Segmentation of Intracranial Arterial Calcification with Deeply Supervised Residual Dropout Networks.
MICCAI(2017)
摘要
Intracranial carotid artery calcification (ICAC) is a major risk factor for stroke, and might contribute to dementia and cognitive decline. Reliance on time-consuming manual annotation of ICAC hampers much demanded further research into the relationship between ICAC and neurological diseases. Automation of ICAC segmentation is therefore highly desirable, but difficult due to the proximity of the lesions to bony structures with a similar attenuation coefficient. In this paper, we propose a method for automatic segmentation of ICAC; the first to our knowledge. Our method is based on a 3D fully convolutional neural network that we extend with two regularization techniques. Firstly, we use deep supervision to encourage discriminative features in the hidden layers. Secondly, we augment the network with skip connections, as in the recently developed ResNet, and dropout layers, inserted in a way that skip connections circumvent them. We investigate the effect of skip connections and dropout. In addition, we propose a simple problem-specific modification of the network objective function that restricts the focus to the most important image regions and simplifies the optimization. We train and validate our model using 882 CT scans and test on 1,000. Our regularization techniques and objective improve the average Dice score by 7.1%, yielding an average Dice of 76.2% and 97.7% correlation between predicted ICAC volumes and manual annotations.
更多查看译文
关键词
Intracranial calcifications, Calcium scoring, Deep learning, Deep supervision, Residual networks, Dropout
AI 理解论文
溯源树
样例
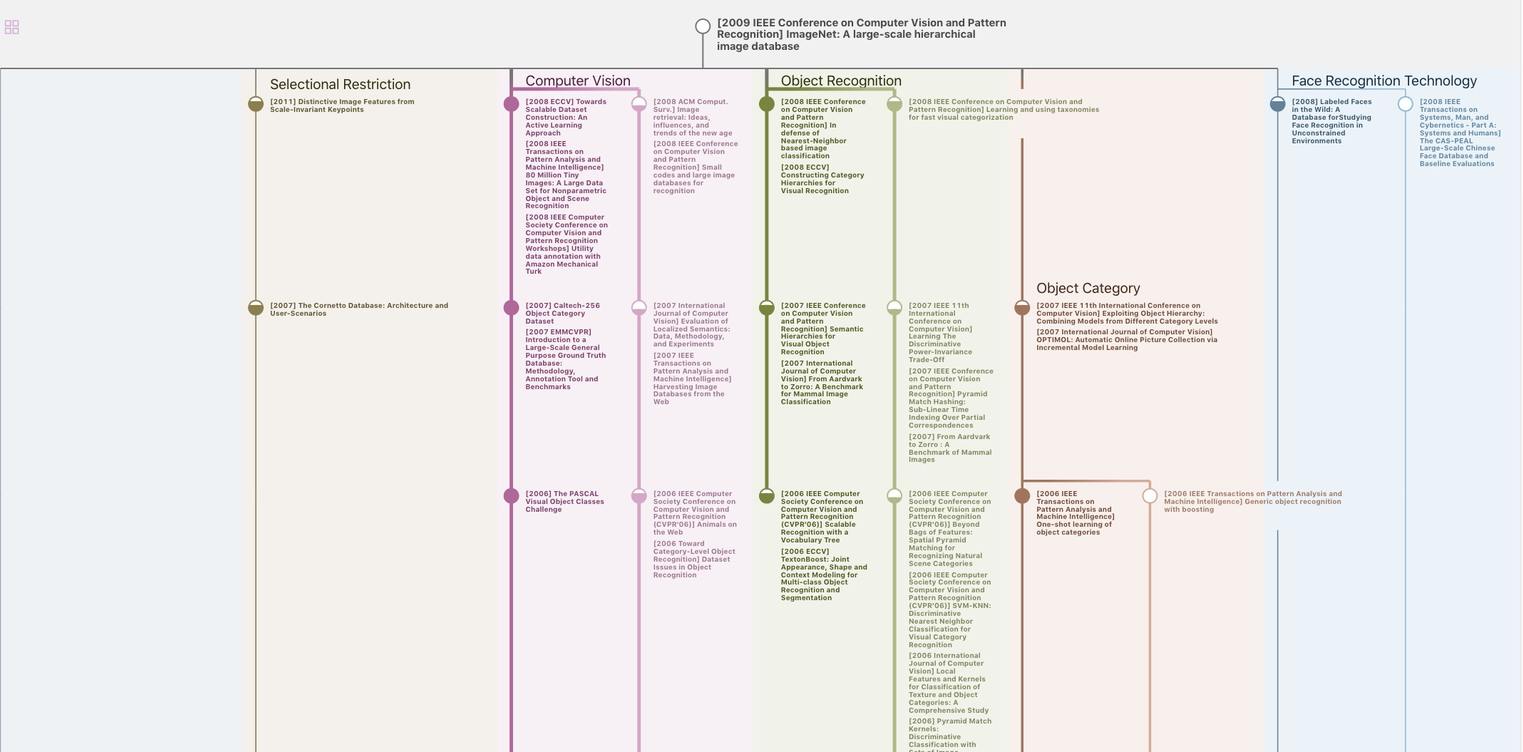
生成溯源树,研究论文发展脉络
Chat Paper
正在生成论文摘要