A combined model based on seasonal autoregressive integrated moving average and modified particle swarm optimization algorithm for electrical load forecasting.
JOURNAL OF INTELLIGENT & FUZZY SYSTEMS(2017)
摘要
Electric load prediction is an important decision tool in area of electricity economy. Recently researchers have presented innovative models to improve the forecasting accuracy of short-term electricity series, which is valuable in allowing both consumers and electric power sector to make effective planning. This study proposed novel combing optimization model to improve the precision of electric load forecasting and called SSPM. First, taken the advantage of linear prediction for the seasonal autoregressive integrated moving average (SARIMA) model and non-linear prediction for the support vector machines (SVM) model to combine a new model. Next, the produce results by SARIMA model is regarded as linear component and used SVM model for correcting the residual from SARIMA as non-linear component of forecasting results. Third, in order to show the dynamic relationship of linear and non-linear components, the weight variable of alpha(1) and alpha(2) are proposed that optimized by particle swarm optimization (PSO) algorithm with lower error of fitness function, the combining model is applied in the daily electric load data at New South Wales (NSW) in Australia. The experimental results indicate that the proposed optimization model obtains better performance of precise and stability than models of SARIMA and SVM respectively, outperform than conventional artificial neural network (ANN). Although the novel model is applied to electric load forecasting in this paper, it has more scopes for application in a number of areas to gain improvement of forecast accuracy in complex time series.
更多查看译文
关键词
Support vector machines,seasonal autoregressive integrated moving average,combining optimization model,electric load forecasting,particle swarm optimization algorithm
AI 理解论文
溯源树
样例
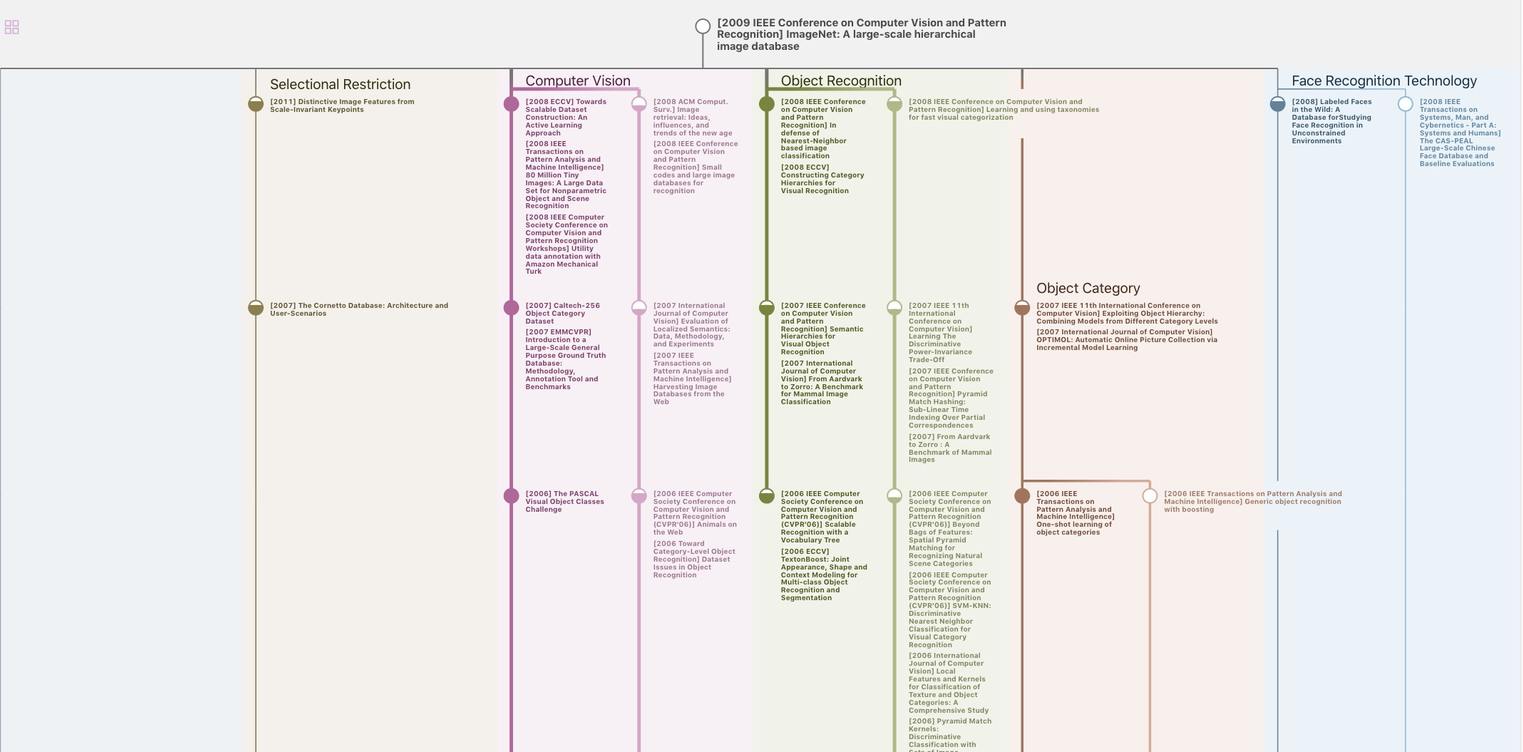
生成溯源树,研究论文发展脉络
Chat Paper
正在生成论文摘要