Deep Functional Maps: Structured Prediction for Dense Shape Correspondence
2017 IEEE International Conference on Computer Vision (ICCV)(2017)
摘要
We introduce a new framework for learning dense correspondence between deformable 3D shapes. Existing learning based approaches model shape correspondence as a labelling problem, where each point of a query shape receives a label identifying a point on some reference domain; the correspondence is then constructed a posteriori by composing the label predictions of two input shapes. We propose a paradigm shift and design a structured prediction model in the space of functional maps, linear operators that provide a compact representation of the correspondence. We model the learning process via a deep residual network which takes dense descriptor fields defined on two shapes as input, and outputs a soft map between the two given objects. The resulting correspondence is shown to be accurate on several challenging benchmarks comprising multiple categories, synthetic models, real scans with acquisition artifacts, topological noise, and partiality.
更多查看译文
关键词
deformable 3D shapes,model shape correspondence,dense shape correspondence learning,label predictions,query shape,deep functional maps,dense descriptor fields,deep residual network
AI 理解论文
溯源树
样例
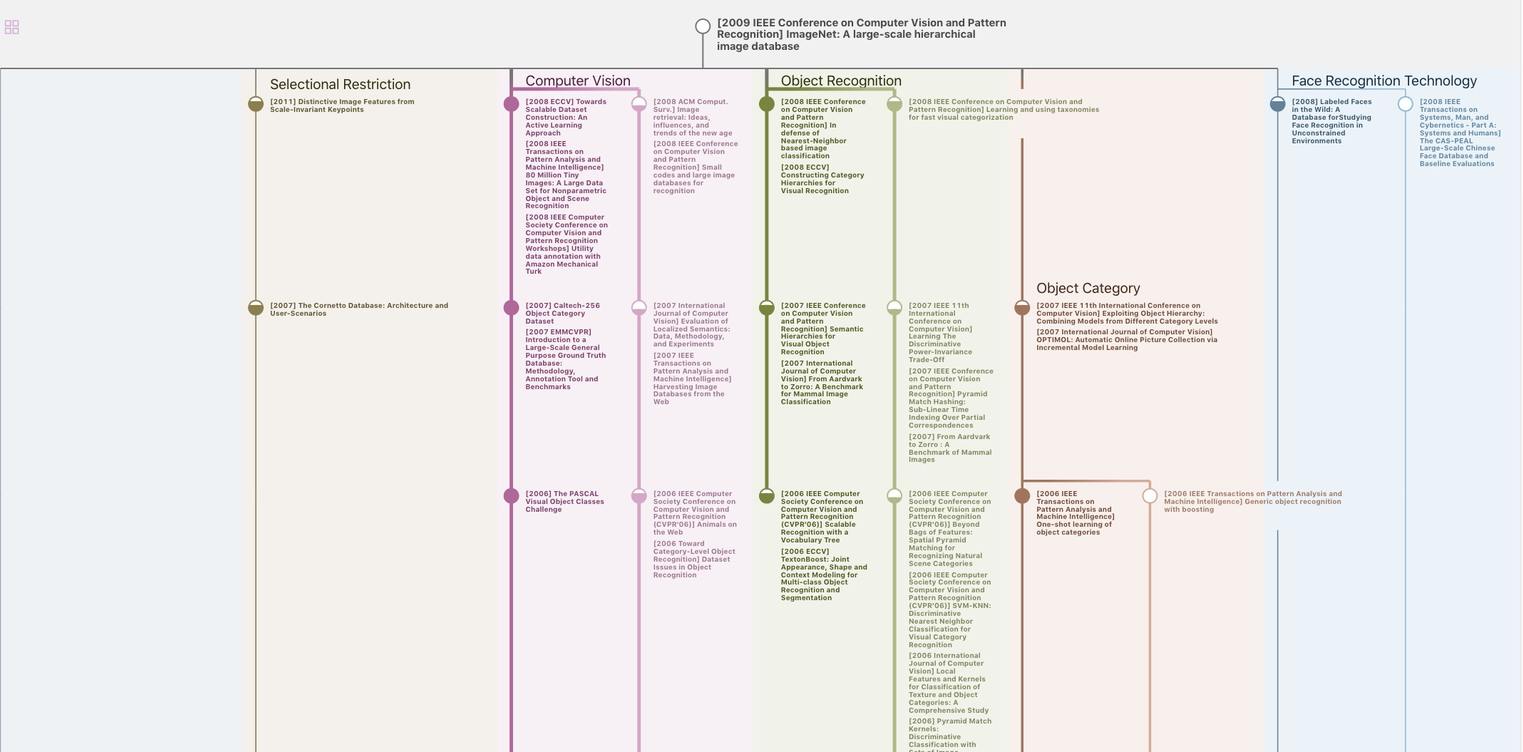
生成溯源树,研究论文发展脉络
Chat Paper
正在生成论文摘要