stable recovery in the non-asymptotic regime. Our results extend those of compressed sensing for linear and generalized linear models with independent identically distributed covariates to those with highly interdependent covariates. We further provide simulation studies as well as application to real spiking data from the mouse's lateral geniculate nucleus and the ferret's retinal ganglion cells, which agree with our theoretical predictions.
更多查看译文
关键词
History,Maximum likelihood estimation,Data models,Computational modeling,Predictive models,Compressed sensing
AI 理解论文
溯源树
样例
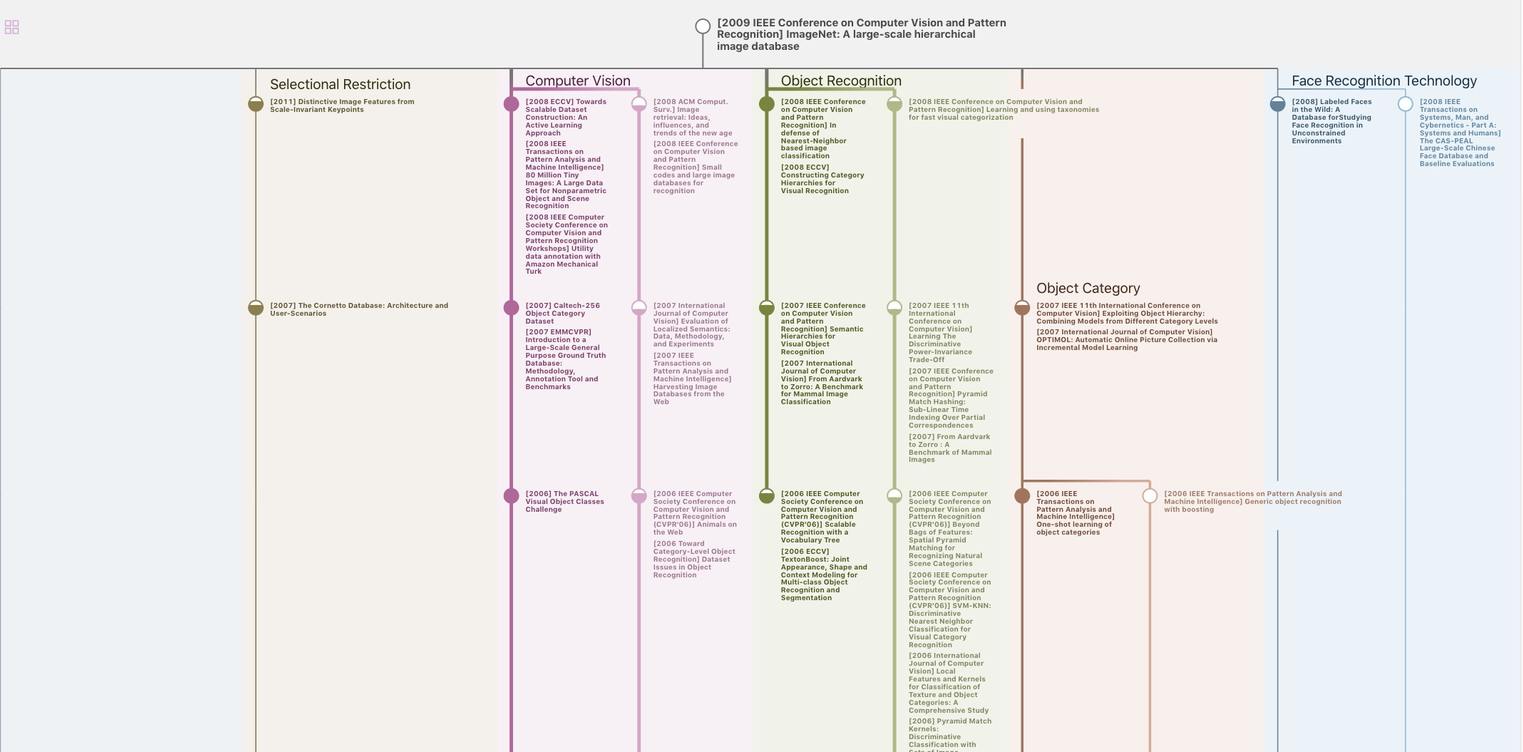
生成溯源树,研究论文发展脉络
Chat Paper
正在生成论文摘要