Optimization-Based Algorithms For Tensor Decompositions: Canonical Polyadic Decomposition, Decomposition In Rank-(L-R, L-R, 1) Terms, And A New Generalization
SIAM J. Optim.(2013)
摘要
The canonical polyadic and rank-(Lr, Lr, 1) block term decomposition (CPD and BTD, respectively) are two closely related tensor decompositions. The CPD and, recently, BTD are important tools in psychometrics, chemometrics, neuroscience, and signal processing. We present a decomposition that generalizes these two and develop algorithms for its computation. Among these algorithms are alternating least squares schemes, several general unconstrained optimization techniques, and matrix-free nonlinear least squares methods. In the latter we exploit the structure of the Jacobian's Gramian to reduce computational and memory cost. Combined with an effective preconditioner, numerical experiments confirm that these methods are among the most efficient and robust currently available for computing the CPD, rank-(Lr, Lr, 1) BTD, and their generalized decomposition.
更多查看译文
关键词
multilinear algebra,tensor decompositions,canonical polyadic decomposition,block term decomposition,optimization,algorithms
AI 理解论文
溯源树
样例
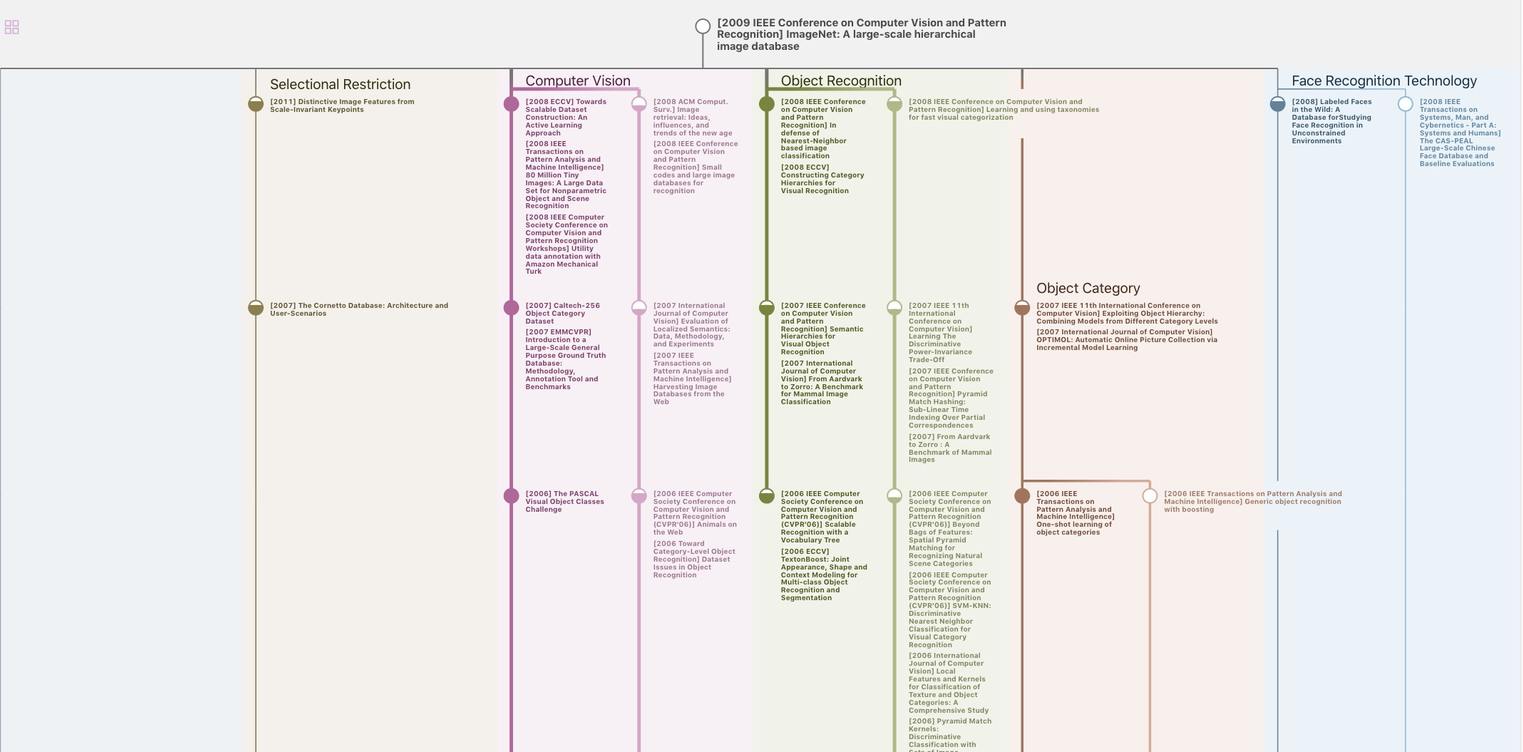
生成溯源树,研究论文发展脉络
Chat Paper
正在生成论文摘要