Unbiasing Truncated Backpropagation Through Time.
arXiv: Neural and Evolutionary Computing(2018)
摘要
emph{Truncated Backpropagation Through Time} (truncated BPTT, cite{jaeger2002tutorial}) is a widespread method for learning recurrent computational graphs. Truncated BPTT keeps the computational benefits of emph{Backpropagation Through Time} (BPTT cite{werbos:bptt}) while relieving the need for a complete backtrack through the whole data sequence at every step. However, truncation favors short-term dependencies: the gradient estimate of truncated BPTT is biased, so that it does not benefit from the convergence guarantees from stochastic gradient theory. We introduce emph{Anticipated Reweighted Truncated Backpropagation} (ARTBP), an algorithm that keeps the computational benefits of truncated BPTT, while providing unbiasedness. ARTBP works by using variable truncation lengths together with carefully chosen compensation factors in the backpropagation equation. We check the viability of ARTBP on two tasks. First, a simple synthetic task where careful balancing of temporal dependencies at different scales is needed: truncated BPTT displays unreliable performance, and in worst case scenarios, divergence, while ARTBP converges reliably. Second, on Penn Treebank character-level language modelling cite{ptb_proc}, ARTBP slightly outperforms truncated BPTT.
更多查看译文
AI 理解论文
溯源树
样例
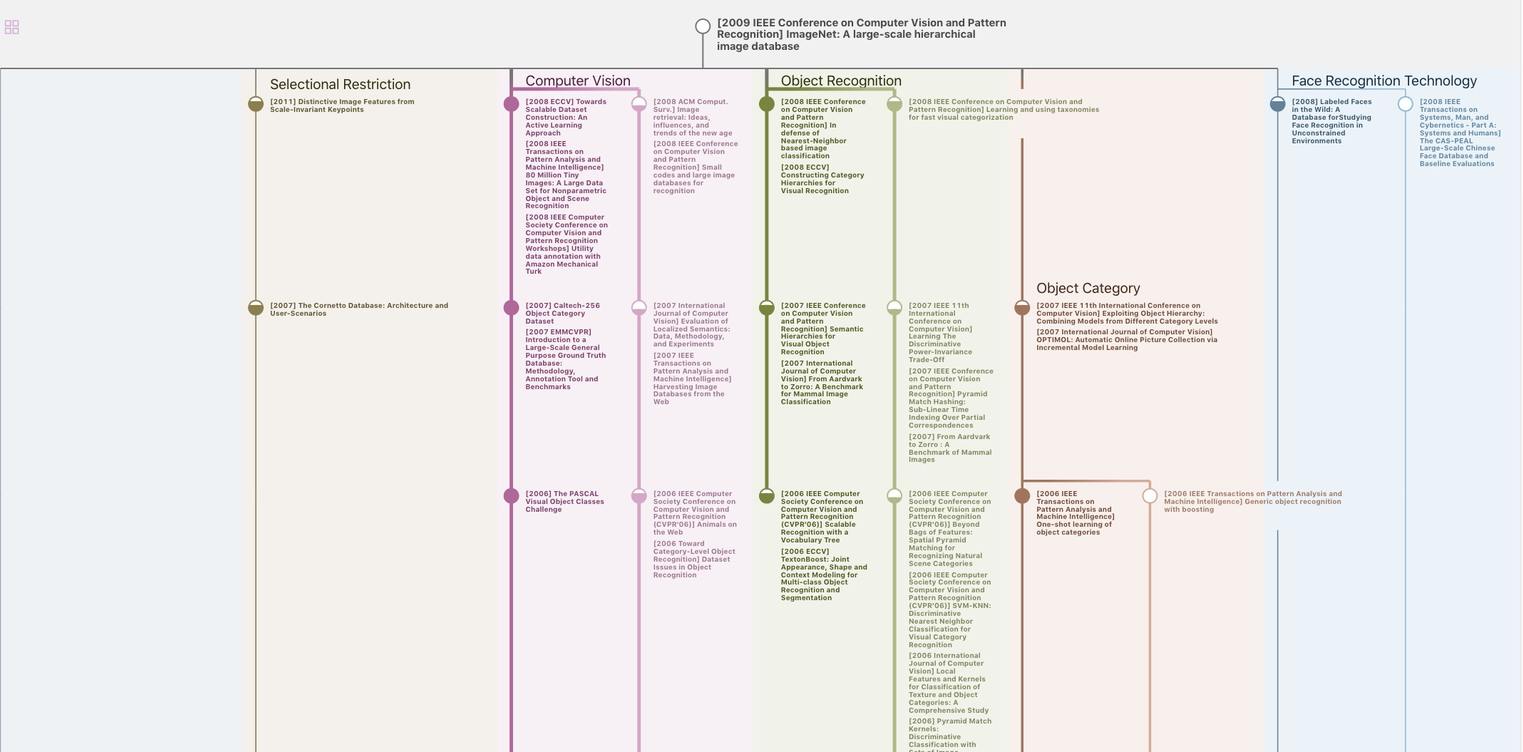
生成溯源树,研究论文发展脉络
Chat Paper
正在生成论文摘要