Open Set Intrusion Recognition for Fine-Grained Attack Categorization
2017 IEEE INTERNATIONAL SYMPOSIUM ON TECHNOLOGIES FOR HOMELAND SECURITY (HST)(2017)
摘要
Confidently distinguishing a malicious intrusion over a network is an important challenge. Most intrusion detection system evaluations have been performed in a closed set protocol in which only classes seen during training are considered during classification. Thus far, there has been no realistic application in which novel types of behaviors unseen at training -- unknown classes as it were -- must be recognized for manual categorization. This paper comparatively evaluates malware classification using both closed set and open set protocols for intrusion recognition on the KDDCUP'99 dataset. In contrast to much of the previous work, we employ a fine-grained recognition protocol, in which the dataset is loosely open set -- i.e., recognizing individual intrusion types -- e.g., "sendmail", "snmp guess", ..., etc., rather than more general attack categories (e.g., "DoS","Probe","R2L","U2R","Normal"). We also employ two different classifier types -- Gaussian RBF kernel SVMs, which are not theoretically guaranteed to bound open space risk, and W-SVMs, which are theoretically guaranteed to bound open space risk. We find that the W-SVM offers superior performance under the open set regime, particularly as the cost of misclassifying unknown classes at query time (i.e., classes not present in the training set) increases. Results of performance tradeoff with respect to cost of unknown as well as discussion of the ramifications of these findings in an operational setting are presented.
更多查看译文
关键词
open set intrusion recognition,fine-grained attack categorization,intrusion detection system,malware classification,closed set protocols,open set protocols,Gaussian RBF kernel SVM
AI 理解论文
溯源树
样例
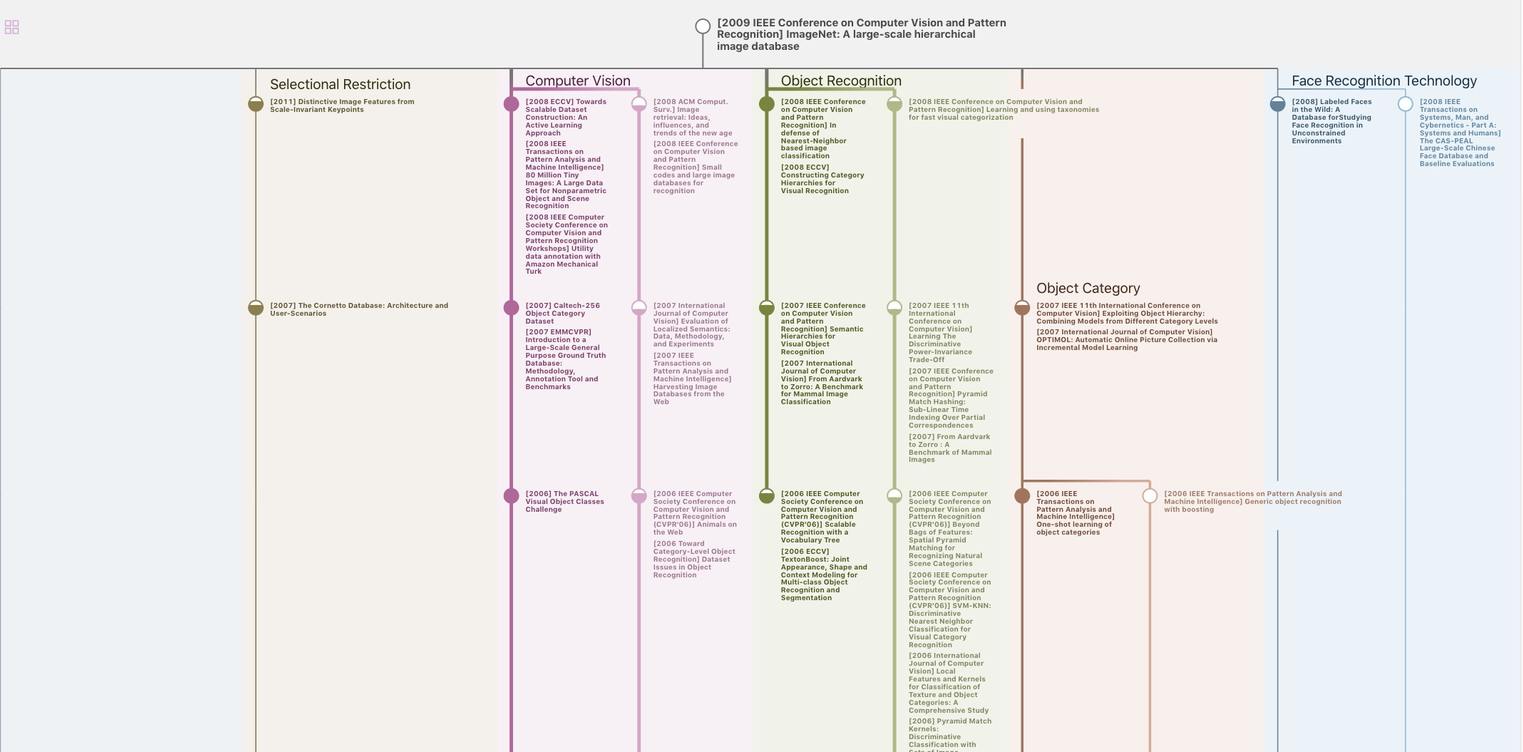
生成溯源树,研究论文发展脉络
Chat Paper
正在生成论文摘要