Are Saddles Good Enough for Deep Learning?
arXiv: Machine Learning(2017)
摘要
Recent years have seen a growing interest in understanding deep neural networks from an optimization perspective. It is understood now that converging to low-cost local minima is sufficient for such models to become effective in practice. However, in this work, we propose a new hypothesis based on recent theoretical findings and empirical studies that deep neural network models actually converge to saddle points with high degeneracy. Our findings from this work are new, and can have a significant impact on the development of gradient descent based methods for training deep networks. We validated our hypotheses using an extensive experimental evaluation on standard datasets such as MNIST and CIFAR-10, and also showed that recent efforts that attempt to escape saddles finally converge to saddles with high degeneracy, which we define as `good saddlesu0027. We also verified the famous Wigneru0027s Semicircle Law in our experimental results.
更多查看译文
关键词
saddles,deep learning
AI 理解论文
溯源树
样例
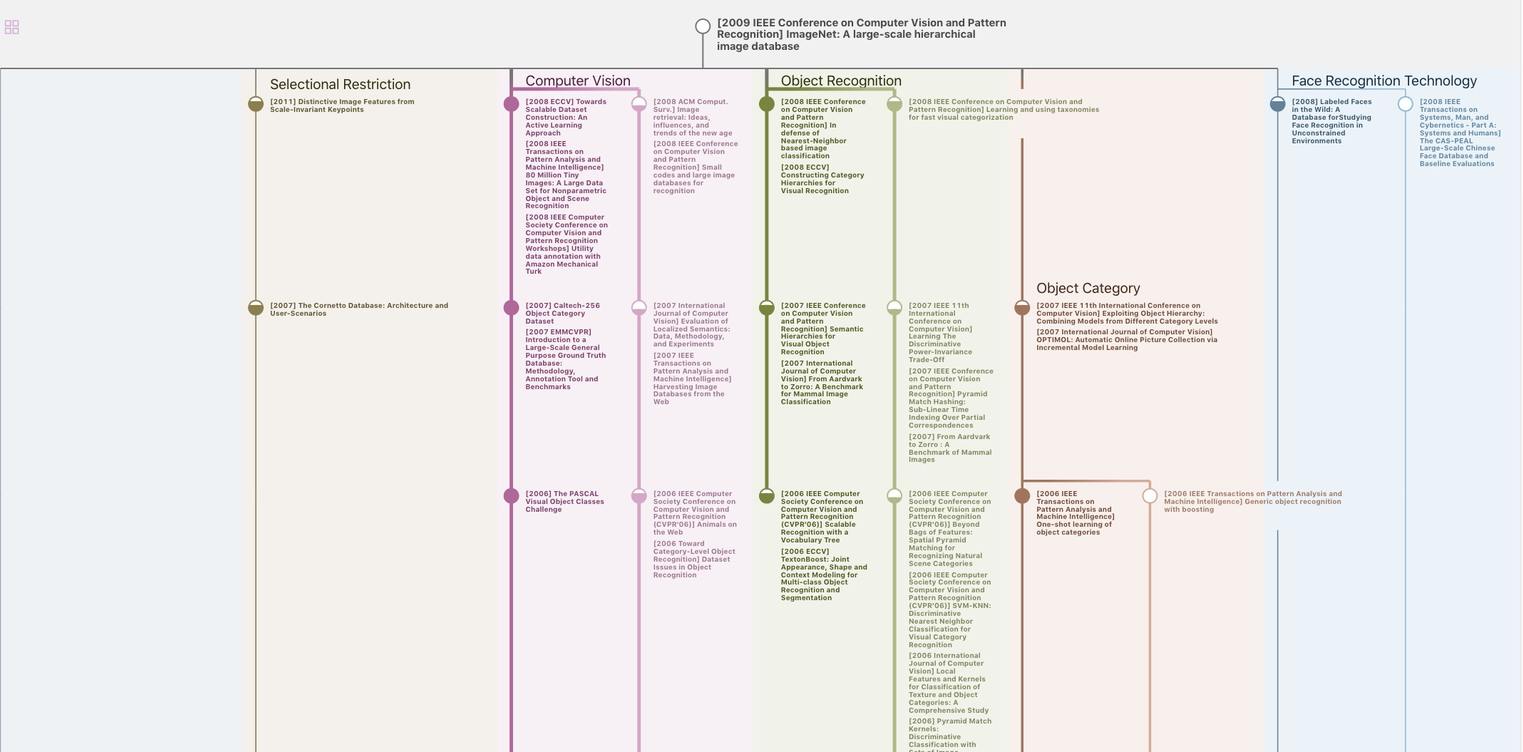
生成溯源树,研究论文发展脉络
Chat Paper
正在生成论文摘要