Coupling machine learning, tree-based and statistical models with cellular automata to simulate urban growth
Computers, Environment and Urban Systems(2017)
摘要
This paper compares six land use change (LUC) models, including artificial neural networks (ANNs), support vector regression (SVR), random forest (RF), classification and regression trees (CART), logistic regression (LR), and multivariate adaptive regression splines (MARS). These models were used to simulate urban growth in the megacity of Tehran Metropolitan Area (TMA). These LUC models were integrated with cellular automata (CA) and validated using a variety of goodness-of-fit metrics. The results showed that the percent correct metrics (PCMs) varied between 54.6% for LR and 59.6% for MARS, while the area under curve (AUC) ranged from 67.6% for LR to 74.7% for ANNs. The results also showed a considerable difference between the spatial patterns within the error maps. The results of this comparative study will enable decision makers and scholars to better understand the performance of the models when reducing the number of misses and false alarms is a priority.
更多查看译文
关键词
Machine learning models,Tree-based models,Statistical models,Cellular automata,Error map,Accuracy assessment
AI 理解论文
溯源树
样例
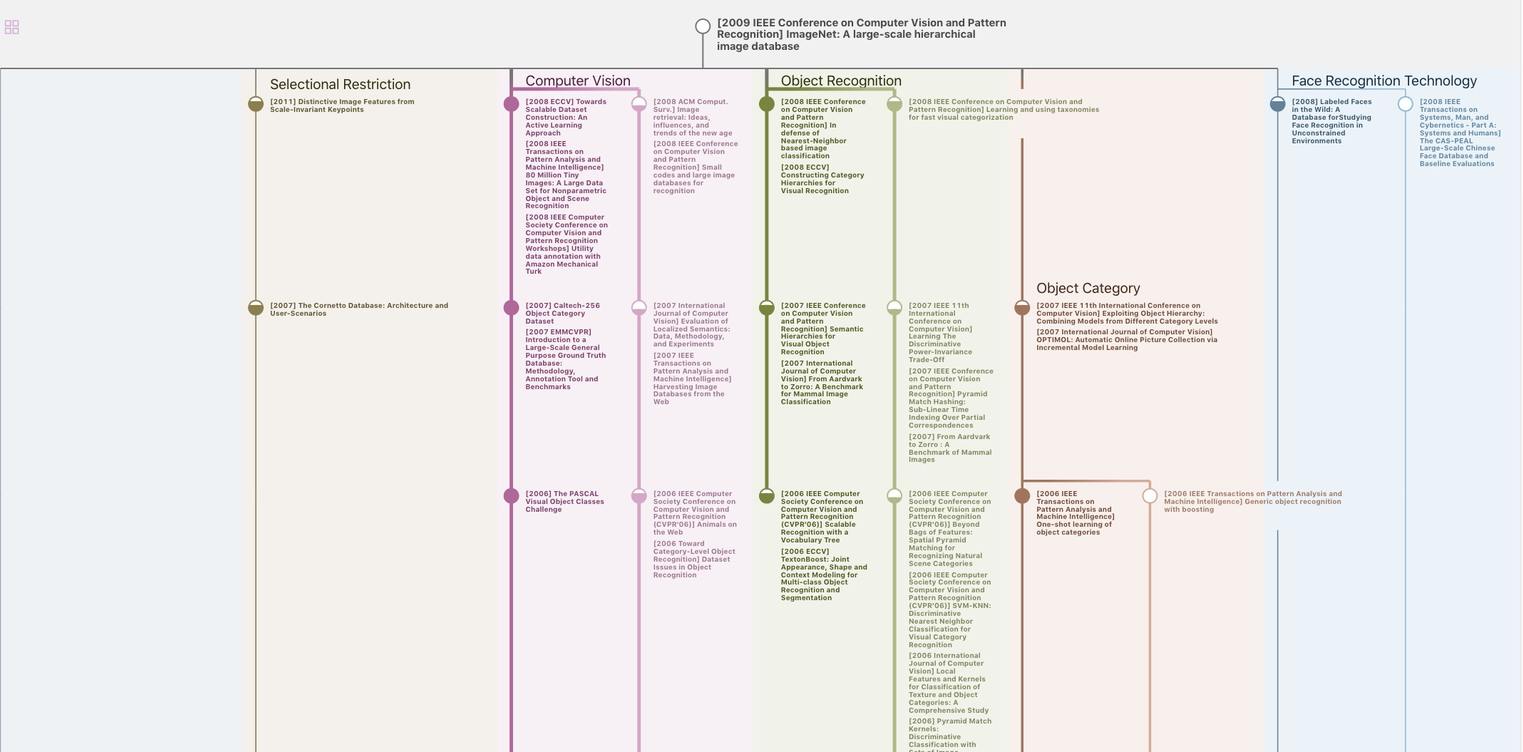
生成溯源树,研究论文发展脉络
Chat Paper
正在生成论文摘要