An RBF neural network approach to geometric error compensation with displacement measurements only
Neural Computing and Applications(2016)
摘要
A novel radial basis function (RBF) neural network-based geometric error compensation method with displacement measurements only is proposed in this paper. The individual geometric error components are formulated mathematically based on laser interferometer calibration with displacement measurements only and modeled using RBF neural network for error compensation in motion controller. Only 4 and 15 displacement measurements are required to identify the error components for XY and XYZ table, respectively. The experiment results on two XY tables illustrate the effectiveness of the proposed method. The overall errors can be reduced significantly after compensation, and different data intervals can be selected to reduce calibration time but maintain a high level of accuracy. The proposed methodology can be extended to other types of precision machine and is more suitable for precision machines requiring a relative low level of accuracy, but fast calibration like those used for acceptance testing and periodic checking.
更多查看译文
关键词
Radial basis function network, Error estimation, Error compensation, Precision machine
AI 理解论文
溯源树
样例
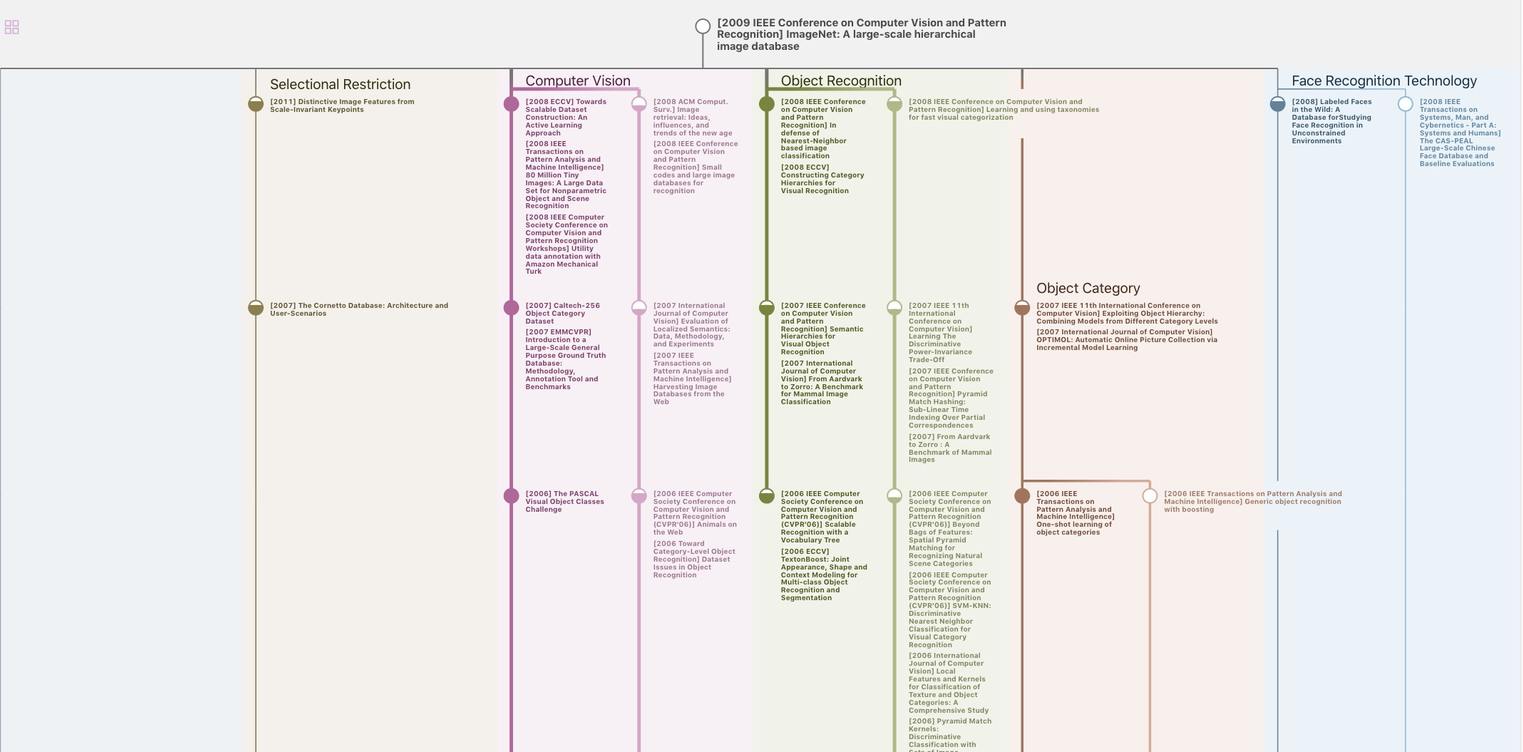
生成溯源树,研究论文发展脉络
Chat Paper
正在生成论文摘要