BiLoc: Bi-Modal Deep Learning for Indoor Localization With Commodity 5GHz WiFi.
IEEE ACCESS(2017)
摘要
In this paper, we study fingerprinting-based indoor localization in commodity 5-GHz WiFi networks. We first theoretically and experimentally validate three hypotheses on the channel state information (CSI) data of 5-GHz 01-DM channels. We then propose a system termed BiLoc, which uses bi-modality deep learning for localization in the indoor environment using off-the-shelf WiFi devices. We develop a deep learning-based algorithm to exploit bi-modal data, i.e., estimated angle of arrivings and average amplitudes (which are calibrated CSI data using several proposed techniques), for both the off-line and online stages of indoor fingerprinting. The proposed BiLoc system is implemented using commodity WiFi devices. Its superior performance is validated with extensive experiments under three typical indoor environments and through comparison with three benchmark schemes.
更多查看译文
关键词
Indoor localization,fingerprinting,deep learning,5GHz commodity WiFi,channel state information,bi-modality fingerprinting
AI 理解论文
溯源树
样例
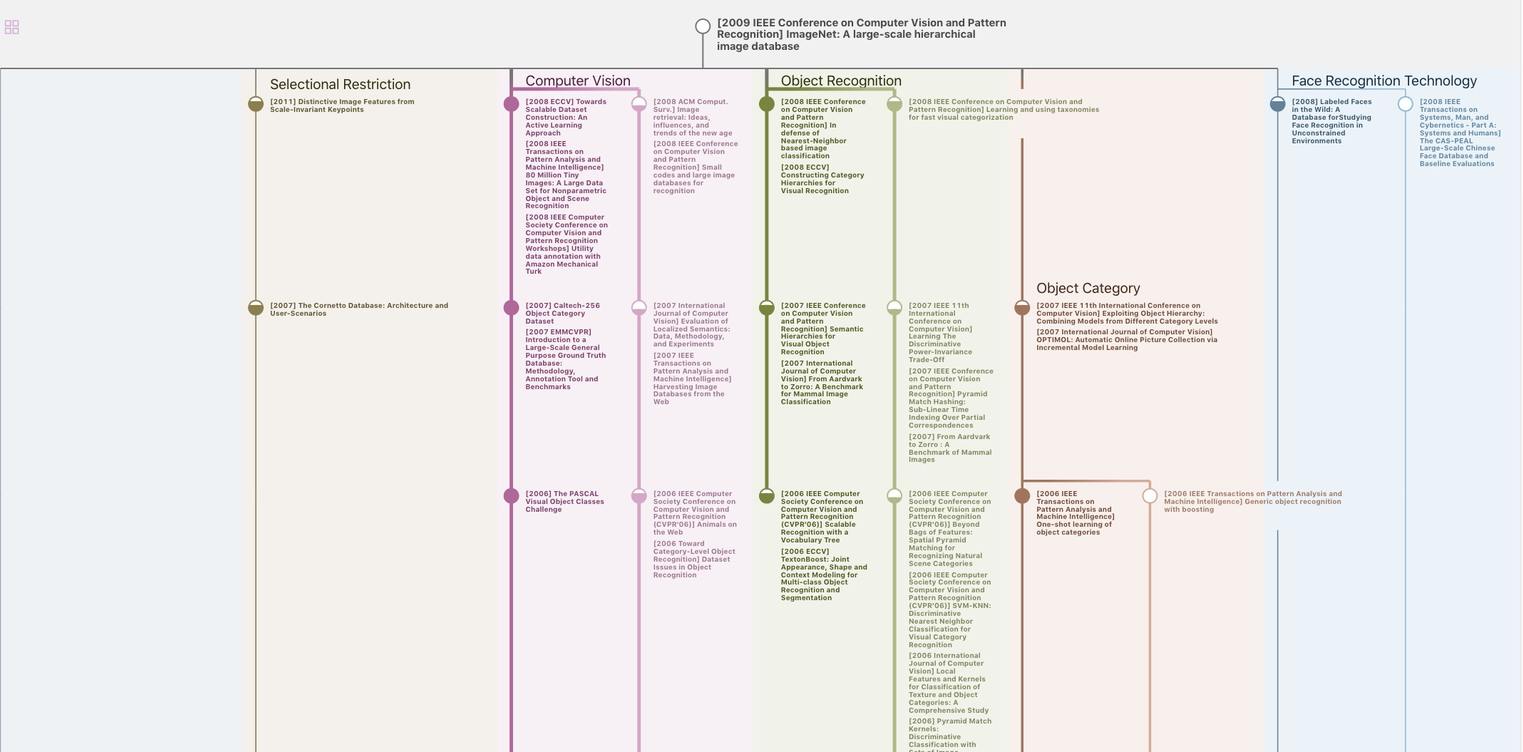
生成溯源树,研究论文发展脉络
Chat Paper
正在生成论文摘要