Crowdsensing in Opportunistic Mobile Social Networks: A Context-aware and Human-centric Approach
arXiv: Social and Information Networks(2017)
摘要
In recent years, there have been efforts to collect human contact traces during social events (e.g., conferences) using Bluetooth devices (e.g., mobile phones, iMotes). The results of these studies have enabled the ability to do the crowd-sourcing task from within the crowd, in order to answer questions, such as: what is the current density of the crowd, or how many people are attending the event? However, in those studies, the sensing devices are usually distributed and configured in a certain manner. For example, the number of devices is fixed, people register for the devices on a volunteering basis. In this paper, we treat the above problem as an optimization problem and draw the connection to the vertex cover problem in graph theory. Since finding the optimal solution for minimum vertex cover problem is NP-complete, approximation algorithms have to be used. However, we will show that the well-known approximation algorithms do not perform well with the crowd-sensing task. In this paper, we propose the notions of node observability and coverage utility score and design a new context-aware approximation algorithm to find vertex cover that is tailored for crowd-sensing task. In addition, we design human-centric bootstrapping strategies to make initial assignment of sensing devices based on meta information about the participants (e.g., interests, friendship). The motivation is to assign the sensing task to a more "socialized" device to obtain better sensing coverage. We perform comprehensive experiments on real-world data traces obtained from previous experimental studies in conference and academic social context. The results show that our proposed approach significantly outperforms the baseline approximation algorithms in terms of sensing coverage.
更多查看译文
关键词
opportunistic mobile social networks,social networks,context-aware,human-centric
AI 理解论文
溯源树
样例
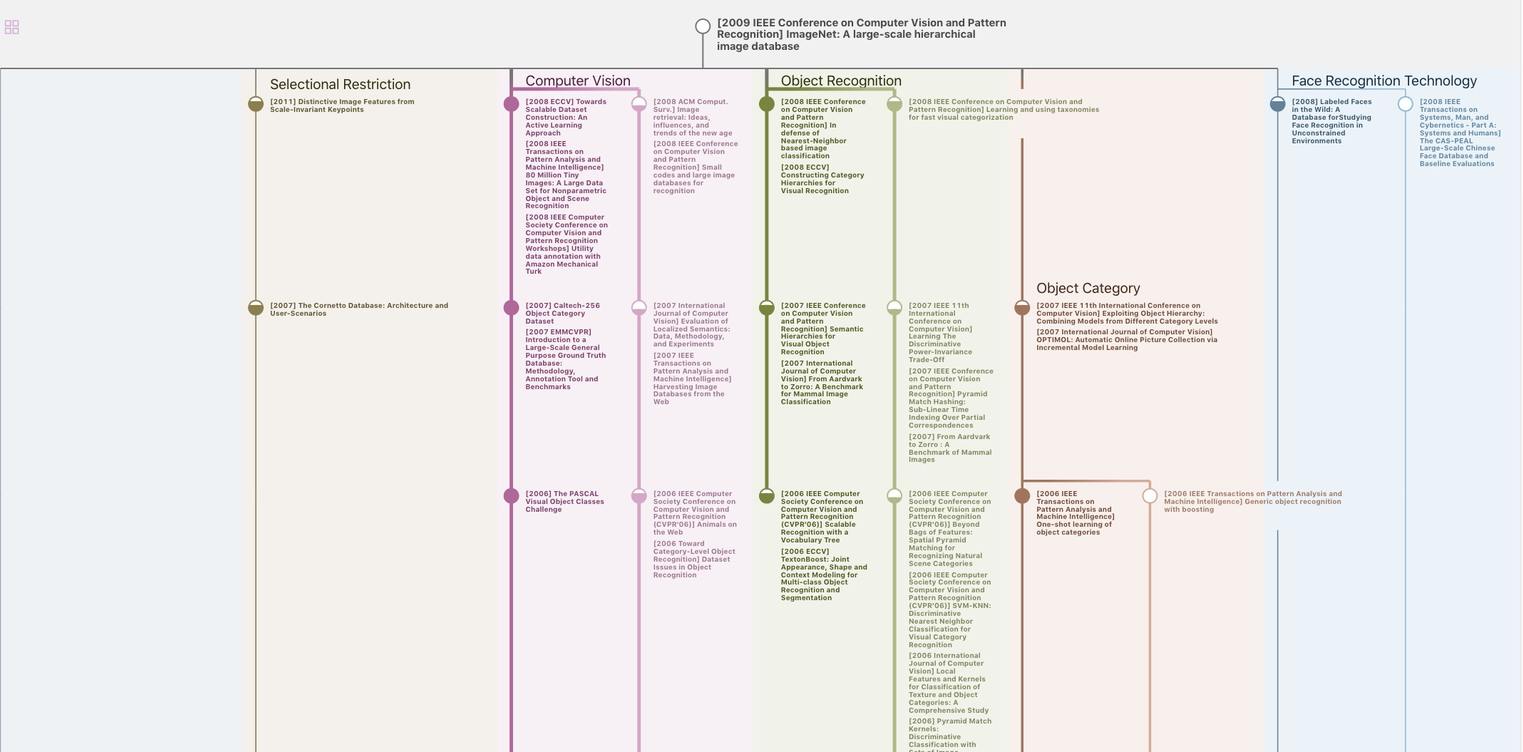
生成溯源树,研究论文发展脉络
Chat Paper
正在生成论文摘要