Deep learning architecture for iris recognition based on optimal Gabor filters and deep belief network.
JOURNAL OF ELECTRONIC IMAGING(2017)
摘要
Gabor filters are widely utilized to detect iris texture information in several state-of-the-art iris recognition systems. However, the proper Gabor kernels and the generative pattern of iris Gabor features need to be predetermined in application. The traditional empirical Gabor filters and shallow iris encoding ways are incapable of dealing with such complex variations in iris imaging including illumination, aging, deformation, and device variations. Thereby, an adaptive Gabor filter selection strategy and deep learning architecture are presented. We first employ particle swarm optimization approach and its binary version to define a set of data-driven Gabor kernels for fitting the most informative filtering bands, and then capture complex pattern from the optimal Gabor filtered coefficients by a trained deep belief network. A succession of comparative experiments validate that our optimal Gabor filters may produce more distinctive Gabor coefficients and our iris deep representations be more robust and stable than traditional iris Gabor codes. Furthermore, the depth and scales of the deep learning architecture are also discussed. (C) 2017 SPIE and IS&T
更多查看译文
关键词
iris recognition,deep learning,Gabor filters,deep belief network,particle swarm optimization
AI 理解论文
溯源树
样例
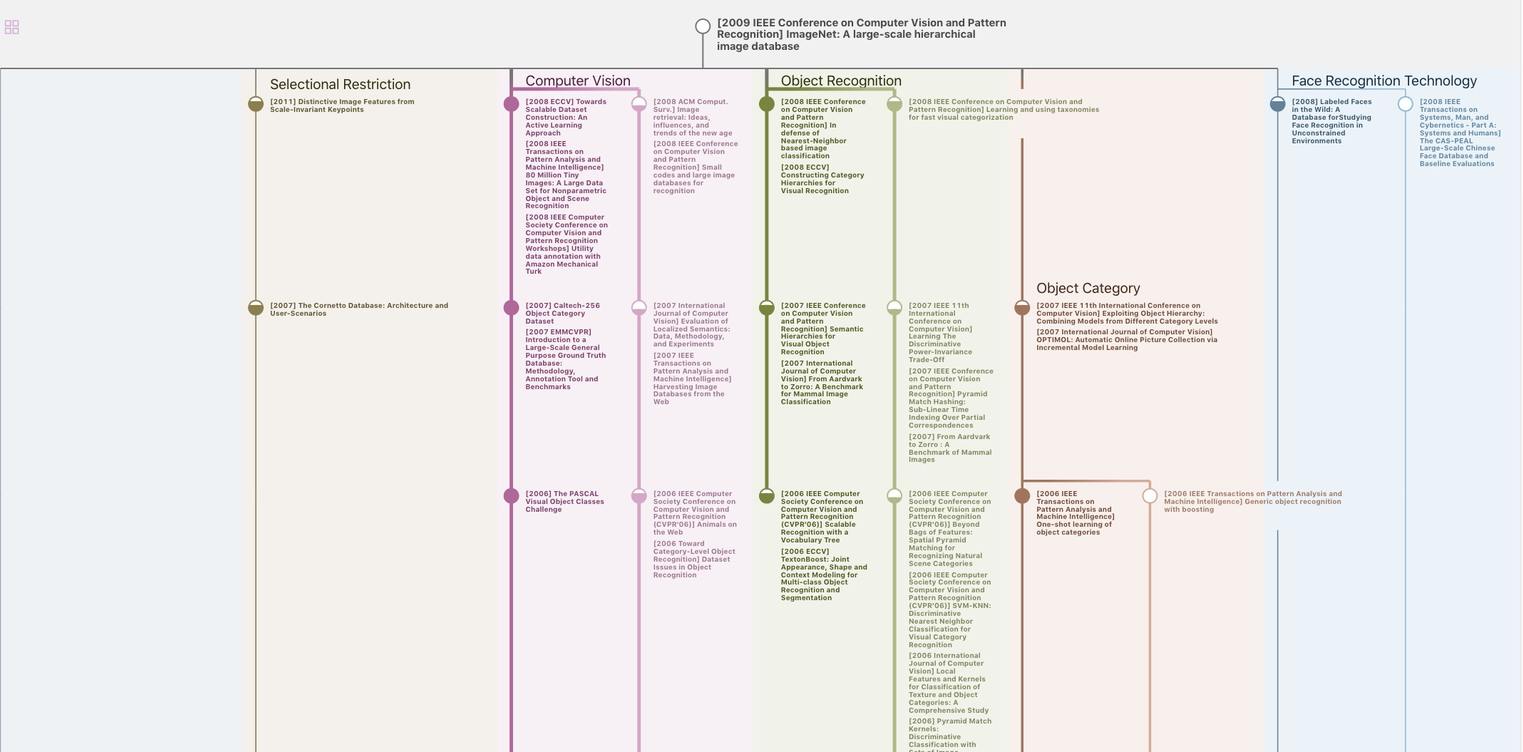
生成溯源树,研究论文发展脉络
Chat Paper
正在生成论文摘要