Causal Embeddings for Recommendation.
RecSys '18: Twelfth ACM Conference on Recommender Systems Vancouver British Columbia Canada October, 2018(2018)
摘要
Many current applications use recommendations in order to modify the natural user behavior, such as to increase the number of sales or the time spent on a website. This results in a gap between the final recommendation objective and the classical setup where recommendation candidates are evaluated by their coherence with past user behavior, by predicting either the missing entries in the user-item matrix, or the most likely next event. To bridge this gap, we optimize a recommendation policy for the task of increasing the desired outcome versus the organic user behavior. We show this is equivalent to learning to predict recommendation outcomes under a fully random recommendation policy. To this end, we propose a new domain adaptation algorithm that learns from logged data containing outcomes from a biased recommendation policy and predicts recommendation outcomes according to random exposure. We compare our method against state-of-the-art factorization methods, in addition to new approaches of causal recommendation and show significant improvements.
更多查看译文
关键词
Recommender Systems, Causality, Embeddings, Neural Networks, Counterfactual Inference
AI 理解论文
溯源树
样例
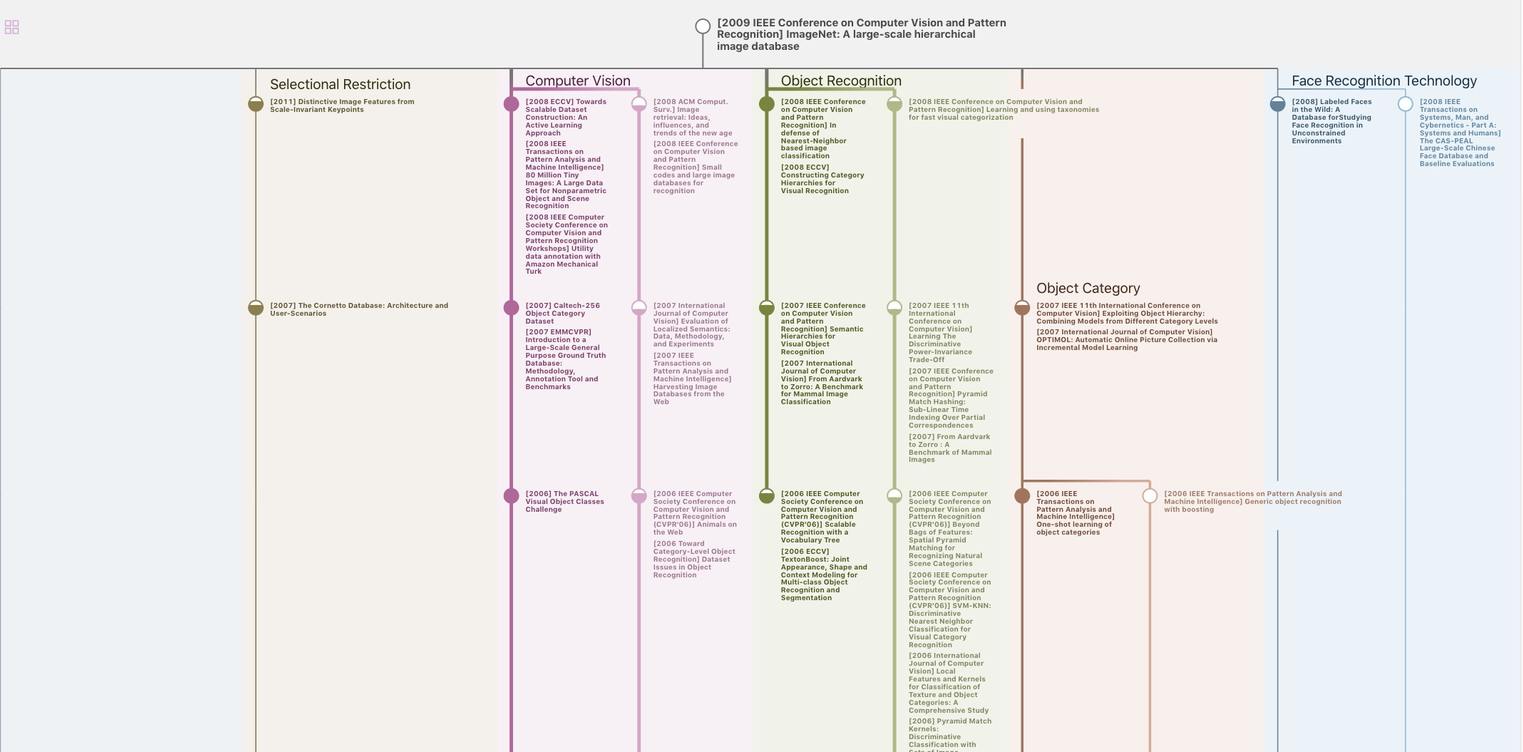
生成溯源树,研究论文发展脉络
Chat Paper
正在生成论文摘要