ToolNet: Holistically-Nested Real-Time Segmentation of Robotic Surgical Tools
2017 IEEE/RSJ International Conference on Intelligent Robots and Systems (IROS)(2017)
摘要
Real-time tool segmentation from endoscopic videos is an essential part of many computer-assisted robotic surgical systems and of critical importance in robotic surgical data science. We propose two novel deep learning architectures for automatic segmentation of non-rigid surgical instruments. Both methods take advantage of automated deep-learning-based multi-scale feature extraction while trying to maintain an accurate segmentation quality at all resolutions. The two proposed methods encode the multi-scale constraint inside the network architecture. The first proposed architecture enforces it by cascaded aggregation of predictions and the second proposed network does it by means of a holistically-nested architecture where the loss at each scale is taken into account for the optimization process. As the proposed methods are for real-time semantic labeling, both present a reduced number of parameters. We propose the use of parametric rectified linear units for semantic labeling in these small architectures to increase the regularization ability of the design and maintain the segmentation accuracy without overfitting the training sets. We compare the proposed architectures against state-of-the-art fully convolutional networks. We validate our methods using existing benchmark datasets, including ex vivo cases with phantom tissue and different robotic surgical instruments present in the scene. Our results show a statistically significant improved Dice Similarity Coefficient over previous instrument segmentation methods. We analyze our design choices and discuss the key drivers for improving accuracy.
更多查看译文
关键词
optimization process,fully convolutional networks,ToolNet,robotic surgical tools,robotic surgical systems,robotic surgical data science,deep learning architectures,robotic surgical instruments,automatic image segmentation,instrument segmentation methods,endoscopic videos segmentation,automated deep-learning-based multiscale feature extraction,phantom tissue,dice similarity coefficient
AI 理解论文
溯源树
样例
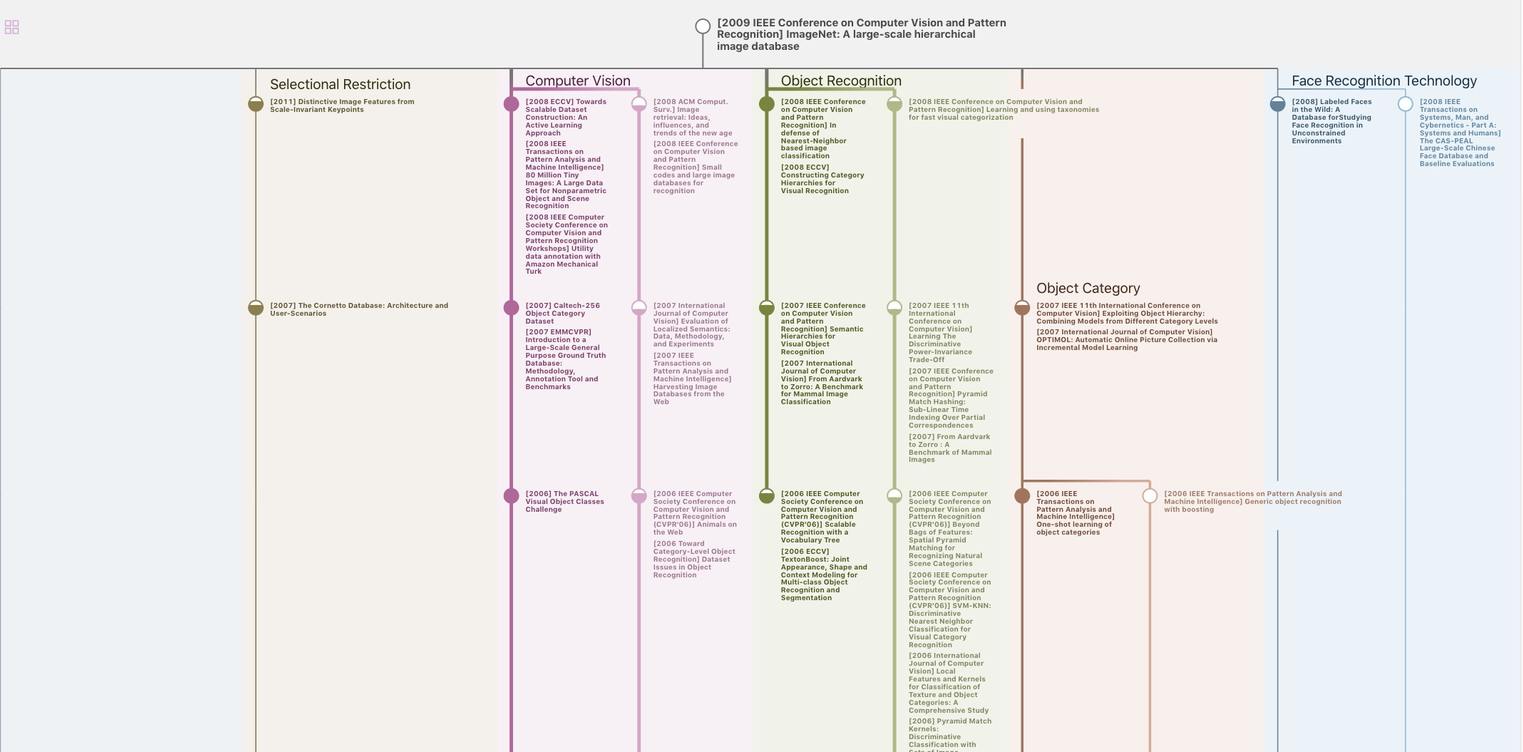
生成溯源树,研究论文发展脉络
Chat Paper
正在生成论文摘要