Self-Trained Stacking Model For Semi-Supervised Learning
INTERNATIONAL JOURNAL ON ARTIFICIAL INTELLIGENCE TOOLS(2017)
摘要
The most important characteristic of semi-supervised learning methods is the combination of available unlabeled data along with an enough smaller set of labeled examples, so as to increase the learning accuracy compared with the default procedure of supervised methods, which on the other hand use only the labeled data during the training phase. In this work, we have implemented a hybrid Self-trained system that combines a Support Vector Machine, a Decision Tree, a Lazy Learner and a Bayesian algorithm using a Stacking variant methodology. We performed an in depth comparison with other well-known Semi-Supervised classification methods on standard benchmark datasets and we finally reached to the point that the presented technique had better accuracy in most cases.
更多查看译文
关键词
Machine learning, semi-supervised methods, stacking theory, classification using labeled and unlabeled data
AI 理解论文
溯源树
样例
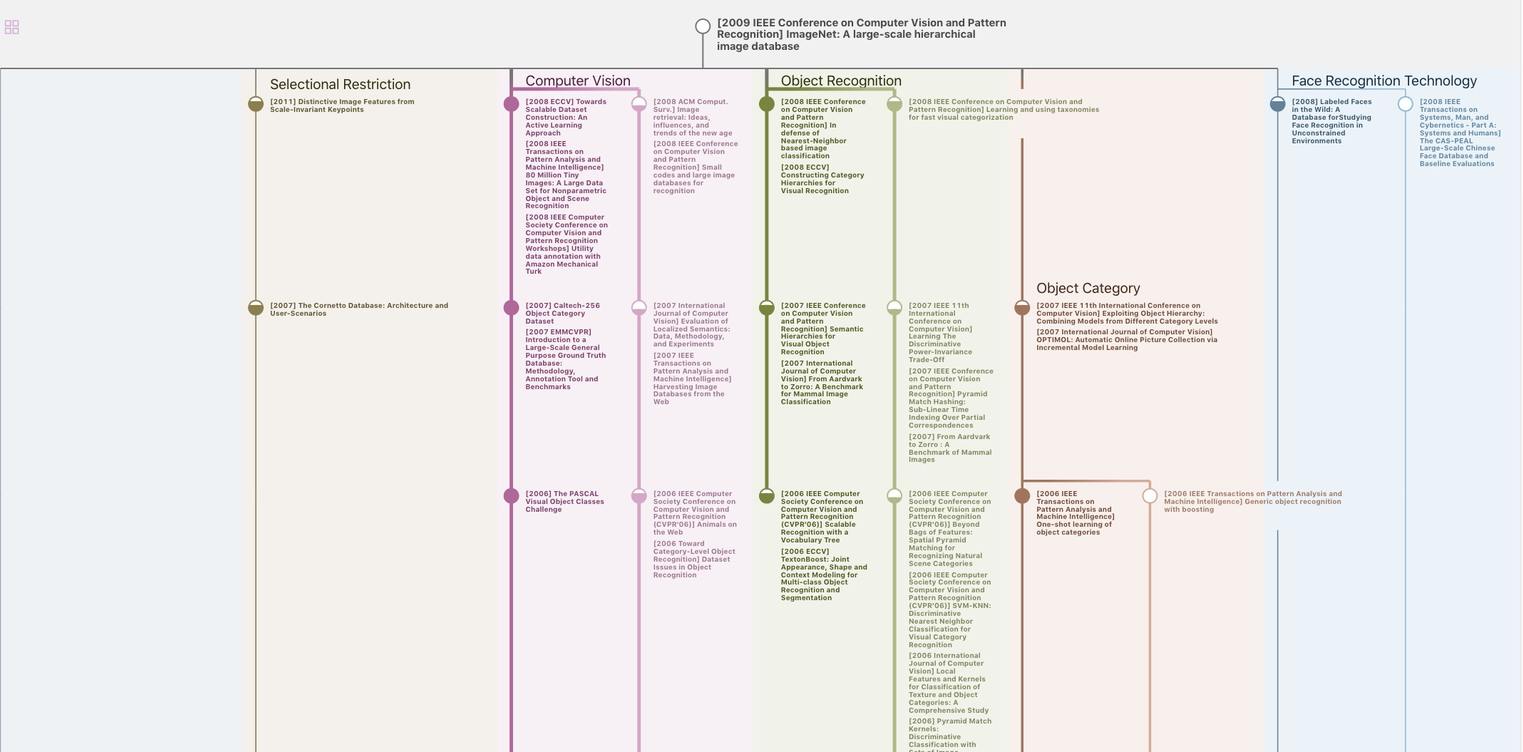
生成溯源树,研究论文发展脉络
Chat Paper
正在生成论文摘要