Unsupervised learning of object landmarks by factorized spatial embeddings
2017 IEEE International Conference on Computer Vision (ICCV)(2017)
摘要
Learning automatically the structure of object categories remains an important open problem in computer vision. In this paper, we propose a novel unsupervised approach that can discover and learn landmarks in object categories, thus characterizing their structure. Our approach is based on factorizing image deformations, as induced by a viewpoint change or an object deformation, by learning a deep neural network that detects landmarks consistently with such visual effects. Furthermore, we show that the learned landmarks establish meaningful correspondences between different object instances in a category without having to impose this requirement explicitly. We assess the method qualitatively on a variety of object types, natural and man-made. We also show that our unsupervised landmarks are highly predictive of manually-annotated landmarks in face benchmark datasets, and can be used to regress these with a high degree of accuracy.
更多查看译文
关键词
manually-annotated landmarks,unsupervised learning,object landmarks,factorized spatial embeddings,object categories,computer vision,unsupervised approach,image deformations,viewpoint change,object deformation,deep neural network,unsupervised landmarks,landmark detection
AI 理解论文
溯源树
样例
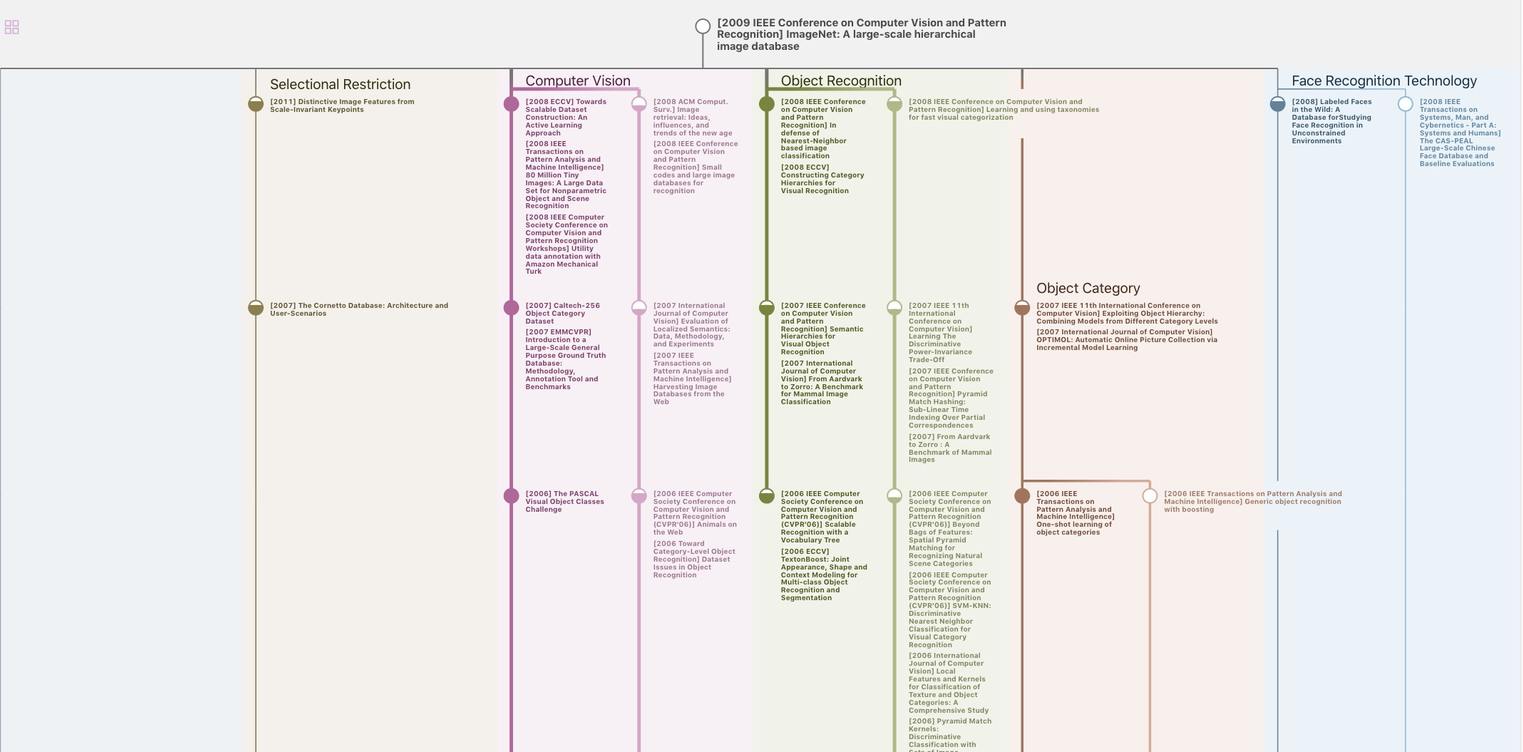
生成溯源树,研究论文发展脉络
Chat Paper
正在生成论文摘要