Effective Evaluation using Logged Bandit Feedback from Multiple Loggers
KDD '17: The 23rd ACM SIGKDD International Conference on Knowledge Discovery and Data Mining Halifax NS Canada August, 2017(2017)
摘要
Accurately evaluating new policies (e.g. ad-placement models, ranking functions, recommendation functions) is one of the key prerequisites for improving interactive systems. While the conventional approach to evaluation relies on online A/B tests, recent work has shown that counterfactual estimators can provide an inexpensive and fast alternative, since they can be applied offline using log data that was collected from a different policy fielded in the past. In this paper, we address the question of how to estimate the performance of a new target policy when we have log data from multiple historic policies. This question is of great relevance in practice, since policies get updated frequently in most online systems. We show that naively combining data from multiple logging policies can be highly suboptimal. In particular, we find that the standard Inverse Propensity Score (IPS) estimator suffers especially when logging and target policies diverge -- to a point where throwing away data improves the variance of the estimator. We therefore propose two alternative estimators which we characterize theoretically and compare experimentally. We find that the new estimators can provide substantially improved estimation accuracy.
更多查看译文
关键词
counterfactual estimators,log data,implicit feedback,off-policy evaluation
AI 理解论文
溯源树
样例
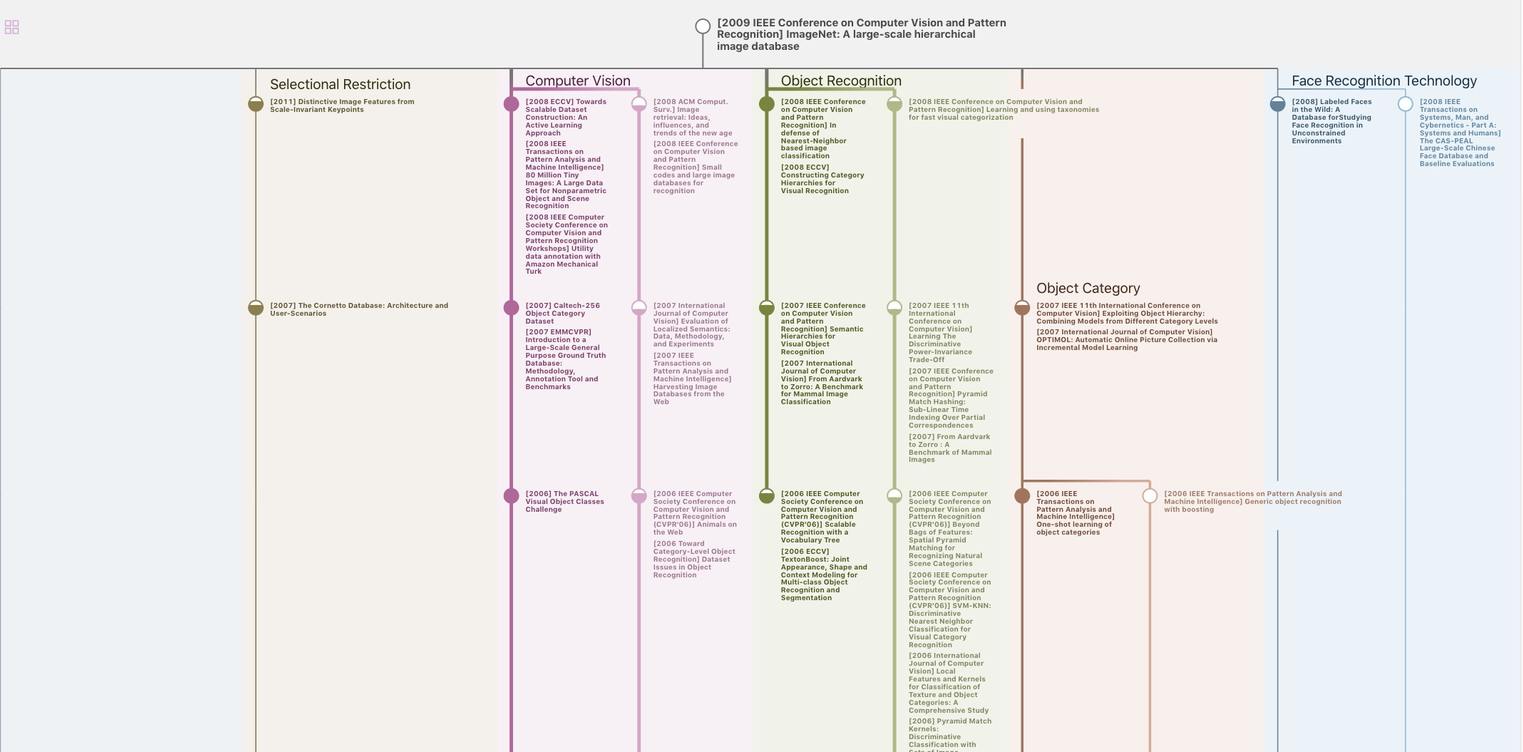
生成溯源树,研究论文发展脉络
Chat Paper
正在生成论文摘要