Learning Spatiotemporal Features for Infrared Action Recognition with 3D Convolutional Neural Networks
2017 IEEE Conference on Computer Vision and Pattern Recognition Workshops (CVPRW)(2017)
摘要
Infrared (IR) imaging has the potential to enable more robust action recognition systems compared to visible spectrum cameras due to lower sensitivity to lighting conditions and appearance variability. While the action recognition task on videos collected from visible spectrum imaging has received much attention, action recognition in infrared (IR) videos is significantly less explored. Our objective is to exploit imaging data in this modality for the action recognition task. In this work, we propose a novel two-stream 3D convolutional neural network architecture by introducing the discriminative code layer and the corresponding discriminative code loss function. The proposed network processes IR images and the IR-based optical flow field sequences. We pretrain the 3D CNN model on the visible spectrum Sports-1M action dataset and finetune it on the Infrared Action Recognition (InfAR) dataset. We conduct an elaborate analysis of different fusion schemes (weighted average, single and double-layer neural nets) applied to different 3D CNN outputs. Experimental results demonstrate that our approach can achieve state-of-the-art average precision performances on the InfAR dataset.
更多查看译文
关键词
infrared imaging,optical flow fields,two-stream 3D CNN model,3D CNN outputs,fusion schemes,IR domain,InfAR dataset,infrared action recognition dataset,Sports-1M action dataset,visible spectrum,IR-based optical flow field sequences,IR image,discriminative code loss function,discriminative code layer,two-stream 3D convolutional neural network architecture,IR videos,visible spectrum imaging,appearance variability,lighting conditions,robust action recognition systems
AI 理解论文
溯源树
样例
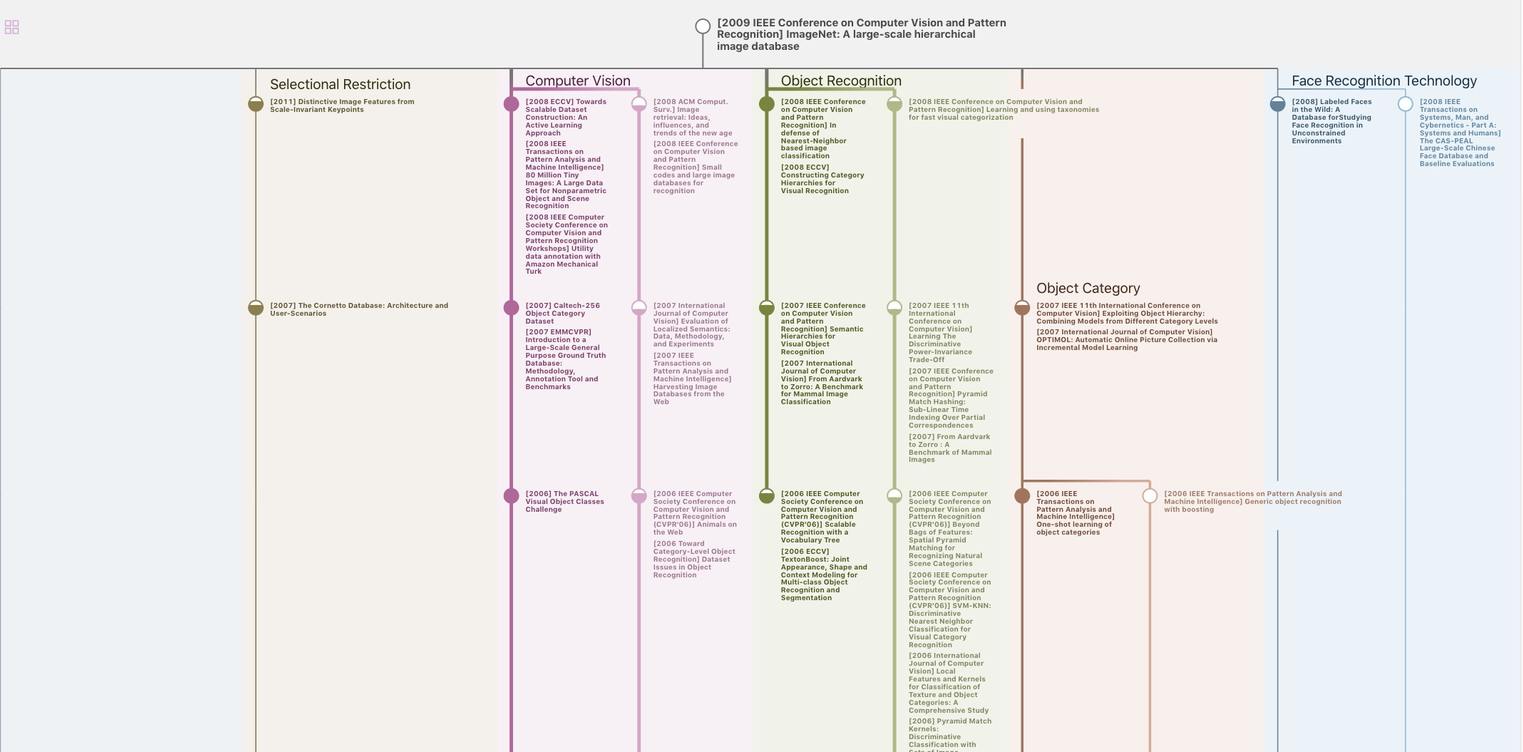
生成溯源树,研究论文发展脉络
Chat Paper
正在生成论文摘要