Exponentially Weighted Ellipsoidal Model For Anomaly Detection
INTERNATIONAL JOURNAL OF INTELLIGENT SYSTEMS(2017)
摘要
Efficient localized data modeling techniques in Internet of Things (IoT) applications enable the nodes to change their behavior upon observing events of interest. Additionally, battery-powered IoT nodes can conserve their energy resources by limiting their data communications to specific events. Despite the real-time nature of the data collected in the IoT and limited memory and computational resources, most of the current data modeling approaches for the IoT involve batch training. Recently, an online efficient anomaly detection technique called iterative data capture anomaly detection has been proposed for environmental sensing and monitoring applications. However, this approach cannot handle changing environments. So far, efforts in extending this algorithm to adapt to changes in the environment have met with limited success. In this paper, we generalize this algorithm to adapt to changes in the data stream by exponentially weighting past observations. We illustrate the proposed algorithm with numerical results on both real-life and simulated data sets, which demonstrate the efficiency and accuracy of our approach compared to existing methods. (C) 2016 Wiley Periodicals, Inc.
更多查看译文
AI 理解论文
溯源树
样例
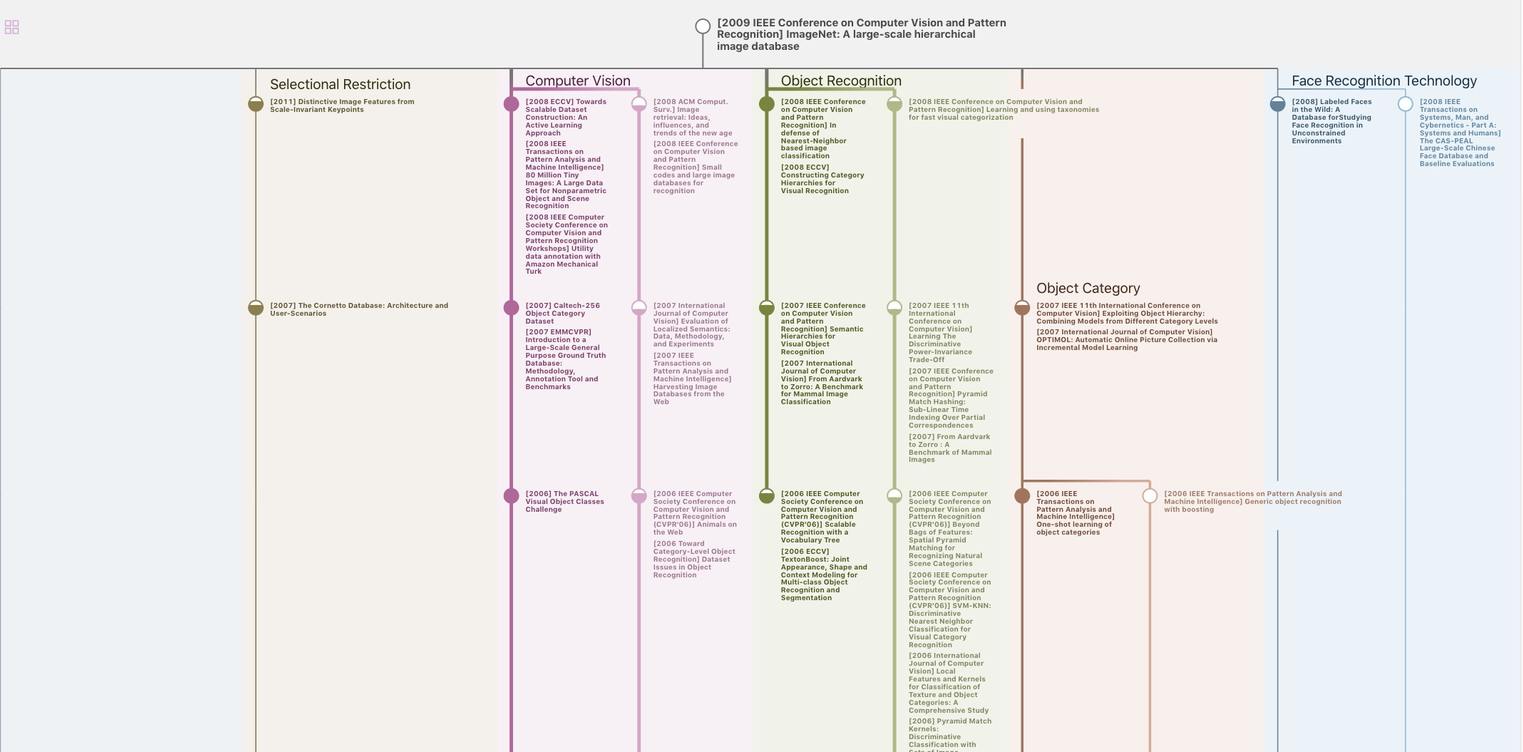
生成溯源树,研究论文发展脉络
Chat Paper
正在生成论文摘要