Temporally Consistent Depth Map Prediction Using Deep Convolutional Neural Network and Spatial-Temporal Conditional Random Field
Journal of Computer Science and Technology(2017)
摘要
Deep convolutional neural networks (DCNNs) based methods recently keep setting new records on the tasks of predicting depth maps from monocular images. When dealing with video-based applications such as 2D (2-dimensional) to 3D (3-dimensional) video conversion, however, these approaches tend to produce temporally inconsistent depth maps, since their CNN models are optimized over single frames. In this paper, we address this problem by introducing a novel spatial-temporal conditional random fields (CRF) model into the DCNN architecture, which is able to enforce temporal consistency between depth map estimations over consecutive video frames. In our approach, temporally consistent superpixel (TSP) is first applied to an image sequence to establish the correspondence of targets in consecutive frames. A DCNN is then used to regress the depth value of each temporal superpixel, followed by a spatial-temporal CRF layer to model the relationship of the estimated depths in both spatial and temporal domains. The parameters in both DCNN and CRF models are jointly optimized with back propagation. Experimental results show that our approach not only is able to significantly enhance the temporal consistency of estimated depth maps over existing single-frame-based approaches, but also improves the depth estimation accuracy in terms of various evaluation metrics.
更多查看译文
关键词
depth estimation,temporal consistency,convolutional neural network,conditional random fields
AI 理解论文
溯源树
样例
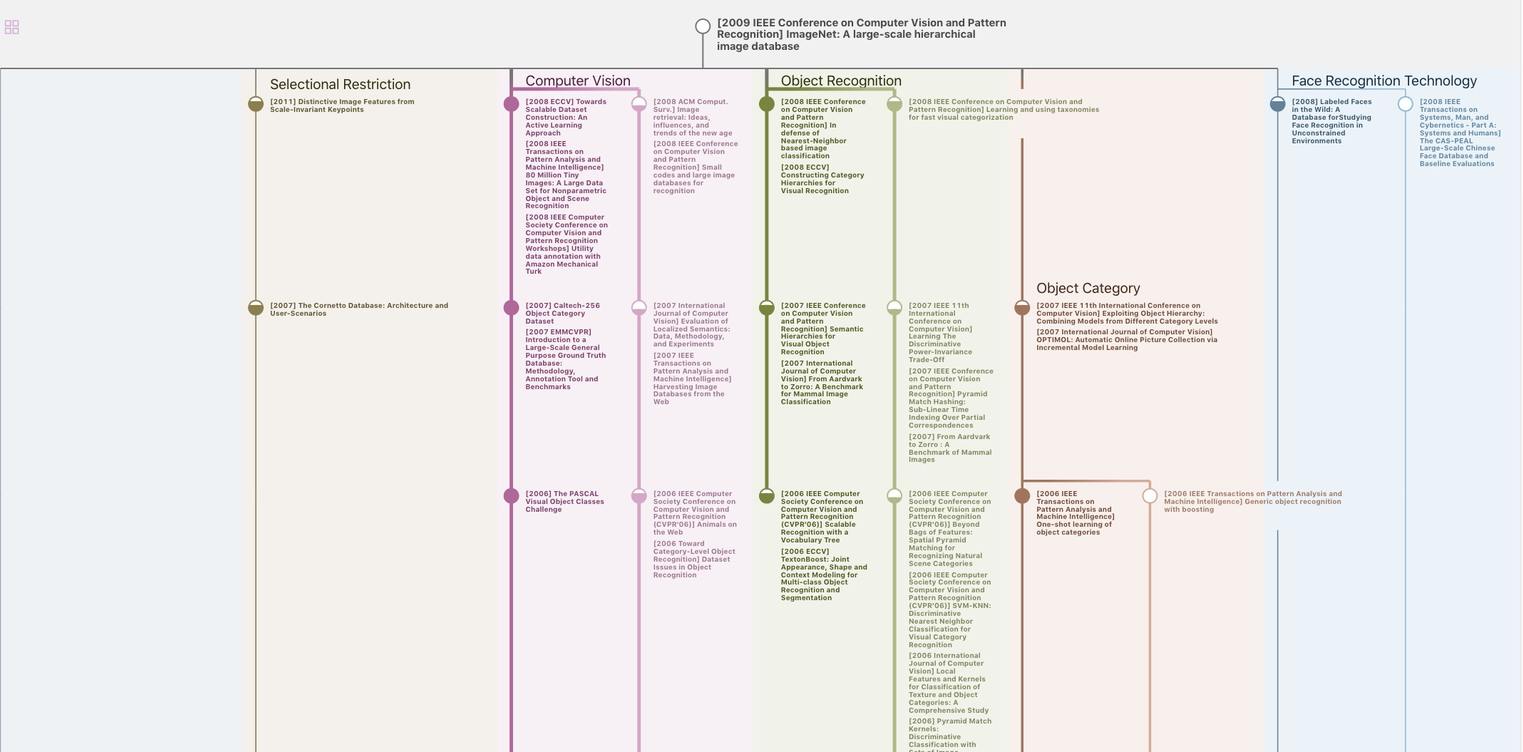
生成溯源树,研究论文发展脉络
Chat Paper
正在生成论文摘要