High-Level Concepts for Affective Understanding of Images
2017 IEEE Winter Conference on Applications of Computer Vision (WACV)(2017)
摘要
This paper aims to bridge the affective gap between image content and the emotional response of the viewer it elicits by using High-Level Concepts (HLCs). In contrast to previous work that relied solely on low-level features or used convolutional neural network (CNN) as a blackbox, we use HLCs generated by pretrained CNNs in an explicit way to investigate the relations/associations between these HLCs and a (small) set of Ekman's emotional classes. As a proof-of-concept, we first propose a linear admixture model for modeling these relations, and the resulting computational framework allows us to determine the associations between each emotion class and certain HLCs (objects and places). This linear model is further extended to a nonlinear model using support vector regression (SVR) that aims to predict the viewer's emotional response using both low-level image features and HLCs extracted from images. These class-specific regressors are then assembled into a regressor ensemble that provide a flexible and effective predictor for predicting viewer's emotional responses from images. Experimental results have demonstrated that our results are comparable to existing methods, with a clear view of the association between HLCs and emotional classes that is ostensibly missing in most existing work.
更多查看译文
关键词
high-level concepts,affective image understanding,image content,viewer emotional response,HLC,emotional classes,linear admixture model,nonlinear model,support vector regression,SVR,low-level image features,class-specific regressors,regressor ensemble
AI 理解论文
溯源树
样例
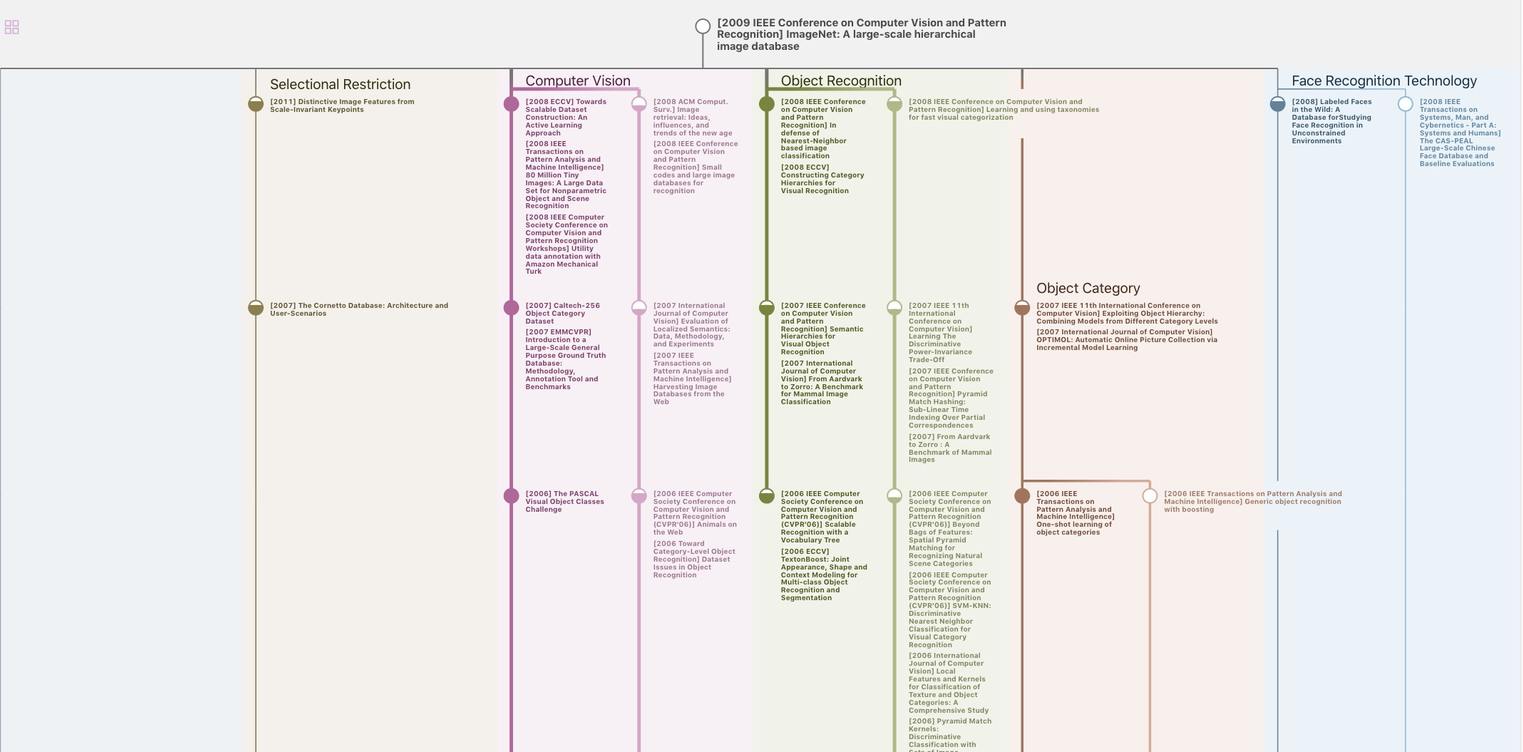
生成溯源树,研究论文发展脉络
Chat Paper
正在生成论文摘要