End-to-End Learning of Geometry and Context for Deep Stereo Regression
2017 IEEE International Conference on Computer Vision (ICCV)(2017)
摘要
We propose a novel deep learning architecture for regressing disparity from a rectified pair of stereo images. We leverage knowledge of the problem's geometry to form a cost volume using deep feature representations. We learn to incorporate contextual information using 3-D convolutions over this volume. Disparity values are regressed from the cost volume using a proposed differentiable soft argmin operation, which allows us to train our method end-to-end to sub-pixel accuracy without any additional post-processing or regularization. We evaluate our method on the Scene Flow and KITTI datasets and on KITTI we set a new state-of-the-art benchmark, while being significantly faster than competing approaches.
更多查看译文
关键词
disparity values,3-D convolutions,contextual information,deep feature representations,stereo images,deep learning architecture,deep stereo regression,additional post-processing,method end-to-end,differentiable soft argmin operation
AI 理解论文
溯源树
样例
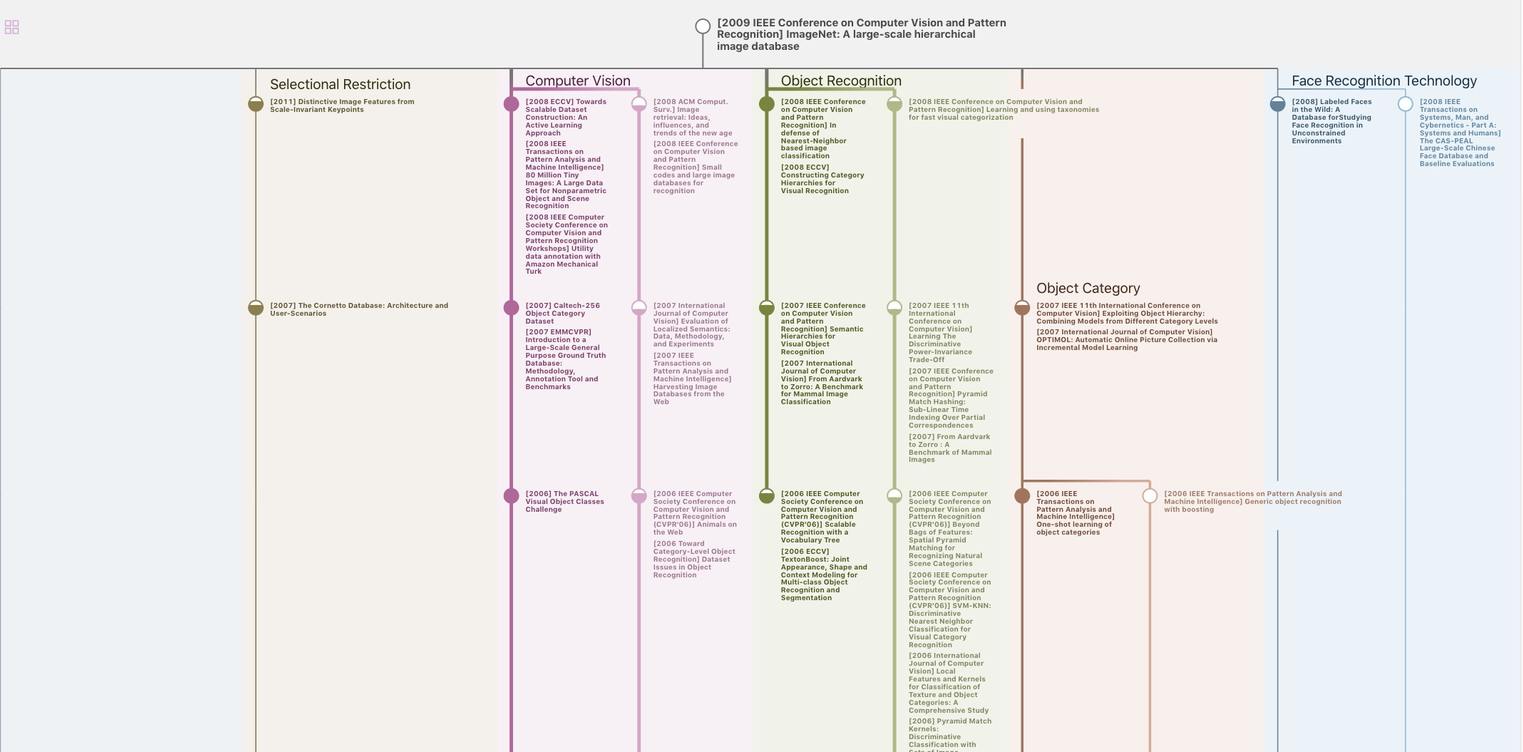
生成溯源树,研究论文发展脉络
Chat Paper
正在生成论文摘要