LabelBank: Revisiting Global Perspectives for Semantic Segmentation.
arXiv: Computer Vision and Pattern Recognition(2017)
摘要
Semantic segmentation requires a detailed labeling of image pixels by object category. Information derived from local image patches is necessary to describe the detailed shape of individual objects. However, this information is ambiguous and can result in noisy labels. Global inference of image content can instead capture the general semantic concepts present. advocate that holistic inference of image concepts provides valuable information for detailed pixel labeling. propose a generic framework to leverage holistic information in the form of a LabelBank for pixel-level segmentation. We show the ability of our framework to improve semantic segmentation performance in a variety of settings. learn models for extracting a holistic LabelBank from visual cues, attributes, and/or textual descriptions. demonstrate improvements in semantic segmentation accuracy on standard datasets across a range of state-of-the-art segmentation architectures and holistic inference approaches.
更多查看译文
AI 理解论文
溯源树
样例
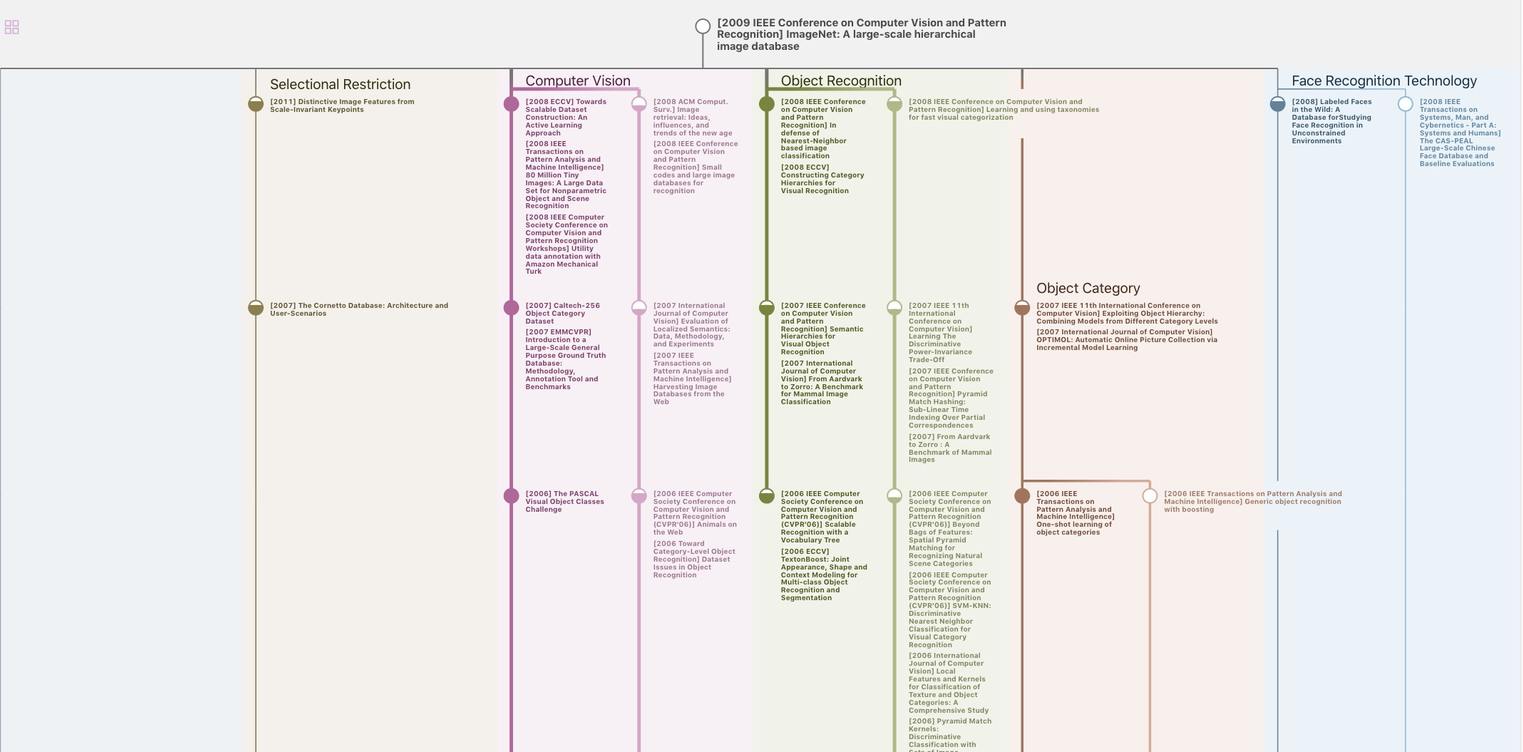
生成溯源树,研究论文发展脉络
Chat Paper
正在生成论文摘要