Convolutional Recurrent Neural Networks For Small-Footprint Keyword Spotting
18TH ANNUAL CONFERENCE OF THE INTERNATIONAL SPEECH COMMUNICATION ASSOCIATION (INTERSPEECH 2017), VOLS 1-6: SITUATED INTERACTION(2017)
摘要
Keyword spotting (KWS) constitutes a major component of human-technology interfaces. Maximizing the detection accuracy at a low false alarm (FA) rate, while minimizing the footprint size, latency and complexity are the goals for KWS. Towards achieving them, we study Convolutional Recurrent Neural Networks (CRNNs). Inspired by large-scale state-of-the-art speech recognition systems, we combine the strengths of convolutional layers and recurrent layers to exploit local structure and long-range context. We analyze the effect of architecture parameters, and propose training strategies to improve performance. With only similar to 230k parameters, our CRNN model yields acceptably low latency, and achieves 97.71% accuracy at 0.5 FA/hour for 5 dB signal-to-noise ratio.
更多查看译文
关键词
Keyword spotting, speech recognition, convolutional neural networks, recurrent neural networks
AI 理解论文
溯源树
样例
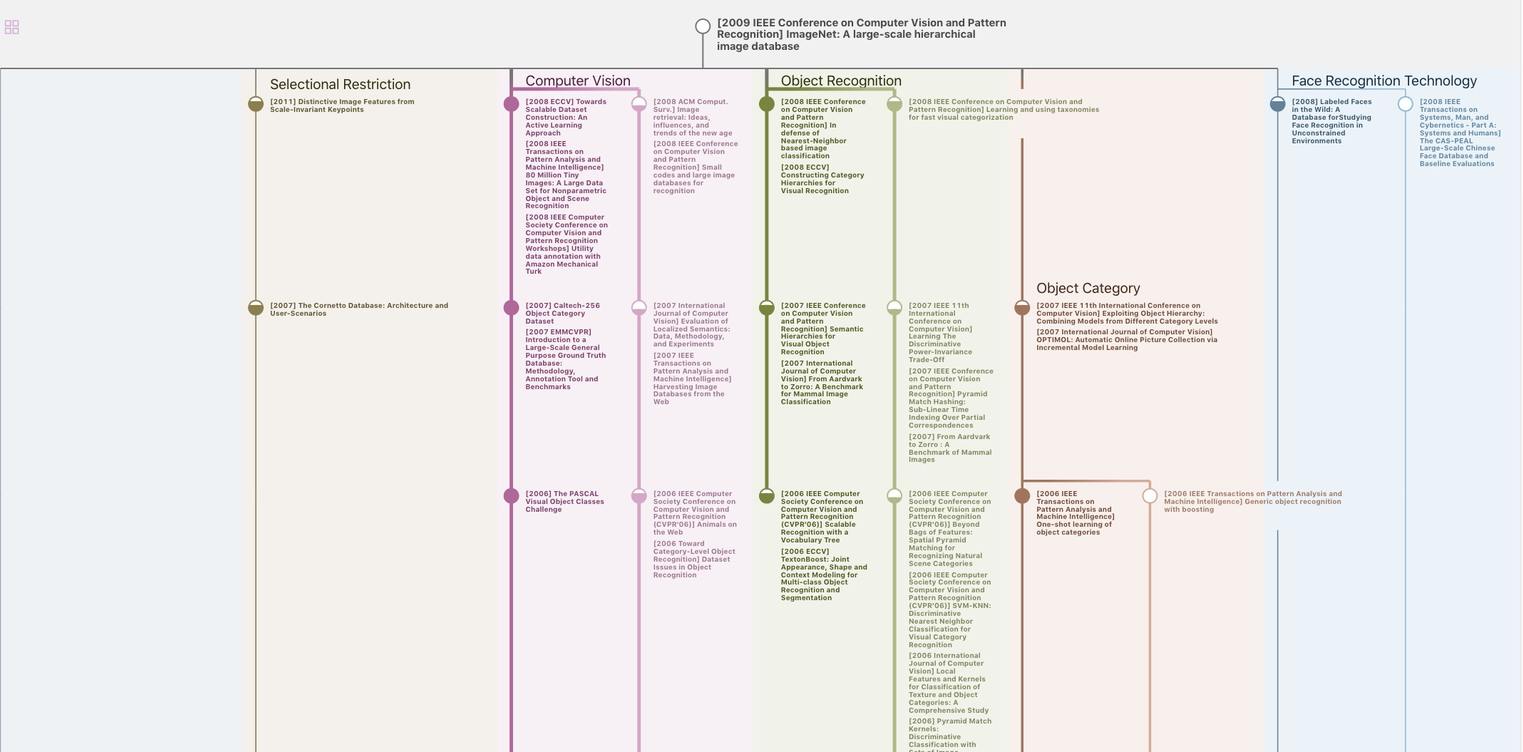
生成溯源树,研究论文发展脉络
Chat Paper
正在生成论文摘要