Constructing a meta-tracker using Dropout to imitate the behavior of an arbitrary black-box tracker.
Neural Networks(2017)
摘要
Imitating the behaviors of an arbitrary visual tracking algorithm enables many higher level tasks such as tracker identification and efficient tracker-fusion. It is also useful for discovering the features essential in a black-box tracker or learning from several trackers to form a super-tracker. In this study, we propose a non-linear feature fusion framework, “MIMIC” that imitates many popular trackers by mixing a pool of heterogeneous features. The MIMIC framework consists of two subtasks, feature selection and feature weight tuning. These subtasks, however, tended to suffer from an overfitting problem when the number of videos available for training is limited. To address this issue, we incorporated Dropout algorithm into the training, which grants the trained MIMIC tracker a high degree of generalization. Extensive experiments testified the effectiveness of the proposed framework so that its applications would be promoted into different related tasks in visual tracking.
更多查看译文
关键词
Dropout,Tracking,Features selection,Generalization
AI 理解论文
溯源树
样例
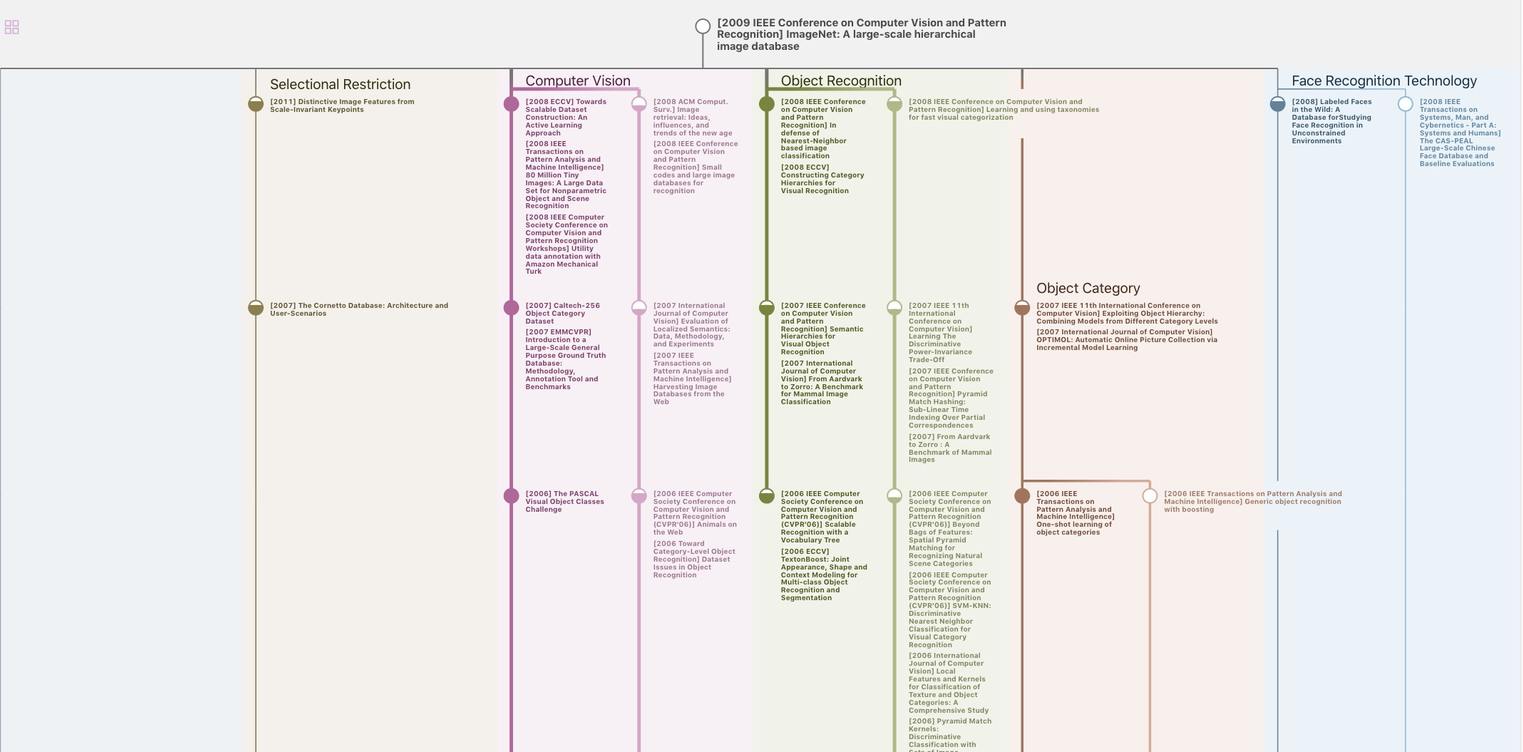
生成溯源树,研究论文发展脉络
Chat Paper
正在生成论文摘要