Unsupervised part learning for visual recognition
2017 IEEE Conference on Computer Vision and Pattern Recognition (CVPR)(2017)
摘要
Part-based image classification aims at representing categories by small sets of learned discriminative parts, upon which an image representation is built. Considered as a promising avenue a decade ago, this direction has been neglected since the advent of deep neural networks. In this context, this paper brings two contributions: first, it shows that despite the recent success of end-to-end holistic models, explicit part learning can boosts classification performance. Second, this work proceeds one step further than recent part-based models (PBM), focusing on how to learn parts without using any labeled data. Instead of learning a set of parts per class, as generally done in the PBM literature, the proposed approach both constructs a partition of a given set of images into visually similar groups, and subsequently learn a set of discriminative parts per group in a fully unsupervised fashion. This strategy opens the door to the use of PBM in new applications for which the notion of image categories is irrelevant, such as instance-based image retrieval, for example. We experimentally show that our learned parts can help building efficient image representations, for classification as well as for indexing tasks, resulting in performance superior to holistic state-of-the art Deep Convolutional Neural Networks (DCNN) encoding.
更多查看译文
关键词
explicit part learning,visual recognition,image representation,deep neural networks,visually similar groups,unsupervised part learning,part-based image classification
AI 理解论文
溯源树
样例
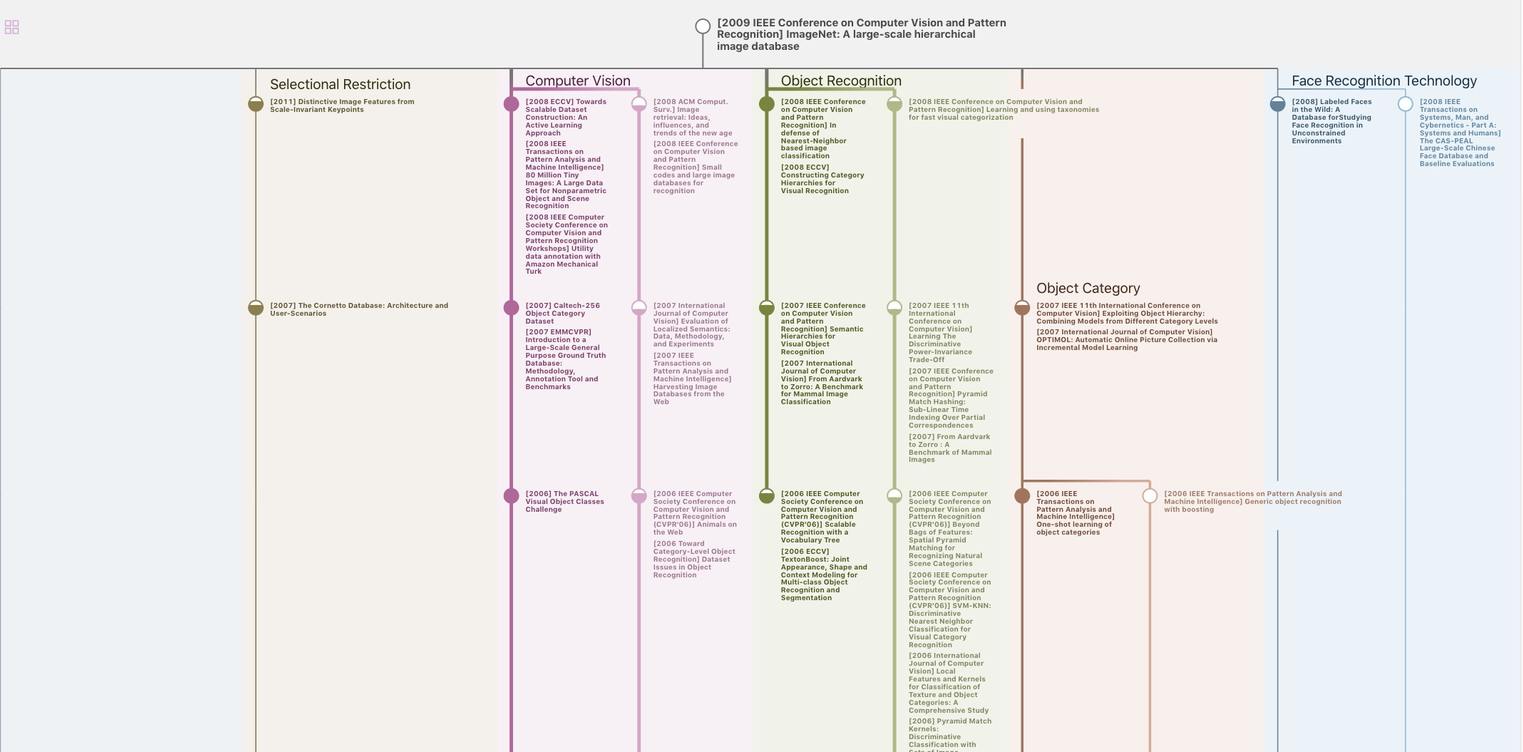
生成溯源树,研究论文发展脉络
Chat Paper
正在生成论文摘要