Pair of projections based on sparse consistence with applications to efficient face recognition.
Sig. Proc.: Image Comm.(2017)
摘要
Dimension reduction based feature extraction and classification method show significant performance on the high-dimensional face images. The traditional dimension reduction methods learn a projection based on the Fisher criterion or local structure of the face images. This work aims at learning a pair of projection based on sparse consistence which is measured by sparse constraint and label information for efficient face recognition. The first projection maps the original high-dimensional face images into a low-dimensional space where each face is sparse, and the second one which can also be treated as a classifier guides the sparse low-dimensional face images to the right label. The pair of projections is optimized together using alternative update rules efficiently. Due to the discriminant power of sparse face images and the supervised classifier, the proposed algorithm integrates the supervised and unsupervised information and is more efficient than them for face recognition on both learning and classifying. Experimental results on the challenging Extended Yale B, AR, and LFW face image databases demonstrate the proposed algorithm on both accuracy and efficiency. HighlightsAn integrate projection learning based on sparse consistence for FR is proposed.Unsupervised/supervised information are introduced into PPL to extract the discriminant feature.Analysis of complexity and convergence guarantee the efficiency of FR.Experiments on the challenging face datasets validate the accuracy and efficiency of PPL.
更多查看译文
关键词
Pace recognition,Dimension reduction,Pair of projections,Sparse consistence
AI 理解论文
溯源树
样例
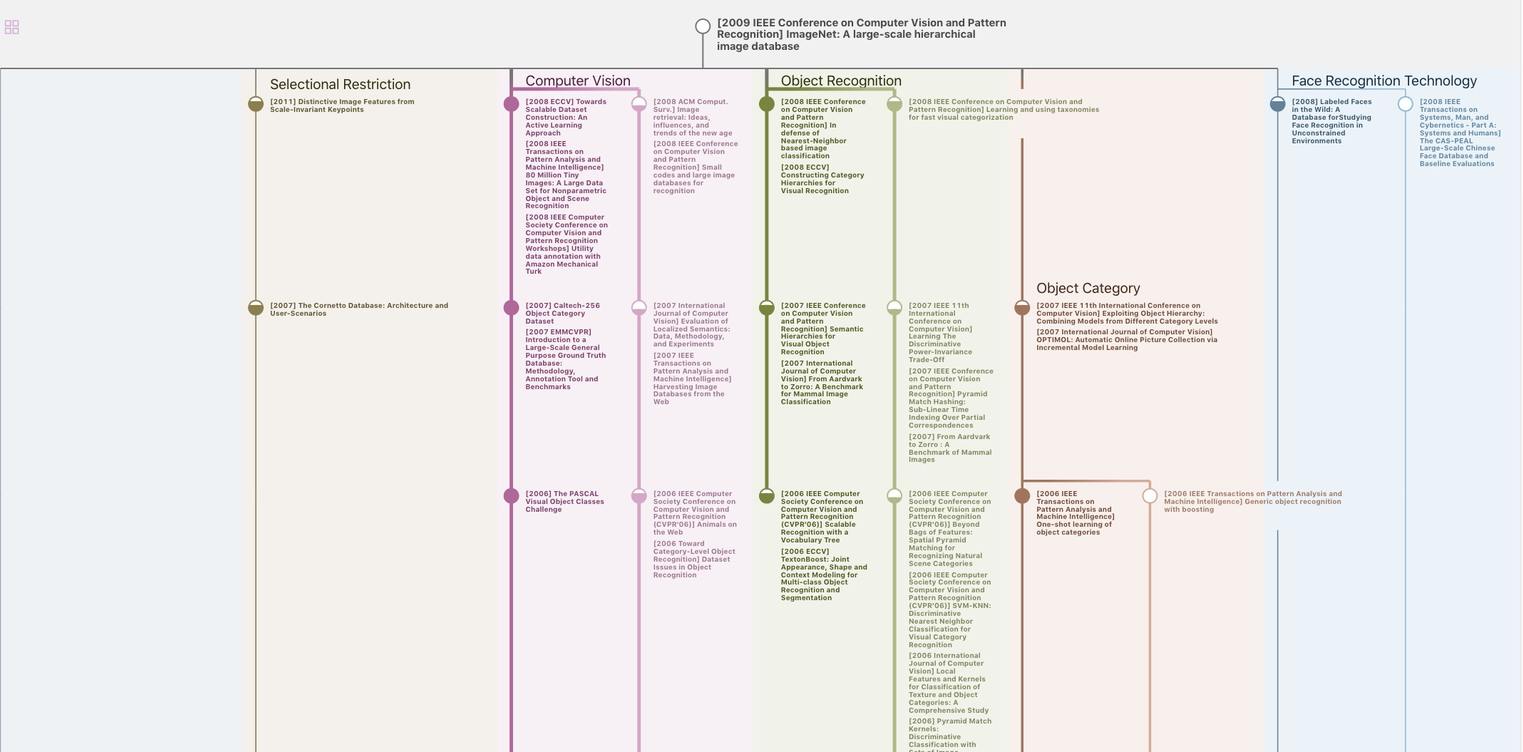
生成溯源树,研究论文发展脉络
Chat Paper
正在生成论文摘要