One-class classification of point patterns of extremes.
JOURNAL OF MACHINE LEARNING RESEARCH(2016)
摘要
Novelty detection or one-class classification starts from a model describing some type of 'normal behaviour' and aims to classify deviations from this model as being either novelties or anomalies. In this paper the problem of novelty detection for point patterns S = {X-1 ,..., X-k} subset of R-d is treated where examples of anomalies are very sparse, or even absent. The latter complicates the tuning of hyperparameters in models commonly used for novelty detection, such as one-class support vector machines and hidden Markov models. To this end, the use of extreme value statistics is introduced to estimate explicitly a model for the abnormal class by means of extrapolation from a statistical model X for the normal class. We show how multiple types of information obtained from any available extreme instances of S can be combined to reduce the high false-alarm rate that is typically encountered when classes are strongly imbalanced, as often occurs in the one-class setting (whereby 'abnormal' data are often scarce). The approach is illustrated using simulated data and then a real-life application is used as an exemplar, whereby accelerometry data from epileptic seizures are analysed - these are known to be extreme and rare with respect to normal accelerometer data.
更多查看译文
关键词
Sequence classification,novelty detection,extreme value theory,class imbalance,asymptotic theory
AI 理解论文
溯源树
样例
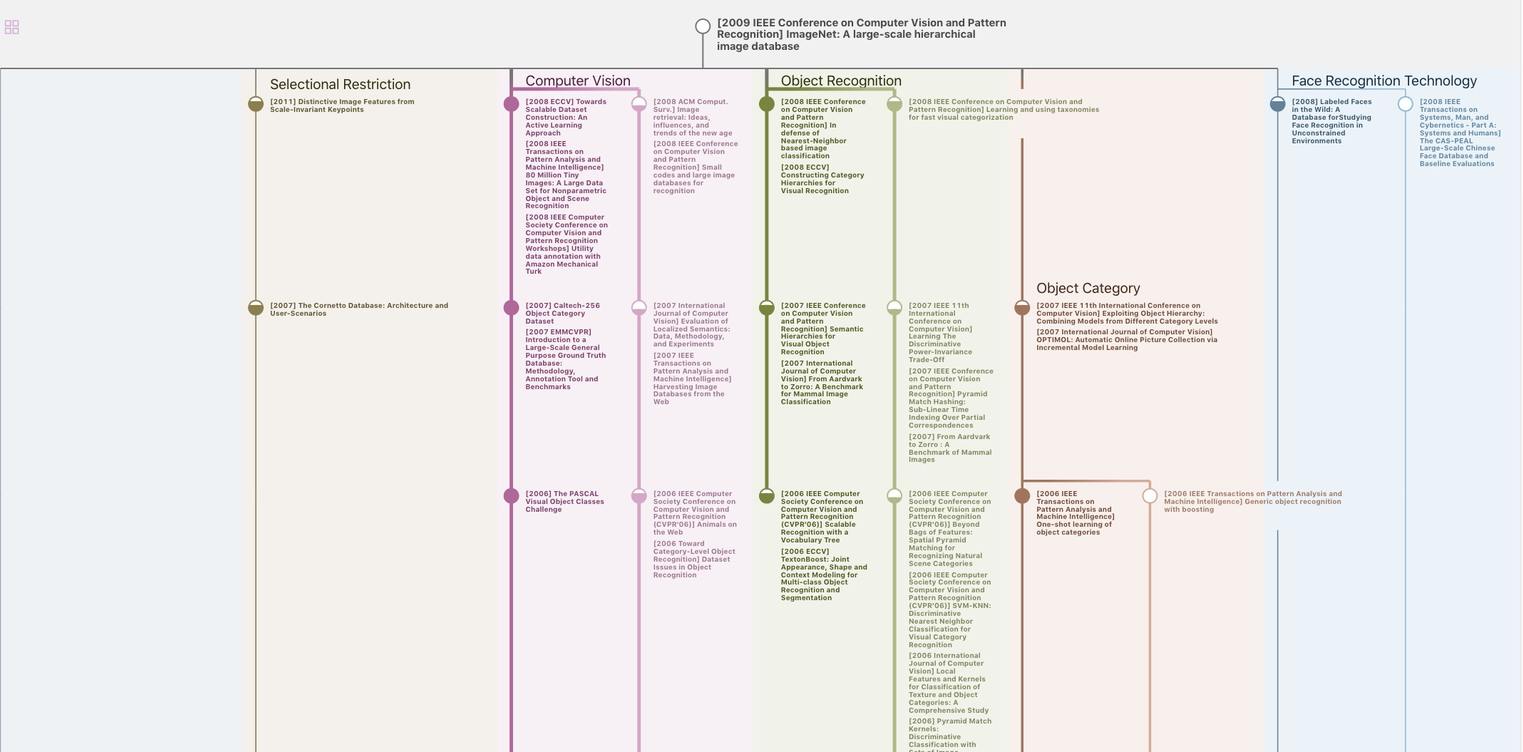
生成溯源树,研究论文发展脉络
Chat Paper
正在生成论文摘要