A Framework For Scalable Real-Time Anomaly Detection Over Voluminous, Geospatial Data Streams
Concurrency and Computation: Practice and Experience(2017)
摘要
This study presents a framework to enable distributed detection, storage, and analysis of anomalies in voluminous data streams. Individual observations within these streams are multidimensional, with each dimension corresponding to a feature of interest. We consider time-series geospatial datasets generated by remote and in situ observational devices. Three aspects make this problem particularly challenging: (1) the cumulative volume and rates of data arrivals, (2) evolution of the datasets over time, and (3) spatiotemporal correlations associated with the data. Further, solutions must minimize user intervention and be amenable to distributed processing to ensure scalability. Our approach achieves accurate, high-throughput classifications in real time, which we demonstrate with our reference anomaly detector implementations. We also provide interfaces that allow new implementations to be developed and parallelized automatically, ensuring applicability across problem domains. To help quantify the magnitude of anomalous observations, detector implementations provide a corresponding degree of irregularity. We have incorporated these algorithms into our distributed storage platform, Galileo, and profiled their suitability through empirical analysis that demonstrates high throughput (10000 observations per-second, per-node) on a real-worl Petabyte dataset.
更多查看译文
关键词
distributed geospatial anomaly detection,online anomaly detection,spatiotemporal data streams time series analytics
AI 理解论文
溯源树
样例
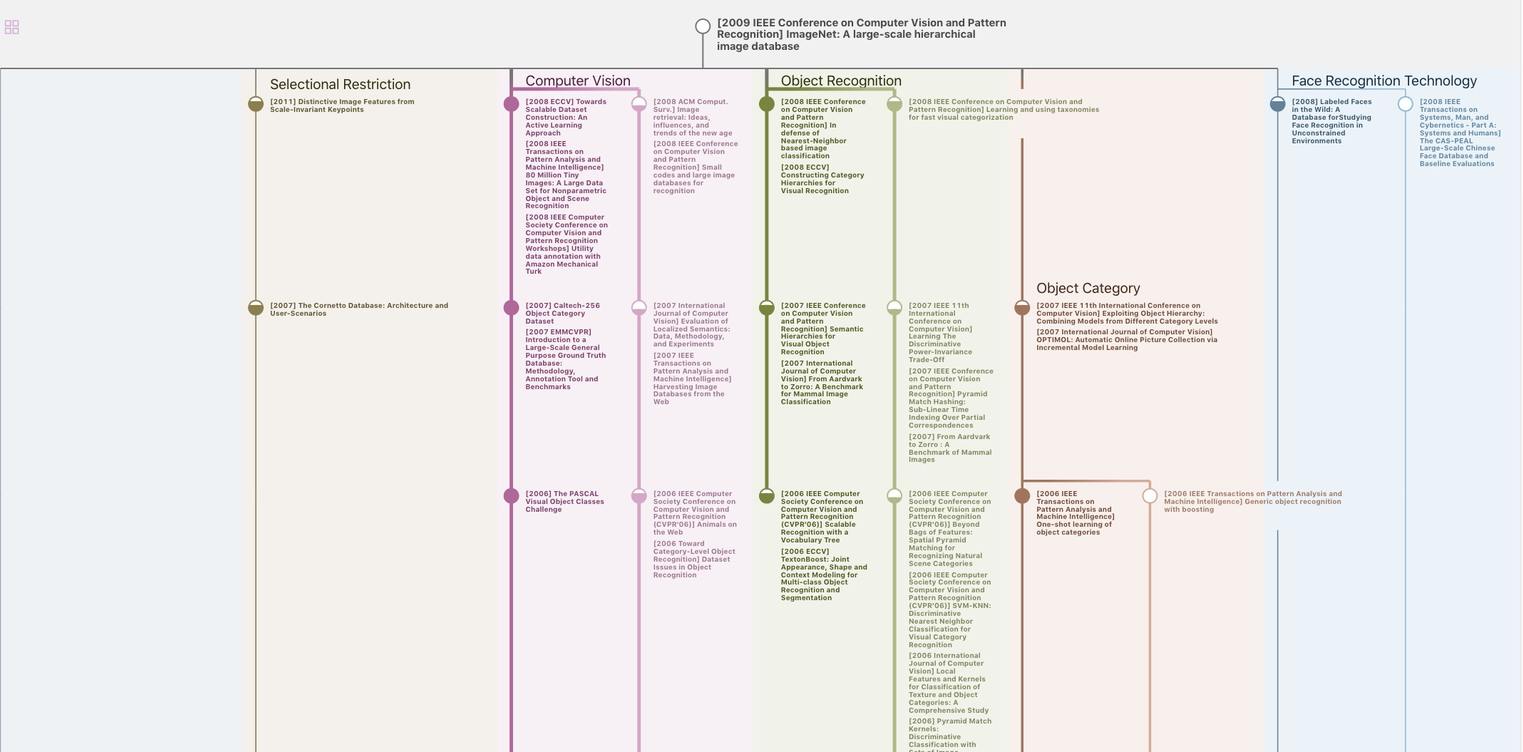
生成溯源树,研究论文发展脉络
Chat Paper
正在生成论文摘要