Maximum Selection and Ranking under Noisy Comparisons
ICML(2017)
摘要
We consider (ϵ,δ)-PAC maximum-selection and ranking for general probabilistic models whose comparisons probabilities satisfy strong stochastic transitivity and stochastic triangle inequality. Modifying the popular knockout tournament, we propose a maximum-selection algorithm that uses 𝒪(n/ϵ^2log1/δ) comparisons, a number tight up to a constant factor. We then derive a general framework that improves the performance of many ranking algorithms, and combine it with merge sort and binary search to obtain a ranking algorithm that uses 𝒪(nlog n (loglog n)^3/ϵ^2) comparisons for any δ≥1/n, a number optimal up to a (loglog n)^3 factor.
更多查看译文
AI 理解论文
溯源树
样例
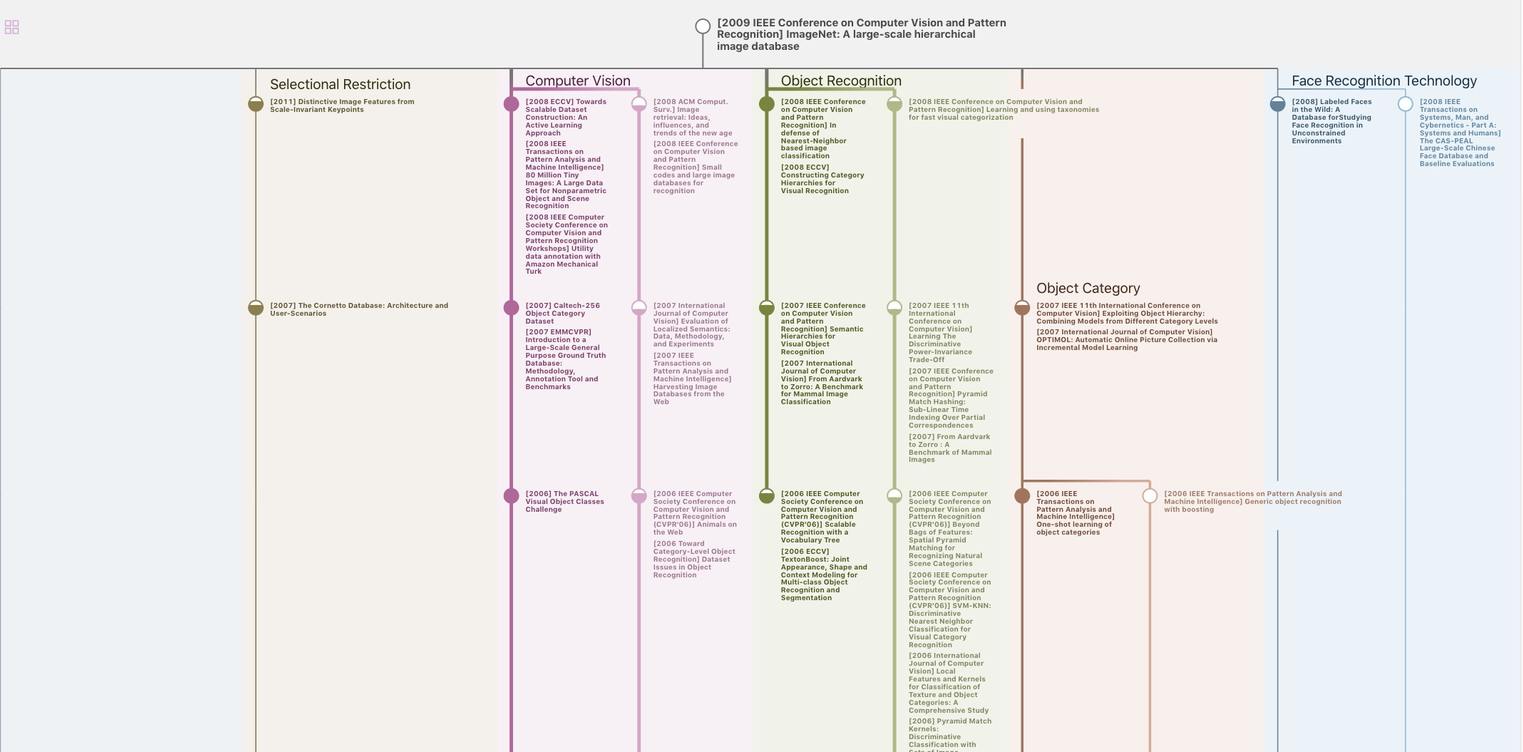
生成溯源树,研究论文发展脉络
Chat Paper
正在生成论文摘要