Community Detection and Stochastic Block Models
Foundations and Trends in Communications and Information Theory(2022)
摘要
The stochastic block model (SBM) is a random graph model with different group of vertices connecting differently. It is widely employed as a canonical model to study clustering and community detection, and provides a fertile ground to study the information-theoretic and computational tradeoffs that arise in combinatorial statistics and more generally data science. This monograph surveys the recent developments that establish the fundamental limits for community detection in the SBM, both with respect to information-theoretic and computational tradeoffs, and for various recovery requirements such as exact, partial and weak recovery. The main results discussed are the phase transitions for exact recovery at the Chernoff-Hellinger threshold, the phase transition for weak recovery at the Kesten-Stigum threshold, the optimal SNR-mutual information tradeoff for partial recovery, and the gap between information-theoretic and computational thresholds. The monograph gives a principled derivation of the main algorithms developed in the quest of achieving the limits, in particular two-round algorithms via graph-splitting, semi-definite programming, (linearized) belief propagation, classical/nonbacktracking spectral methods and graph powering. Extensions to other block models, such as geometric block models, and a few open problems are also discussed.
更多查看译文
关键词
Community detection,clustering,stochastic block models,random graphs,unsupervised learning,spectral algorithms,computational gaps,network data analysis
AI 理解论文
溯源树
样例
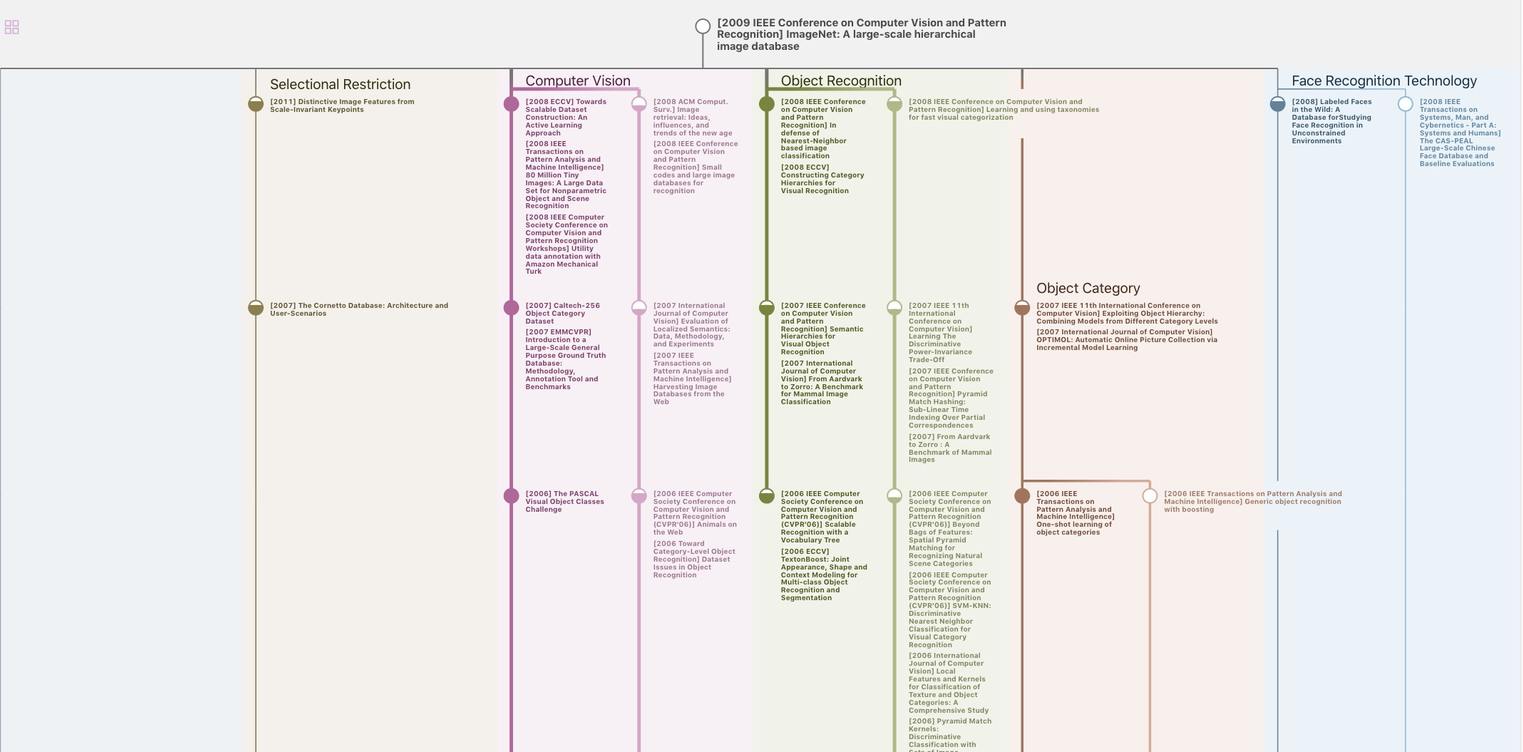
生成溯源树,研究论文发展脉络
Chat Paper
正在生成论文摘要