Learning Local Receptive Fields and their Weight Sharing Scheme on Graphs
2017 IEEE Global Conference on Signal and Information Processing (GlobalSIP)(2017)
摘要
We propose a simple and generic layer formulation that extends the properties of convolutional layers to any domain that can be described by a graph. Namely, we use the support of its adjacency matrix to design learnable weight sharing filters able to exploit the underlying structure of signals in the same fashion as for images. The proposed formulation makes it possible to learn the weights of the filter as well as a scheme that controls how they are shared across the graph. We perform validation experiments with image datasets and show that these filters offer performances comparable with convolutional ones.
更多查看译文
关键词
deep learning,convolutional neural networks,local receptive fields,graph signal processing
AI 理解论文
溯源树
样例
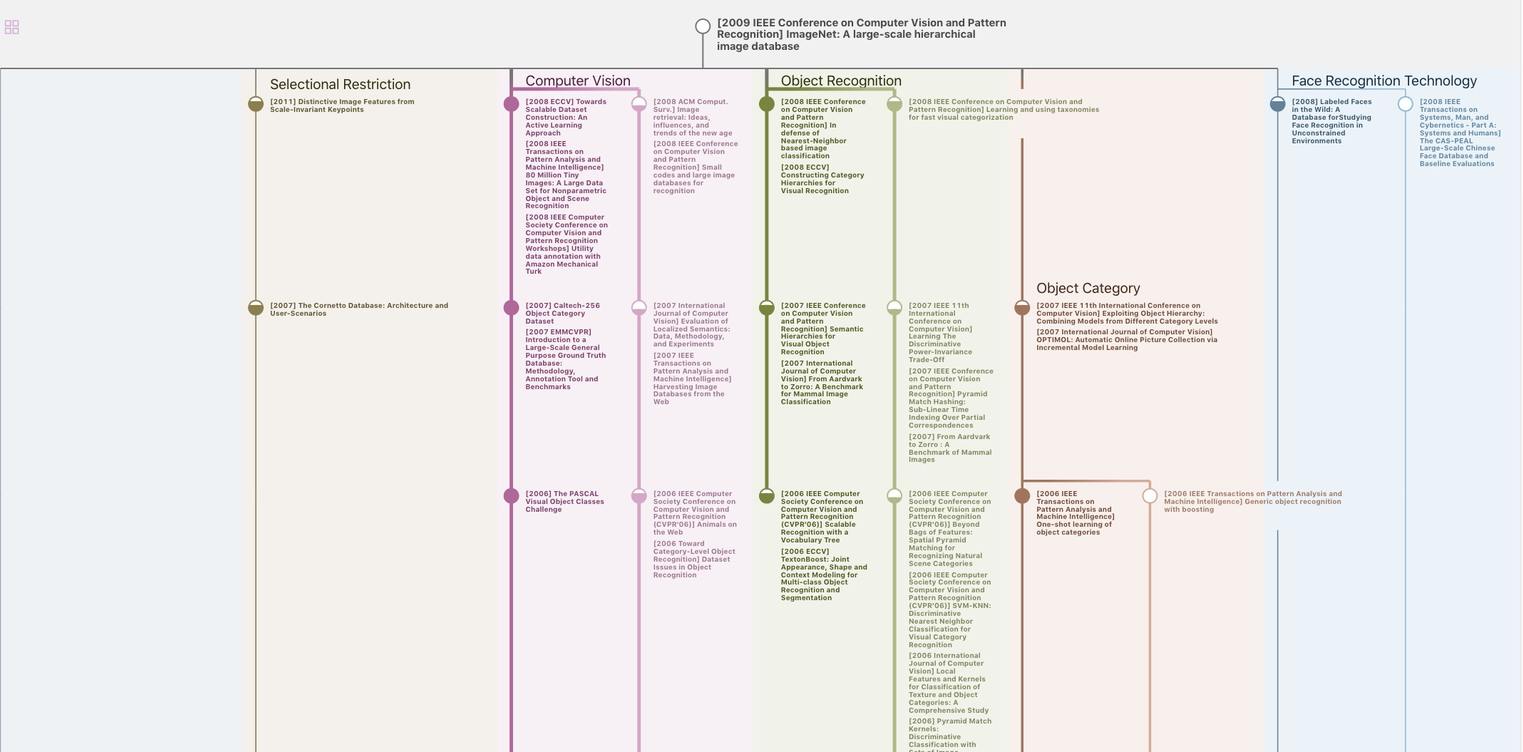
生成溯源树,研究论文发展脉络
Chat Paper
正在生成论文摘要