Distributed Partial Clustering
ACM Transactions on Parallel Computing(2017)
摘要
Recent years have witnessed an increasing popularity of algorithm design for distributed data, largely due to the fact that massive datasets are often collected and stored in different locations. In the distributed setting communication typically dominates the query processing time. Thus it becomes crucial to design communication efficient algorithms for queries on distributed data. Simultaneously, it has been widely recognized that partial optimizations, where we are allowed to disregard a small part of the data, provide us significantly better solutions. The motivation for disregarded points often arise from noise and other phenomena that are pervasive in large data scenarios. In this paper we focus on partial clustering problems, k-center, k-median and k-means, in the distributed model, and provide algorithms with communication sublinear of the input size. As a consequence we develop the first algorithms for the partial k-median and means objectives that run in subquadratic running time. We also initiate the study of distributed algorithms for clustering uncertain data, where each data point can possibly fall into multiple locations under certain probability distribution.
更多查看译文
关键词
Clustering,distributed computing,k-means,k-medians,k-centers
AI 理解论文
溯源树
样例
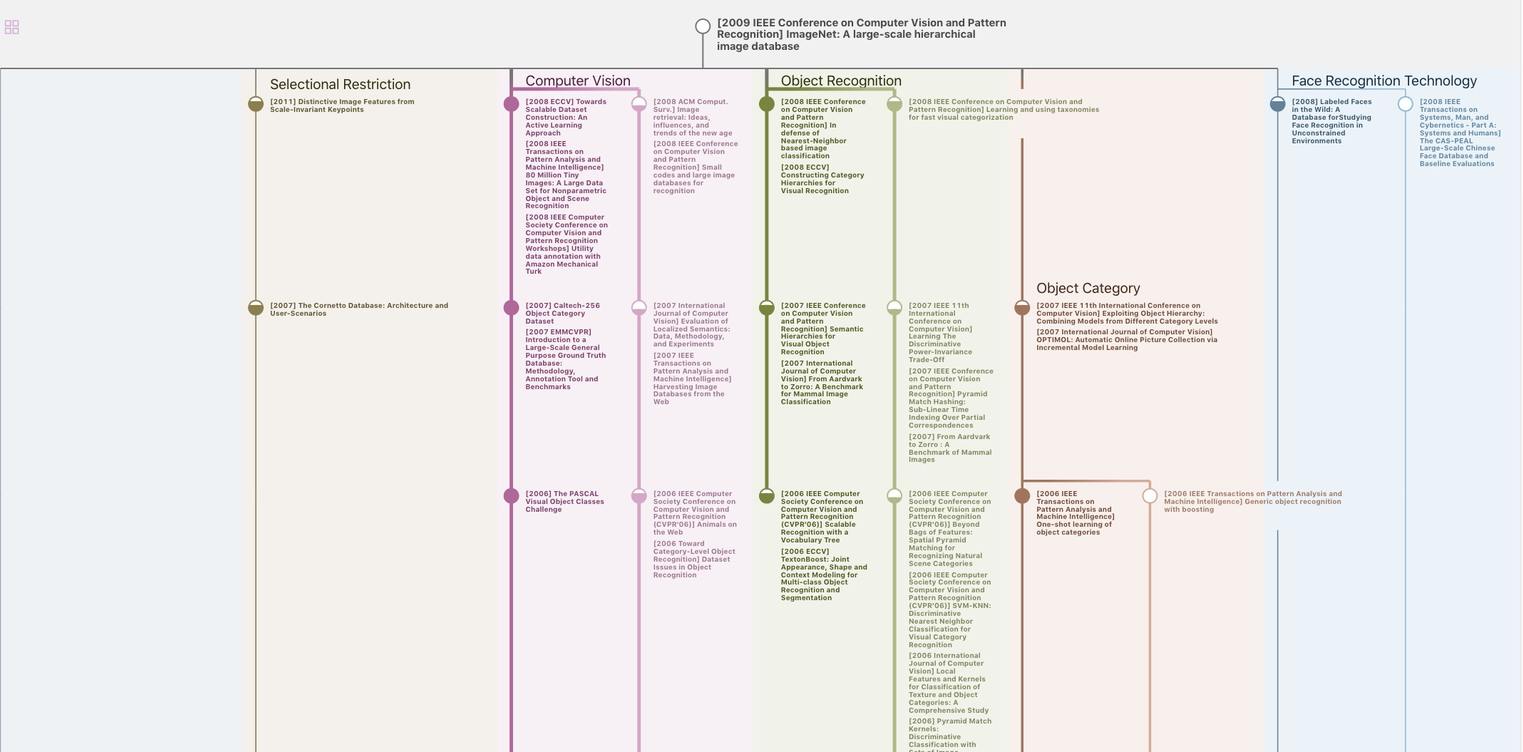
生成溯源树,研究论文发展脉络
Chat Paper
正在生成论文摘要