Recurrent collective classification
Knowledge and Information Systems(2018)
摘要
We propose a new method for training iterative collective classifiers for labeling nodes in network data. The iterative classification algorithm (ICA) is a canonical method for incorporating relational information into classification. Yet, existing methods for training ICA models rely on the assumption that relational features reflect the true labels of the nodes. This unrealistic assumption introduces a bias that is inconsistent with the actual prediction algorithm. In this paper, we introduce recurrent collective classification (RCC), a variant of ICA analogous to recurrent neural network prediction. RCC accommodates any differentiable local classifier and relational feature functions. We provide gradient-based strategies for optimizing over model parameters to more directly minimize the loss function. In our experiments, this direct loss minimization translates to improved accuracy and robustness on real network data. We demonstrate the robustness of RCC in settings where local classification is very noisy, settings that are particularly challenging for ICA.
更多查看译文
关键词
Collective classification,Recurrent neural network,Iterative classification algorithm
AI 理解论文
溯源树
样例
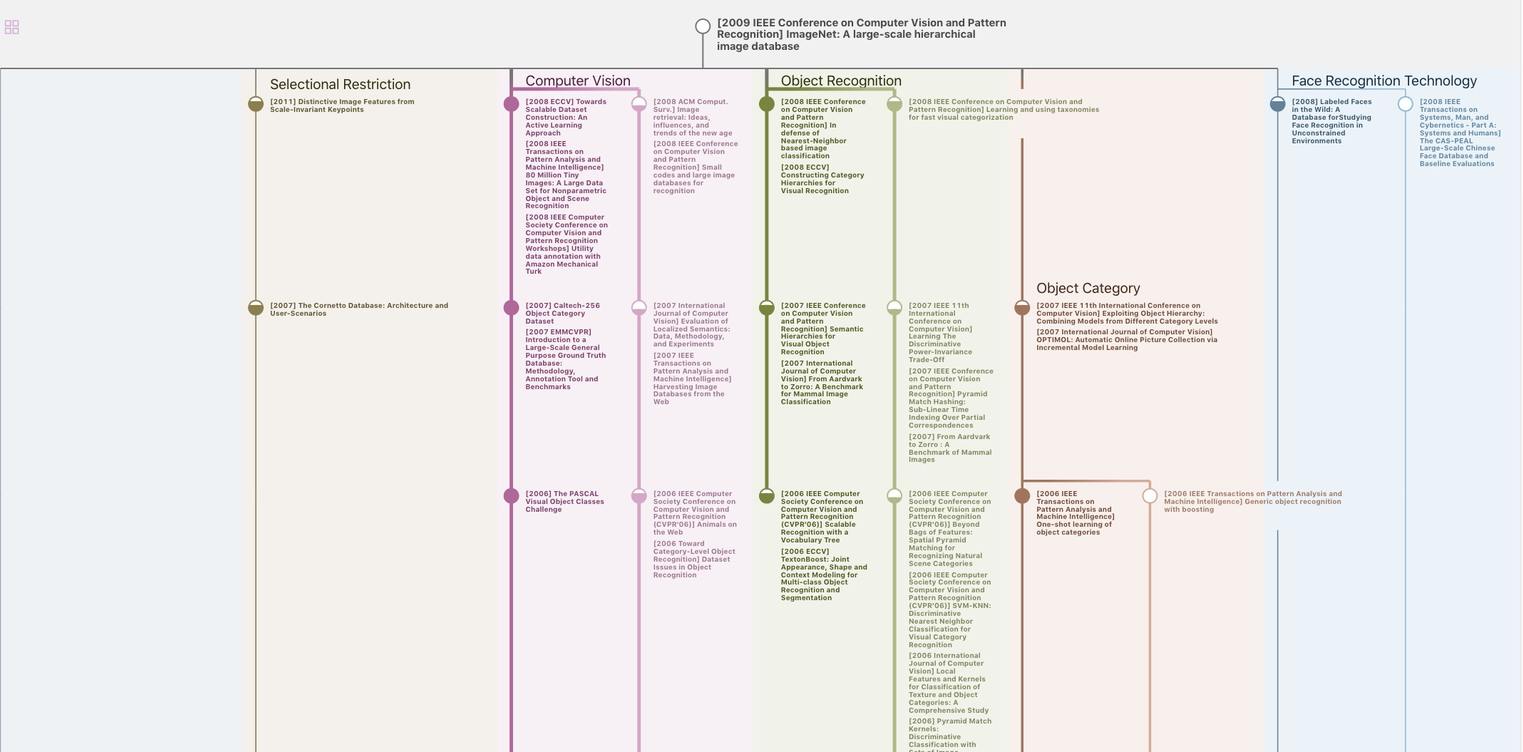
生成溯源树,研究论文发展脉络
Chat Paper
正在生成论文摘要